Open Access
ARTICLE
MDNN: Predicting Student Engagement via Gaze Direction and Facial Expression in Collaborative Learning
1 School of Computer Science, Central China Normal University, Wuhan, 430079, China
2 Computer Science, School of Science, Rensselaer Polytechnic Institute, Troy, 12180, USA
3 National Engineering Laboratory of Educational Big Data Application Technology, Central China Normal University, Wuhan, 430079, China
* Corresponding Author: Yi Chen. Email:
(This article belongs to the Special Issue: Humanized Computing and Reasoning in Teaching and Learning)
Computer Modeling in Engineering & Sciences 2023, 136(1), 381-401. https://doi.org/10.32604/cmes.2023.023234
Received 15 April 2022; Accepted 05 September 2022; Issue published 05 January 2023
Abstract
Prediction of students’ engagement in a Collaborative Learning setting is essential to improve the quality of learning. Collaborative learning is a strategy of learning through groups or teams. When cooperative learning behavior occurs, each student in the group should participate in teaching activities. Researchers showed that students who are actively involved in a class gain more. Gaze behavior and facial expression are important nonverbal indicators to reveal engagement in collaborative learning environments. Previous studies require the wearing of sensor devices or eye tracker devices, which have cost barriers and technical interference for daily teaching practice. In this paper, student engagement is automatically analyzed based on computer vision. We tackle the problem of engagement in collaborative learning using a multi-modal deep neural network (MDNN). We combined facial expression and gaze direction as two individual components of MDNN to predict engagement levels in collaborative learning environments. Our multi-modal solution was evaluated in a real collaborative environment. The results show that the model can accurately predict students’ performance in the collaborative learning environment.Keywords
Cite This Article
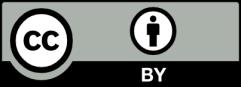
This work is licensed under a Creative Commons Attribution 4.0 International License , which permits unrestricted use, distribution, and reproduction in any medium, provided the original work is properly cited.