Open Access
ARTICLE
DuFNet: Dual Flow Network of Real-Time Semantic Segmentation for Unmanned Driving Application of Internet of Things
1 School of Computer Science and Engineering, Nanjing University of Science and Technology, Nanjing, 210094, China
2 School of Cyberspace Security, Nanjing University of Science and Technology, Wuxi, 320200, China
* Corresponding Author: Zhichao Lian. Email:
(This article belongs to the Special Issue: Artificial Intelligence for Mobile Edge Computing in IoT)
Computer Modeling in Engineering & Sciences 2023, 136(1), 223-239. https://doi.org/10.32604/cmes.2023.024742
Received 07 June 2022; Accepted 14 September 2022; Issue published 05 January 2023
Abstract
The application of unmanned driving in the Internet of Things is one of the concrete manifestations of the application of artificial intelligence technology. Image semantic segmentation can help the unmanned driving system by achieving road accessibility analysis. Semantic segmentation is also a challenging technology for image understanding and scene parsing. We focused on the challenging task of real-time semantic segmentation in this paper. In this paper, we proposed a novel fast architecture for real-time semantic segmentation named DuFNet. Starting from the existing work of Bilateral Segmentation Network (BiSeNet), DuFNet proposes a novel Semantic Information Flow (SIF) structure for context information and a novel Fringe Information Flow (FIF) structure for spatial information. We also proposed two kinds of SIF with cascaded and paralleled structures, respectively. The SIF encodes the input stage by stage in the ResNet18 backbone and provides context information for the feature fusion module. Features from previous stages usually contain rich low-level details but high-level semantics for later stages. The multiple convolutions embed in Parallel SIF aggregate the corresponding features among different stages and generate a powerful global context representation with less computational cost. The FIF consists of a pooling layer and an upsampling operator followed by projection convolution layer. The concise component provides more spatial details for the network. Compared with BiSeNet, our work achieved faster speed and comparable performance with 72.34% mIoU accuracy and 78 FPS on Cityscapes Dataset based on the ResNet18 backbone.Graphic Abstract
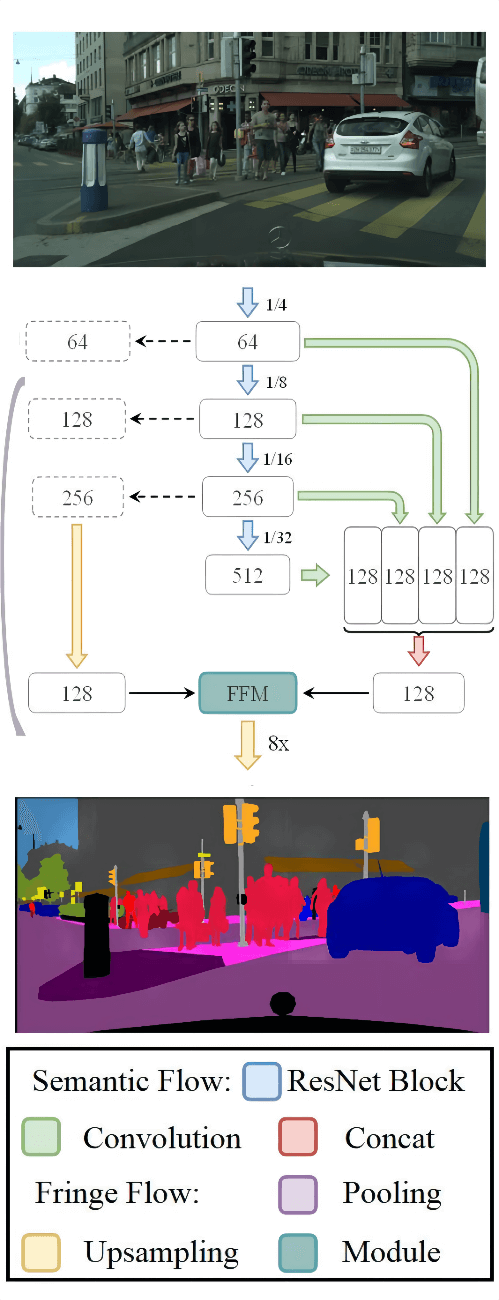
Keywords
Cite This Article
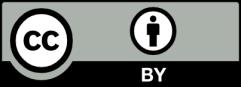
This work is licensed under a Creative Commons Attribution 4.0 International License , which permits unrestricted use, distribution, and reproduction in any medium, provided the original work is properly cited.