Open Access
ARTICLE
Remote Sensing Data Processing Process Scheduling Based on Reinforcement Learning in Cloud Environment
1 School of Computer and Information Engineering, Henan University, Kaifeng, 475004, China
2 Henan Province Engineering Research Center of Spatial Information Processing, Henan University, Kaifeng, 475004, China
3 School of Software, Henan University, Kaifeng, 475004, China
4 Department of Economics and Finance/Sustainable Real Estate Research Center, Hong Kong Shue Yan University, Hong Kong, 999077, China
5 Department of Computer Science and Engineering, European University Cyprus, Nicosia, 1516, Cyprus
6 CIICESI-ESTG, Politécnico do Porto, Felgueiras, 4610-156, Portugal
* Corresponding Author: Pu Cheng. Email:
Computer Modeling in Engineering & Sciences 2023, 135(3), 1965-1979. https://doi.org/10.32604/cmes.2023.024871
Received 10 June 2022; Accepted 20 July 2022; Issue published 23 November 2022
Abstract
Task scheduling plays a crucial role in cloud computing and is a key factor determining cloud computing performance. To solve the task scheduling problem for remote sensing data processing in cloud computing, this paper proposes a workflow task scheduling algorithm---Workflow Task Scheduling Algorithm based on Deep Reinforcement Learning (WDRL). The remote sensing data process modeling is transformed into a directed acyclic graph scheduling problem. Then, the algorithm is designed by establishing a Markov decision model and adopting a fitness calculation method. Finally, combine the advantages of reinforcement learning and deep neural networks to minimize make-time for remote sensing data processes from experience. The experiment is based on the development of CloudSim and Python and compares the change of completion time in the process of remote sensing data. The results show that compared with several traditional meta-heuristic scheduling algorithms, WDRL can effectively achieve the goal of optimizing task scheduling efficiency.Keywords
Cite This Article
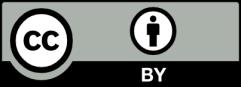
This work is licensed under a Creative Commons Attribution 4.0 International License , which permits unrestricted use, distribution, and reproduction in any medium, provided the original work is properly cited.