Open Access
ARTICLE
An Auto-Grading Oriented Approach for Off-Line Handwritten Organic Cyclic Compound Structure Formulas Recognition
Faculty of Artificial Intelligence in Education, Central China Normal University, Wuhan, 430079, China
* Corresponding Author: Bin He. Email:
(This article belongs to the Special Issue: Humanized Computing and Reasoning in Teaching and Learning)
Computer Modeling in Engineering & Sciences 2023, 135(3), 2267-2285. https://doi.org/10.32604/cmes.2023.023229
Received 15 April 2022; Accepted 22 August 2022; Issue published 23 November 2022
Abstract
Auto-grading, as an instruction tool, could reduce teachers’ workload, provide students with instant feedback and support highly personalized learning. Therefore, this topic attracts considerable attentions from researchers recently. To realize the automatic grading of handwritten chemistry assignments, the problem of chemical notations recognition should be solved first. The recent handwritten chemical notations recognition solutions belonging to the end-to-end trainable category suffered from the problem of lacking the accurate alignment information between the input and output. They serve the aim of reading notations into electrical devices to better prepare relevant e-documents instead of auto-grading handwritten assignments. To tackle this limitation to enable the auto-grading of handwritten chemistry assignments at a fine-grained level. In this work, we propose a component-detection-based approach for recognizing off-line handwritten Organic Cyclic Compound Structure Formulas (OCCSFs). Specifically, we define different components of OCCSFs as objects (including graphical objects and text objects), and adopt the deep learning detector to detect them. Then, regarding the detected text objects, we introduce an improved attention-based encoder-decoder model for text recognition. Finally, with these detection results and the geometric relationships of detected objects, this article designs a holistic algorithm for interpreting the spatial structure of handwritten OCCSFs. The proposed method is evaluated on a self-collected data set consisting of 3000 samples and achieves promising results.Keywords
Cite This Article
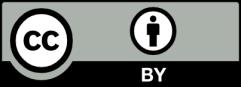
This work is licensed under a Creative Commons Attribution 4.0 International License , which permits unrestricted use, distribution, and reproduction in any medium, provided the original work is properly cited.