Open Access
ARTICLE
Prediction of Flash Flood Susceptibility of Hilly Terrain Using Deep Neural Network: A Case Study of Vietnam
1 University of Transport Technology, Hanoi, 100000, Vietnam
2 Department of Civil, Environmental and Natural Resources Engineering, Lulea University of Technology, Lulea, 971 87, Sweden
3 Department of Civil Engineering, Transilvania University of Brașov, Brasov, 500152, Romania
4 Danube Delta National Institute for Research and Development, Tulcea, 820112, Romania
5 Departement of Geodesy and Geomatics, National University of Civil Engineering, Hanoi, 100000, Vietnam
6 Faculty of Hydraulic Engineering, National University of Civil Engineering, Hanoi, 100000, Vietnam
7 DDG (R) Geological Survey of India, Gandhinagar, 382010, India
* Corresponding Authors: Nadhir Al-Ansari. Email: ; Romulus Costache. Email:
(This article belongs to the Special Issue: Computational Intelligent Systems for Solving Complex Engineering Problems: Principles and Applications)
Computer Modeling in Engineering & Sciences 2023, 135(3), 2219-2241. https://doi.org/10.32604/cmes.2023.022566
Received 15 March 2022; Accepted 30 May 2022; Issue published 23 November 2022
Abstract
Flash floods are one of the most dangerous natural disasters, especially in hilly terrain, causing loss of life, property, and infrastructures and sudden disruption of traffic. These types of floods are mostly associated with landslides and erosion of roads within a short time. Most of Vietnam is hilly and mountainous; thus, the problem due to flash flood is severe and requires systematic studies to correctly identify flood susceptible areas for proper landuse planning and traffic management. In this study, three Machine Learning (ML) methods namely Deep Learning Neural Network (DL), Correlation-based Feature Weighted Naive Bayes (CFWNB), and Adaboost (AB-CFWNB) were used for the development of flash flood susceptibility maps for hilly road section (115 km length) of National Highway (NH)-6 in Hoa Binh province, Vietnam. In the proposed models, 88 past flash flood events were used together with 14 flash floods affecting topographical and geo-environmental factors. The performance of the models was evaluated using standard statistical measures including Receiver Operating Characteristic (ROC) Curve, Area Under Curve (AUC) and Root Mean Square Error (RMSE). The results revealed that all the models performed well (AUC > 0.80) in predicting flash flood susceptibility zones, but the performance of the DL model is the best (AUC: 0.972, RMSE: 0.352). Therefore, the DL model can be applied to develop an accurate flash flood susceptibility map of hilly terrain which can be used for proper planning and designing of the highways and other infrastructure facilities besides landuse management of the area.Keywords
Cite This Article
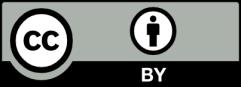
This work is licensed under a Creative Commons Attribution 4.0 International License , which permits unrestricted use, distribution, and reproduction in any medium, provided the original work is properly cited.