Open Access
ARTICLE
Performance Analysis of RIS Assisted NOMA Networks over Rician Fading Channels
1 School of Electronic and Information Engineering, Beihang University, Beijing, 100191, China
2 College of Information Science and Electronic Engineering, Zhejiang University, Hangzhou, 310027, China
3 International Joint Innovation Center, Zhejiang University, Haining, 314400, China
4 Zhejiang Provincial Key Laboratory of Information Processing, Communication and Networking (IPCAN), Hangzhou, 310027, China
5 School of Information and Communication Engineering, Beijing Information Science and Technology University, Beijing, 100101, China
6 Zhejiang Laboratory, Hangzhou, 311121, China
* Corresponding Author: Feng Liu. Email:
(This article belongs to the Special Issue: Recent Advances in Backscatter and Intelligent Reflecting Surface Communications for 6G-enabled Internet of Things Networks)
Computer Modeling in Engineering & Sciences 2023, 135(3), 2531-2555. https://doi.org/10.32604/cmes.2023.024940
Received 17 June 2022; Accepted 15 July 2022; Issue published 23 November 2022
Abstract
In this paper, we consider a downlink non-orthogonal multiple access (NOMA) network assisted by two reconfigurable intelligent surfaces (RISs) over Rician fading channels, in which each user communicates with the base station by the virtue of a RIS to enhance the reliability of the received signal. To evaluate the system performance of our proposed RIS-NOMA network, we first derive the exact and asymptotic expressions for the outage probability and ergodic rate of two users. Then, we derive the exact and asymptotic upper bound expressions for the ergodic rate of the nearby user. Based on asymptotic analytical results, the diversity orders for the outage probability and the high signal-to-noise ratio (SNR) slopes for the ergodic rate of the two users are obtained in the high SNR regime. Moreover, we derive the system throughputs of the proposed RIS-NOMA network in delay-limited and delay-tolerant transmission modes. Numerical results confirm our analysis and demonstrate that: 1) The outage probability and ergodic rate of RIS-NOMA networks are superior to that of RIS-assisted orthogonal multiple access (OMA) networks; 2) The RIS-NOMA networks have ability to achieve a larger system throughput compared to RIS-OMA networks; and 3) The system performance of RIS-NOMA networks can be significantly improved as the number of reflecting elements and Rician factor increases.Keywords
As a promising multiple access technology in the next generation wireless communication network, non-orthogonal multiple access (NOMA) has the ability to tremendously enhance the spectral and energy efficiency [1,2]. The distinguishing feature of NOMA is that multiple users with different quality-of-service requirements are served by exploiting the superposition coding scheme in the same time/frequency/code resource block [3–5]. In [6,7], the authors have proved that the outage probability and ergodic rate of NOMA can be better than that of conventional orthogonal multiple access (OMA). Zhu et al. [8] have proposed an optimal power allocation scheme learned by adopting the deep deterministic policy gradient algorithm in multiple-input multiple-output NOMA vehicular edge computing.
Recently, reconfigurable intelligent surface (RIS) has been regarded as a promising technology for the next generation wireless communication, which can improve the spectral and energy efficiency of future wireless communication networks in a cost-effective way [9–11]. Specifically, RIS is a low-cost planar array composed of a large number of reconfigurable passive elements, in which the amplitude and phase of the incident signal for each element can be changed independently [12,13]. By adjusting the amplitude and phase coefficients of RIS elements through programmable controller, the electromagnetic signal can be reconfigured to significantly enhance the signal quality and the coverage of wireless communication [14]. Compared with the conventional relaying technology, the advantage of RIS is that the passive reflection does not consume energy and it does not generate self-interference in full duplex (FD) mode [15]. The RIS-empowered multiuser uplink communication system has been investigated in [16], in which a channel estimation framework based on the parallel factor decomposition has been proposed to unfold the resulting cascaded channel model. In [17], the authors have studied the downlink wireless communication system, in which the transmit beamforming and the RIS have been jointly optimized to maximize the energy efficiency of the system. A new joint design of the transmit beamforming and the phase shifting of the RIS has been proposed in [18], in which the sum rate of the multiuser system has been maximized by using deep reinforcement learning (DRL). In [19], the multiuser communication system assisted by RIS has been considered, in which the power allocation and the phase shifting of the RIS have been jointly optimized to minimize the sum transmit power. Considering RIS-empowered terahertz band communications, a DRL-based hybrid beamforming scheme has been proposed in [20] to improve the communication coverage.
Since both RIS and NOMA can greatly improve the spectrum and energy efficiency, it is critical to study their combined advantages to further enhance the spectrum and energy efficiency of future wireless communication networks. In [21], a simple RIS-NOMA transmission scheme has been designed, in which the outage performance of the cell-edge user has been improved with the assistance of RIS in a NOMA system. In [22], the authors have investigated the performance of RIS-NOMA networks in terms of the outage probability, ergodic rate, and energy efficiency by exploiting 1-bit coding scheme. The effects of coherent phase shifting and random phase shifting on outage performance for RIS-NOMA networks have been investigated in [23]. By designing the passive beamforming weights, the authors in [24] have investigated the outage probability and ergodic rate of prioritized user for RIS-NOMA. The RIS reflection with discrete phase shifting for RIS-NOMA and RIS-OMA has been analyzed in [25]. In [26], a novel NOMA solution with RIS partitioning has been proposed to reduce the transmission interference. Considering the impact of the direct link from the base station (BS) to users on the network performance, the outage behaviors of multiple RISs-assisted NOMA networks have been studied by using discrete phase shifting in [27]. The authors in [28] have examined the outage performance of RIS-NOMA networks under presence of hardware impairment. In [29], the outage probability and ergodic rate of RIS-aided uplink and downlink NOMA networks have been investigated by employing continuous phase shifting. The authors in [30] have studied RIS assisted downlink NOMA networks, in which the performance of the networks has been analyzed by using the coherent phase shifting.
1.2 Motivation and Contributions
As mentioned in the above works, compared with the conventional relaying technology, the RIS is capable of reflecting the incident signal transmitted from the transmitter to the receiver in FD mode without self-interference and energy consumption, which not only enhances the spectrum and energy efficiency, but also has a very low cost. Therefore, the integration of RIS and NOMA can further improve the spectrum and energy efficiency of the next generation wireless communication networks. Furthermore, the current literature on RIS-NOMA mainly concentrates on the optimization problem, but the research on performance analysis is not enough. Compared with conventional NOMA systems, the performance analysis of RIS-NOMA systems is more complex and challenging owing to the influence of the RIS. These motivate us to study the performance of RIS assisted NOMA systems, which provides an important theoretical basis for deployment of RIS in the future.
In this paper, we consider a RIS assisted NOMA network, in which a pair of NOMA users in a cell cannot directly receive signals from the BS due to the obstruction of obstacles, and each user needs the help of a RIS. Moreover, we comprehensively analyze the performance of RIS-NOMA networks in terms of the outage probability, ergodic rate, and system throughput. The primary contributions of this paper are summarized as follows:
• We derive the closed-form and asymptotic expressions for the outage probability of a pair of NOMA users over Rician fading channels for the proposed RIS-assisted NOMA network. To gain further insight, we obtain the diversity orders of the pair of NOMA users for RIS-NOMA network according to the asymptotic analyses at high signal-to-noise ratio (SNR). We observe that the diversity orders of the pair of NOMA users depend on the number of reflecting elements and Rician factor.
• We compare the outage performance of the pair of users for RIS-NOMA and RIS-OMA networks. We demonstrate that the outage performance of RIS-NOMA outperforms that of RIS-OMA. We prove that the RIS-NOMA network is capable of improving outage performance with increasing the number of reflecting elements and Rician factor.
• We derive the exact and asymptotic expressions for the ergodic rate of the pair of NOMA users over Rician fading channels for our proposed RIS-NOMA network. To approximate the exact ergodic rate, we also derive the exact and asymptotic upper bound expressions for the ergodic rate of the nearby user. Based on theoretical analyses, we obtain the high SNR slopes for the ergodic rate of the pair of NOMA users. We observe that the throughput ceiling appears in the ergodic rate of the distant user at high SNR. We demonstrate that the superiority of RIS-NOMA over RIS-OMA in terms of the ergodic rate. We evidence that the ergodic rate of RIS-NOMA becomes larger with the increase of the number of reflecting elements.
• We analyze the system throughput of our proposed RIS-NOMA network in two transmission modes. In delay-limited transmission mode, we observe that the system throughput of RIS-NOMA converges to a constant at high SNR. Furthermore, we prove that the proposed RIS-NOMA is capable of enhancing the system throughput compared to RIS-OMA. In delay-tolerant transmission mode, we evidence that the proposed RIS-NOMA can achieve a larger system throughput than RIS-OMA at high SNR. Moreover, we confirm that as the number of reflecting elements increases, the system throughput of RIS-NOMA can be significantly improved in the two transmission modes.
The main notations used in this paper are explained as follows.
Consider a two-RIS assisted NOMA network with one single-antenna BS and two single-antenna users as shown in Fig. 1, in which the BS sends the superposed signals to the pair of NOMA users by means of two RISs. Assume that there is no direct link between the BS and each NOMA user due to strong attenuation, and each NOMA user needs a RIS to assist in communicating with the BS.
Figure 1: System model of RIS assisted downlink NOMA networks
It is assumed that the channel state information of all channels is completely known at the BS. The complex channel coefficients from the BS to
and
where the channel coefficients from the BS to the k-th reflecting element of
and
Defining
Based on the superposition coding scheme, the BS broadcasts superposed signal
and
where
where
The signal of
For comparison, we regard the RIS-OMA scheme as the benchmark, in which the BS sends information to two OMA users through two RISs in two time slots. In each time slot, signal
The received SINR at
In order to achieve optimal performance, we use the coherent phase shift design. For the wireless communication links of RIS-NOMA networks, our goal is to optimize the channel by adjusting the amplitude and phase of the RIS to achieve the optimal channel gain for each user. For the BS-RIS-
where
The channel coefficients
where
and
where
In communication systems, the outage probability can be defined as the probability that the outage occurs for a user when the information rate of the user is lower than its target rate. The outage probability is a widely used system performance metric in fixed-rate transmission systems. In this section, the outage performance of downlink RIS-NOMA networks is investigated.
By using the SIC scheme at nearby user
where
Theorem 3.1. Under Rician fading channels, the closed-form expression for the outage probability of
where
Proof: See Appendix A.
Remark 3.1. From Eq. (17), it is observed that the approximate outage probability of
On account of the NOMA principle, the signal of
The outage probability of
Theorem 3.2. Under Rician fading channels, the closed-form expression for the outage probability of
where
Proof: See Appendix B.
Remark 3.2. From Eq. (19), it can be seen that the approximate outage probability of
3.3 Outage Probability of
For RIS-OMA networks,
where
Corollary 3.1. Under Rician fading channels, the closed-form expression for the outage probability of
where
In communication systems, the diversity order can be defined as the slope of the outage probability at high SNR. The diversity order describes the speed at which the outage probability decreases with the transmit SNR, which is usually used to evaluate the outage performance of wireless communication networks. The diversity order can be expressed as
In order to obtain further insights, the approximate results of the outage probability are analyzed at high SNR, which can be used to determine the diversity order. The asymptotic outage probabilities of
Corollary 3.2. Based on Eqs. (17) and (19), when
and
Proof: Applying the series representation [32], when
and
Substituting Eqs. (25) and (26) into Eqs. (17) and (19), respectively, Eqs. (23) and (24) can be obtained. The proof is completed.
Remark 3.3. From Eqs. (23) and (24), it can be observed that the asymptotic outage probabilities of
Substituting Eqs. (23) and (24) into Eq. (22), the diversity orders of
and
Remark 3.4. From Eqs. (27) and (28), we can observe that the diversity orders of
Remark 3.5. It can be observed that the diversity orders increase as K,
Remark 3.6. Similarly, the diversity order of
3.5 Delay-Limited System Throughput
The system throughput is an important performance metric in wireless communication systems. The research on system throughput is of great significance for practical implementation. In the delay-limited transmission mode, the information is transmitted from the BS to users at a constant rate, which leads to outage due to the influence of the wireless fading channels. Therefore, the system throughput depends on the outage probability in the delay-limited transmission mode. The delay-limited system throughput of RIS-NOMA is expressed as
where
The ergodic rate is a commonly used system performance metric in adaptive-rate transmission systems. In this section, the ergodic rates of two users for RIS-NOMA networks are analyzed.
Based on SIC principle, if
The ergodic rate of
Theorem 4.1. Under Rician fading channels, the exact expression for the ergodic rate of
where
Proof: See Appendix C.
Remark 4.1. From Eq. (31), we can observe that the ergodic rate of
Assuming that
The ergodic rate of
Theorem 4.2. Under Rician fading channels, the approximate expression for the ergodic rate of
where
Proof: See Appendix D.
Remark 4.2. From Eq. (33), it is observed that the ergodic rate of
4.3 Ergodic Rate of
For RIS-OMA networks, since
Similar to the derivation process of Eq. (31), the ergodic rate of
Corollary 4.1. Under Rician fading channels, the exact expression for the ergodic rate of
Similar to the diversity order, the high SNR slope can be defined as the slope of the ergodic rate at high SNR, which describes how fast the ergodic rate increases with the transmit SNR. The high SNR slope can be expressed as
To gain deep insights into the system performance, the approximate ergodic rate at high SNR is studied, which can be used to calculate the high SNR slope. However, the approximate ergodic rate of
Theorem 4.3. Under Rician fading channels, the upper bound for the ergodic rate of
Proof: Based on Eq. (30), by adopting the Jensen’s inequality, the upper bound for the ergodic rate of
On the basis of Eq. (12), the upper bound for the ergodic rate of
According to Eqs. (14) and (15), the upper bound for the ergodic rate of
Hence Eq. (37) can be obtained. The proof is completed.
Remark 4.3. From Eq. (37), we can observe that the upper bound for the ergodic rate of
The asymptotic upper bound for the ergodic rate of
Corollary 4.2. Based on Eq. (37), when
Remark 4.4. From Eq. (41), we can observe that the asymptotic upper bound for the ergodic rate of
Substituting Eq. (41) into Eq. (36), the high SNR slope of
Remark 4.5. From Eq. (42), we can observe that the high SNR slope of
The asymptotic ergodic rate of
Corollary 4.3. Based on Eq. (32), when
Proof: With the aid of Eqs. (9) and (12), we can obtain
In view of Eq. (32), when
Hence Eq. (43) can be obtained. The proof is completed.
Remark 4.6. From Eq. (43), it can be observed that the asymptotic ergodic rate of
Substituting Eq. (43) into Eq. (36), the high SNR slope of
Remark 4.7. From Eq. (46), it can be observed that the high SNR slope of
Similar to the derivation process of Eq. (37), the upper bound for the ergodic rate of
Corollary 4.4. Under Rician fading channels, the upper bound for the ergodic rate of
The asymptotic upper bound for the ergodic rate of
Corollary 4.5. Based on Eq. (47), when
Remark 4.8. Substituting Eq. (48) into Eq. (36), the high SNR slope of
4.5 Delay-Tolerant System Throughput
In the delay-tolerant transmission mode, the information is transmitted from the BS to users at any constant rate, which is subject to the channel conditions of the users. Hence the delay-tolerant system throughput of RIS-NOMA is expressed as
where
In this section, numerical results are presented to evaluate the performance of RIS-NOMA networks over Rician fading channels. The effects of the Rician factor, the number of reflecting elements of the RIS, and the pass loss exponent on the performance of RIS-NOMA networks are discussed. The accuracy of the derived theoretical results can be verified by Monte Carlo simulations. In order to compare the performance with the RIS-NOMA networks, the conventional RIS-OMA networks are also provided. Without loss of generality, the power allocation factors of two users are set to
Fig. 2 plots the outage probabilities of two users vs. the transmit SNR for RIS-NOMA networks. The parameters are set to
Figure 2: Outage probability vs. the transmit SNR when K = 8, κ = −10 dB, and α = 2
Fig. 3 plots the outage probabilities of two users vs. the transmit SNR with different K for RIS-NOMA networks. The parameters are set to
Figure 3: Outage probability vs. the transmit SNR with different K when κ = −10 dB and α = 2
In order to explain the influence of the Rician factor on the outage performance of RIS-NOMA networks, Fig. 4 plots the outage probabilities of two users vs. the transmit SNR with different
Figure 4: Outage probability vs. the transmit SNR with different κ when K = 8 and α = 2
To further illustrate the impact of the path loss exponent on the outage performance of RIS-NOMA networks, Fig. 5 plots the outage probabilities of two users vs. the transmit SNR with different
Figure 5: Outage probability vs. the transmit SNR with different α when K = 8 and κ = −10 dB
Fig. 6 plots the ergodic rates of two users vs. the transmit SNR for RIS-NOMA networks. The parameters are set to
Figure 6: Ergodic rate vs. the transmit SNR when K = 20, κ = −10 dB, and α = 2
Considering the influence of the number of reflecting elements on the ergodic performance of RIS-NOMA networks, Fig. 7 plots the ergodic rates of two users vs. the transmit SNR with different K for RIS-NOMA networks. The parameters are set to
Figure 7: Ergodic rate vs. the transmit SNR with different K when κ = −10 dB and α = 2
Fig. 8 plots the system throughput vs. the transmit SNR with different K for RIS-NOMA networks in delay-limited transmission mode. The parameters are set to
Figure 8: System throughput in delay-limited transmission mode vs. the transmit SNR with different K when κ = −10 dB and α = 2
As a further advance, Fig. 9 plots the system throughput vs. the transmit SNR with different K for RIS-NOMA networks in delay-tolerant transmission mode. The parameters are set to
Figure 9: System throughput in delay-tolerant transmission mode vs. the transmit SNR with different K when κ = −10 dB and α = 2
In this paper, the system performance of RIS-assisted downlink NOMA communication networks has been investigated in terms of the outage probability, ergodic rate, and system throughput over Rician fading channels. The exact and asymptotic expressions for the outage probability and ergodic rate of two users for RIS-NOMA networks have been derived. Based on the theoretical analyses, the diversity orders and high SNR slopes of the two users have been obtained at high SNR. In addition, the system throughputs of RIS-NOMA networks have been derived in delay-limited and delay-tolerant transmission modes. Simulation results have indicated that the outage probability and ergodic rate of RIS-NOMA outperform that of RIS-OMA. It has been shown that the system throughput of RIS-NOMA is superior to that of RIS-OMA. Moreover, it has been demonstrated that the system performance of RIS-NOMA networks becomes better with increasing the number of reflecting elements and Rician factor. Based on this work, we will consider extending the research to multiple users in the future. In addition, optimized power allocation and multiple antennas of device nodes are also promising research directions.
Funding Statement: This work was supported in part by the Key R&D Program of Zhejiang Province under Grant No. 2020C05005, in part by the National Natural Science Foundation of China under Grants U2033215, 91738301, 91538202, and 91638301, in part by the Program for New Century Excellent Talents in University under Grant NCET-09-0025, and in part by the Fundamental Research Funds for the Central Universities. The work of C. Huang was partially supported by the China National Key R&D Program under Grant 2021YFA1000500, National Natural Science Foundation of China under Grant 62101492, Zhejiang Provincial Natural Science Foundation of China under Grant LR22F010002, Distinguished Young Scholars of the National Natural Science Foundation of China, Ng Teng Fong Charitable Foundation in the form of ZJU-SUTD IDEA Grant, Zhejiang University Education Foundation Qizhen Scholar Foundation, and Fundamental Research Funds for the Central Universities under Grant 2021FZZX001-21. The work of X. Yue was supported by the National Natural Science Foundation of China under Grant 62071052 and the R&D Program of Beijing Municipal Education Commission under Grant KM202011232003.
Conflicts of Interest: The authors declare that they have no conflicts of interest to report regarding the present study.
References
1. Ding, Z., Liu, Y., Choi, J., Sun, Q., Elkashlan, M. et al. (2017). Application of non-orthogonal multiple access in LTE and 5G networks. IEEE Communications Magazine, 55(2), 185–191. DOI 10.1109/MCOM.35. [Google Scholar] [CrossRef]
2. Shirvanimoghaddam, M., Dohler, M., Johnson, S. J. (2017). Massive non-orthogonal multiple access for cellular IoT: Potentials and limitations. IEEE Communications Magazine, 55(9), 55–61. DOI 10.1109/MCOM.2017.1600618. [Google Scholar] [CrossRef]
3. Liu, Y., Qin, Z., Elkashlan, M., Ding, Z., Nallanathan, A. et al. (2017). Non-orthogonal multiple access for 5G and beyond. Proceedings of the IEEE, 105(12), 2347–2381. DOI 10.1109/JPROC.2017.2768666. [Google Scholar] [CrossRef]
4. Islam, S. M. R., Avazov, N., Dobre, O. A., Kwak, K. (2017). Power-domain non-orthogonal multiple access (NOMA) in 5G systems: Potentials and challenges. IEEE Communications Surveys and Tutorials, 19(2), 721–742. DOI 10.1109/COMST.2016.2621116. [Google Scholar] [CrossRef]
5. Montalban, J., Scopelliti, P., Fadda, M., Iradier, E., Desogus, C. et al. (2018). Multimedia multicast services in 5G networks: Subgrouping and non-orthogonal multiple access techniques. IEEE Communications Magazine, 56(3), 91–95. DOI 10.1109/MCOM.2018.1700660. [Google Scholar] [CrossRef]
6. Ding, Z., Yang, Z., Fan, P., Poor, H. V. (2014). On the performance of non-orthogonal multiple access in 5G systems with randomly deployed users. IEEE Signal Processing Letters, 21(12), 1501–1505. DOI 10.1109/LSP.97. [Google Scholar] [CrossRef]
7. Yue, X., Qin, Z., Liu, Y., Kang, S., Chen, Y. (2018). A unified framework for non-orthogonal multiple access. IEEE Transactions on Communications, 66(11), 5346–5359. DOI 10.1109/TCOMM.2018.2842217. [Google Scholar] [CrossRef]
8. Zhu, H., Wu, Q., Wu, X. J., Fan, Q., Fan, P. et al. (2022). Decentralized power allocation for MIMO-NOMA vehicular edge computing based on deep reinforcement learning. IEEE Internet of Things Journal, 9(14), 12770–12782. DOI 10.1109/JIOT.2021.3138434. [Google Scholar] [CrossRef]
9. Liu, Y., Liu, X., Mu, X., Hou, T., Xu, J. et al. (2021). Reconfigurable intelligent surfaces: Principles and opportunities. IEEE Communications Surveys and Tutorials, 23(3), 1546–1577. DOI 10.1109/COMST.2021.3077737. [Google Scholar] [CrossRef]
10. Zhang, Z., Xiao, Y., Ma, Z., Xiao, M., Ding, Z. et al. (2019). 6G wireless networks: Vision, requirements, architecture, and key technologies. IEEE Vehicular Technology Magazine, 14(3), 28–41. DOI 10.1109/MVT.10209. [Google Scholar] [CrossRef]
11. Pan, C., Ren, H., Wang, K., Kolb, J. F., Elkashlan, M. et al. (2021). Reconfigurable intelligent surfaces for 6G systems: Principles, applications, and research directions. IEEE Communications Magazine, 59(6), 14–20. DOI 10.1109/MCOM.001.2001076. [Google Scholar] [CrossRef]
12. Huang, C., Zappone, A., Alexandropoulos, G. C., Debbah, M., Yuen, C. (2019). Reconfigurable intelligent surfaces for energy efficiency in wireless communication. IEEE Transactions on Wireless Communications, 18(8), 4157–4170. DOI 10.1109/TWC.7693. [Google Scholar] [CrossRef]
13. Huang, C., Hu, S., Alexandropoulos, G. C., Zappone, A., Yuen, C. et al. (2020). Holographic MIMO surfaces for 6G wireless networks: Opportunities, challenges, and trends. IEEE Wireless Communications, 27(5), 118–125. DOI 10.1109/MWC.7742. [Google Scholar] [CrossRef]
14. Zhou, G., Pan, C., Ren, H., Wang, K., Nallanathan, A. (2020). A framework of robust transmission design for IRS-aided MISO communications with imperfect cascaded channels. IEEE Transactions on Signal Processing, 68, 5092–5106. DOI 10.1109/TSP.2020.3019666. [Google Scholar] [CrossRef]
15. Wu, Q., Zhang, R. (2020). Towards smart and reconfigurable environment: Intelligent reflecting surface aided wireless network. IEEE Communications Magazine, 58(1), 106–112. DOI 10.1109/MCOM.35. [Google Scholar] [CrossRef]
16. Wei, L., Huang, C., Alexandropoulos, G. C., Yuen, C., Zhang, Z. et al. (2021). Channel estimation for RIS-empowered multi-user MISO wireless communications. IEEE Transactions on Communications, 69(6), 4144–4157. DOI 10.1109/TCOMM.2021.3063236. [Google Scholar] [CrossRef]
17. Yang, Z., Chen, M., Saad, W., Xu, W., Shikh-Bahaei, M. et al. (2022). Energy-efficient wireless communications with distributed reconfigurable intelligent surfaces. IEEE Transactions on Wireless Communications, 21(1), 665–679. DOI 10.1109/TWC.2021.3098632. [Google Scholar] [CrossRef]
18. Huang, C., Mo, R., Yuen, C. (2020). Reconfigurable intelligent surface assisted multiuser MISO systems exploiting deep reinforcement learning. IEEE Journal on Selected Areas in Communications, 38(8), 1839–1850. DOI 10.1109/JSAC.49. [Google Scholar] [CrossRef]
19. Yang, Z., Xu, W., Huang, C., Shi, J., Shikh-Bahaei, M. (2021). Beamforming design for multiuser transmission through reconfigurable intelligent surface. IEEE Transactions on Communications, 69(1), 589–601. DOI 10.1109/TCOMM.26. [Google Scholar] [CrossRef]
20. Huang, C., Yang, Z., Alexandropoulos, G. C., Xiong, K., Wei, L. et al. (2021). Multi-hop RIS-empowered terahertz communications: A DRL-based hybrid beamforming design. IEEE Journal on Selected Areas in Communications, 39(6), 1663–1677. DOI 10.1109/JSAC.2021.3071836. [Google Scholar] [CrossRef]
21. Ding, Z., Poor, H. V. (2020). A simple design of IRS-NOMA transmission. IEEE Communications Letters, 24(5), 1119–1123. DOI 10.1109/COML.4234. [Google Scholar] [CrossRef]
22. Yue, X., Liu, Y. (2022). Performance analysis of intelligent reflecting surface assisted NOMA networks. IEEE Transactions on Wireless Communications, 21(4), 2623–2636. DOI 10.1109/TWC.2021.3114221. [Google Scholar] [CrossRef]
23. Ding, Z., Schober, R., Poor, H. V. (2020). On the impact of phase shifting designs on IRS-NOMA. IEEE Wireless Communication Letters, 9(10), 1596–1600. DOI 10.1109/LWC.5962382. [Google Scholar] [CrossRef]
24. Hou, T., Liu, Y., Song, Z., Sun, X., Chen, Y. et al. (2020). Reconfigurable intelligent surface aided NOMA networks. IEEE Journal on Selected Areas in Communications, 38(11), 2575–2588. DOI 10.1109/JSAC.49. [Google Scholar] [CrossRef]
25. Zheng, B., Wu, Q., Zhang, R. (2020). Intelligent reflecting surface-assisted multiple access with user pairing: NOMA or OMA? IEEE Communications Letters, 24(4), 753–757. DOI 10.1109/COML.4234. [Google Scholar] [CrossRef]
26. Khaleel, A., Basar, E. (2022). A novel NOMA solution with RIS partitioning. IEEE Journal of Selected Topics in Signal Processing, 16(1), 70–81. DOI 10.1109/JSTSP.2021.3127725. [Google Scholar] [CrossRef]
27. Cheng, Y., Li, K. H., Liu, Y., Teh, K. C., Karagiannidis, G. K. (2021). Non-orthogonal multiple access (NOMA) with multiple intelligent reflecting surfaces. IEEE Transactions on Wireless Communications, 20(11), 7184–7195. DOI 10.1109/TWC.2021.3081423. [Google Scholar] [CrossRef]
28. Hemanth, A., Umamaheswari, K., Pogaku, A. C., Do, D. T., Lee, B. M. (2020). Outage performance analysis of reconfigurable intelligent surfaces-aided NOMA under presence of hardware impairment. IEEE Access, 8, 212156–212165. DOI 10.1109/Access.6287639. [Google Scholar] [CrossRef]
29. Cheng, Y., Li, K. H., Liu, Y., Teh, K. C., Vincent Poor, H. (2021). Downlink and uplink intelligent reflecting surface aided networks: NOMA and OMA. IEEE Transactions on Wireless Communications, 20(6), 3988–4000. DOI 10.1109/TWC.2021.3054841. [Google Scholar] [CrossRef]
30. Gong, X., Huang, C., Yue, X., Liu, F., Yang, Z. (2022). Performance analysis for reconfigurable intelligent surface assisted downlink NOMA networks. IET Communications, 16(13), 1593–1605. DOI 10.1049/cmu2.12375. [Google Scholar] [CrossRef]
31. Simon, M. K. (2006). Probability distributions involving gaussian random variables. USA: Springer. [Google Scholar]
32. Gradshteyn, I. S., Ryzhik, I. M. (2000). Table of integrals, series and products. 6th edition. New York, NY, USA: Academic Press. [Google Scholar]
33. Primak, S., Kontorovitch, V., Lyandres, V. (2004). Stochastic methods and their applications to communications: Stochastic differential equations approach. West Sussex, UK: Wiley. [Google Scholar]
34. Hildebrand, B. F. (1987). Introduction to numerical analysis. New York, NY, USA: Dover. [Google Scholar]
Appendix A: Proof of Theorem 1
Substituting Eqs. (7) and (8) into Eq. (16), based on Eq. (12), the outage probability of
where
Applying the series of Laguerre polynomials [33], the PDF of
where
Substituting Eq. (A.2) into Eq. (A.1), the outage probability of
Using
Applying the lower incomplete Gamma function [32], the outage probability of
where
Appendix B: Proof of Theorem 2
Substituting Eq. (9) into Eq. (18), the outage probability of
where the last equality holds because of Eq. (12).
Similarly, the PDF of
where
Substituting Eq. (B.2) into Eq. (B.1), using
By referring to the lower incomplete Gamma function, the outage probability of
where
Appendix C: Proof of Theorem 3
Substituting Eq. (8) into Eq. (30), according to Eq. (12), the ergodic rate of
The CDF of
Based on Eq. (17), the CDF of
Substituting Eq. (C.3) into Eq. (C.1), the ergodic rate of
Hence Eq. (31) can be obtained. The proof is completed.
Appendix D: Proof of Theorem 4
Substituting Eq. (9) into Eq. (32), in view of Eq. (12), the ergodic rate of
The CDF of
where
Substituting Eq. (D.3) into Eq. (D.1), the ergodic rate of
Using
Applying Chebyshev-Gauss quadrature [34], the ergodic rate of
where
Cite This Article
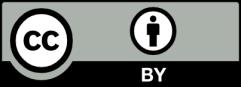
This work is licensed under a Creative Commons Attribution 4.0 International License , which permits unrestricted use, distribution, and reproduction in any medium, provided the original work is properly cited.