Open Access
ARTICLE
Vessels Segmentation in Angiograms Using Convolutional Neural Network: A Deep Learning Based Approach
1 School of Computer Science and Engineering, Vellore Institute of Technology, Vellore, 632014, India
2 Department of Computer Science and Information Engineering, Asia University, Taichung, 41354, Taiwan
3 Department of Medical Research, China Medical University Hospital, China Medical University, Taichung, 406, Taiwan
4 Guangdong-Hong Kong-Macao Joint Laboratory for Intelligent Micro-Nano Optoelectronic Technology, School of Mathematics and Big Data, Foshan University, Foshan, 528000, China
5 Medica Superspecialty Hospital, Kolkata, 700099, India
6 Automation and Applied Informatics, Aurel Vlaicu University of Arad, Arad, 310130, Romania
* Corresponding Author: Ching-Hsien Hsu. Email:
(This article belongs to the Special Issue: Bio-inspired Computer Modelling: Theories and Applications in Engineering and Sciences)
Computer Modeling in Engineering & Sciences 2023, 136(1), 241-255. https://doi.org/10.32604/cmes.2023.019644
Received 05 October 2021; Accepted 23 August 2022; Issue published 05 January 2023
Abstract
Coronary artery disease (CAD) has become a significant cause of heart attack, especially among those 40 years old or younger. There is a need to develop new technologies and methods to deal with this disease. Many researchers have proposed image processing-based solutions for CAD diagnosis, but achieving highly accurate results for angiogram segmentation is still a challenge. Several different types of angiograms are adopted for CAD diagnosis. This paper proposes an approach for image segmentation using Convolution Neural Networks (CNN) for diagnosing coronary artery disease to achieve state-of-the-art results. We have collected the 2D X-ray images from the hospital, and the proposed model has been applied to them. Image augmentation has been performed in this research as it’s the most significant task required to be initiated to increase the dataset’s size. Also, the images have been enhanced using noise removal techniques before being fed to the CNN model for segmentation to achieve high accuracy. As the output, different settings of the network architecture undoubtedly have achieved different accuracy, among which the highest accuracy of the model is 97.61%. Compared with the other models, these results have proven to be superior to this proposed method in achieving state-of-the-art results.Keywords
Cite This Article
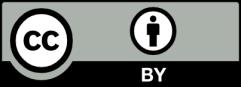
This work is licensed under a Creative Commons Attribution 4.0 International License , which permits unrestricted use, distribution, and reproduction in any medium, provided the original work is properly cited.