Open Access
ARTICLE
A Convolutional Autoencoder Based Fault Detection Method for Metro Railway Turnout
1 School of Electronic and Information Engineering, Tongji University, Shanghai, 201804, China
2 School of Engineering, City University of Hong Kong, Hong Kong, 200433, China
3 School of Civil Aviation, Northwestern Polytechnical University, Xi’an, 710072, China
4 School of Computer Engineering, Jimei University, Xiamen, 361021, China
* Corresponding Author: Xingqiu Li. Email:
(This article belongs to the Special Issue: AI and Machine Learning Modeling in Civil and Building Engineering)
Computer Modeling in Engineering & Sciences 2023, 136(1), 471-485. https://doi.org/10.32604/cmes.2023.024033
Received 22 May 2022; Accepted 15 September 2022; Issue published 05 January 2023
Abstract
Railway turnout is one of the critical equipment of Switch & Crossing (S&C) Systems in railway, related to the train’s safety and operation efficiency. With the advancement of intelligent sensors, data-driven fault detection technology for railway turnout has become an important research topic. However, little research in the literature has investigated the capability of data-driven fault detection technology for metro railway turnout. This paper presents a convolutional autoencoder-based fault detection method for the metro railway turnout considering human field inspection scenarios. First, the one-dimensional original time-series signal is converted into a two-dimensional image by data pre-processing and 2D representation. Next, a binary classification model based on the convolutional autoencoder is developed to implement fault detection. The profile and structure information can be captured by processing data as images. The performance of our method is evaluated and tested on real-world operational current data in the metro stations. Experimental results show that the proposed method achieves better performance, especially in terms of error rate and specificity, and is robust in practical engineering applications.Keywords
Cite This Article
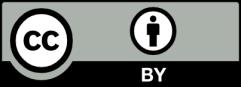
This work is licensed under a Creative Commons Attribution 4.0 International License , which permits unrestricted use, distribution, and reproduction in any medium, provided the original work is properly cited.