Open Access
ARTICLE
Ranked-Set Sampling Based Distribution Free Control Chart with Application in CSTR Process
1 Department of Mathematics, College of Science, King Khalid University, Abha, 62529, Saudi Arabia
2 Statistical Research and Studies Support Unit, King Khalid University, Abha, 62529, Saudi Arabia
3 School of Mathematics and Statistics, Xi’an Jiaotong University China, Xi’an, 710049, China
4 Department of Mathematics, Women University of Azad Jammu and Kashmir, Bagh, 12500, Pakistan
5 Government Post Graduate College, Haripur, 22620, Pakistan
6 Department of Statistics, University of Azad Jammu and Kashmir, Muzaffarabad, 13100, Pakistan
7 Department of Mathematics, Air University, Islamabad, 44000, Pakistan
* Corresponding Author: Zahid Rasheed. Email:
(This article belongs to the Special Issue: New Trends in Statistical Computing and Data Science)
Computer Modeling in Engineering & Sciences 2023, 135(3), 2091-2118. https://doi.org/10.32604/cmes.2023.022201
Received 26 February 2022; Accepted 15 July 2022; Issue published 23 November 2022
Abstract
Nonparametric (distribution-free) control charts have been introduced in recent years when quality characteristics do not follow a specific distribution. When the sample selection is prohibitively expensive, we prefer ranked-set sampling over simple random sampling because ranked set sampling-based control charts outperform simple random sampling-based control charts. In this study, we proposed a nonparametric homogeneously weighted moving average based on the Wilcoxon signed-rank test with ranked set sampling () control chart for detecting shifts in the process location of a continuous and symmetric distribution. Monte Carlo simulations are used to obtain the run length characteristics to evaluate the performance of the proposed control chart. The proposed control chart’s performance is compared to that of parametric and nonparametric control charts. These control charts include the exponentially weighted moving average (EWMA) control chart, Wilcoxon signed-rank with simple random sampling based the nonparametric EWMA control chart, the nonparametric EWMA sign control chart, Wilcoxon signed-rank with ranked set sampling-based the nonparametric EWMA control chart, and the homogeneously weighted moving average control charts. The findings show that the proposed control chart performs better than its competitors, particularly for the small shifts. Finally, an example is presented to demonstrate how the proposed scheme can be implemented practically.Keywords
The statistical process control (SPC) toolkit collects various statistical tools used to monitor the production and service processes. Control charts are one of the most important tools in the SPC toolkit for detecting changes in process parameters (location and/or dispersion). There are numerous control charts for efficient process monitoring in the SPC literature. Shewhart [1] introduced the basic control charts, called the Shewhart control charts. These control charts are also known as memoryless control charts because they only use current process information. Although the Shewhart control charts are simple to design and implement, they are less sensitive for small to moderate shifts detection. The other control charts that are more sensitive than the Shewhart control charts are the Cumulative sum (CUSUM) control chart, introduced by Page [2], and the exponentially weighted moving average (EWMA) control chart suggested by Roberts [3]. The CUSUM and EWMA control charts are also called the memory control chart, as these control charts use both current and previous information about the process.
Generally, it is assumed that the process often follows a specific distribution. When this assumption is violated, the quality of these objects may be affected. Nonparametric (NP) control charts are used in this scenario because they do not require the assumption of specified distribution. In addition, the population variance is unnecessary when employing NP control charts to observe the shifts in the process location. The sign (SN) and Wilcoxon signed-rank (SR) are commonly used NP techniques in the control charts context. For instance, Yang et al. [4] studied the NP EWMA sign (NPEWMA-SN) control chart for monitoring shifts in process location. Similarly, Graham et al. [5] and Graham et al. [6] investigated single observation-based NP EWMA sign and NP EWMA signed-rank control charts to monitor process location, respectively. Likewise, Chakraborty et al. [7] introduced the generally weighted moving average SN (GWMA-SN) control chart to detect the process location shifts. Later, Abid et al. [8] offered the ranked set sampling (RSS) based NP EWMA Wilcoxon signed-rank (NPREWMA-SR) control chart for monitoring shifts in process location efficiently. Moreover, Ali et al. [9] recommended NP control charts to detect location parameter changes. Also, Ali et al. [9] designed the RSS-based NP EWMA SN (NPREWMA-SN) control chart for monitoring shifts in process location.
The CUSUM and EWMA types schemes and modified versions are used for efficient process monitoring. For instance, Lucas et al. [10] offered the combined Shewhart-EWMA (CS-EWMA) control chart to enhance the shift detection ability in the process location. Similarly, Shamma et al. [11] proposed a double EWMA (DEWMA) chart, outperforming the EWMA control chart in early process location shifts detection. Likewise, Zhang et al. [12] introduced the DEWMA chart’s run length (RL) features. They suggested that DEWMA and EWMA charts are equally beneficial for detecting large shifts in process parameters. Later, Abbas et al. [13] and Zaman et al. [14] designed the mixed EWMA-CUSUM (MEC) and mixed CUSUM-EWMA (MCE) charts, respectively, to efficiently monitor process location shifts. Also, Anwar et al. [15] suggested auxiliary information-based (AIB) MEC and MCE control charts for monitoring the process location parameter. For other CUSUM and EWMA based studies, the readers are referred to the work of Adegoke et al. [16], Sanusi et al. [17], Haq [18], Zaman et al. [19], Aslam et al. [20], Adeoti et al. [21], Anwar et al. [15], Abbasi et al. [22], Rasheed et al. [23], Rasheed et al. [24], Anwar et al. [25], Liu et al. [26], and Rasheed et al. [27].
Hunter [28] noticed that the EWMA control chart assigns a higher weight to current observations and less weight to prior observations of the process. Later, Abbas [29] proposed the homogeneously weighted moving average (HWMA) control chart for observing shifts in process location. After that, Abid et al. [30] introduced the mixed HWMA-CUSUM (MHC) control chart for monitoring shifts in the process location. Similarly, Adeoti et al. [31] advocated a hybrid HWMA (HHWMA) control chart to detect the process location shifts. In addition, Abid et al. [32] offered the double HWMA (DHWMA) control chart to observe process location shifts. They showed that the DHWMA control chart has better detection ability than the HWMA control chart. Recently, Anwar et al. [33] suggested an AIB DHWMA control chart for improved process location monitoring.
Simple random sampling (SRS) and perfect RSS are frequently used in SPC to monitor underlying process data. These sampling techniques are commonly used with parametric and nonparametric control charts. However, when actual measurements are difficult to obtain, or the cost of sampling is prohibitively expensive, the RSS should be used instead of SRS. Ranking errors can impact estimator performance and result in ambiguous estimates. Dell et al. [34] studied the impacts of inaccuracy and imperfect RSS on the performance of mean estimators. They claim that the RSS mean estimator remains unbiased when imperfect ranking is used and outperforms the SRS estimator. Besides that, the RSS’s efficiency remains higher than that of the imperfect RSS and SRS. Numerous NP HWMA type control charts based on simple random sampling (SRS) are available. Still, according to the author’s knowledge, no NP HWMA control chart based on perfect and imperfect RSS is present in the literature. Capitalizing this research gap, this article introduces an NP HWMA SR control chart with perfect and imperfect RSS (
The remainder of the paper is structured as follows: Section 2 offers the proposed control chart’s methodology and design and the competing control chart. Section 3 evaluates the IC and OOC performance of the proposed
2 Competing and Proposed Control Charts
This section explains the design structure of the proposed control chart and the competing control charts. The competing control charts include EWMA, NPEWMA-SR, NPEWMA-SN, NPREWMA-SR, and HWMA control charts. More details are included in the following subsections, given as follows.
2.1 Classical EWMA Control Chart
Suppose X is the process characteristic that follows a normal distribution, i.e.,
where
The L is the control chart coefficient, and its value is determined so that IC
Graham et al. [6] suggested the SRS-based NP EWMA SR (NPEWMA-SR) control chart for monitoring shifts in the process location. If
Bakir [35] and Abbas et al. [36] suggested that
where
If the plotting statistic
Suppose
where
The underlying process is said to be IC if
Abid et al. [8] recommended the RSS-based NP EWMA SR (NPREWMA-SR) control chart for monitoring the process location shifts. When obtaining measurements for a variable of interest is both expensive and complicated, RSS is desirable to SRS; however, ranking these measurements can be accomplished using a extensively available and cost-effective ranking technique.
When measured values are deleterious or costly, but ranking findings is comparatively simple, the RSS is effective [37]. The proposed structure based on RSS is a more efficient alternative to the usual nonparametric control chart relying on Wilcoxon signed rank statistic. RSS involves the selection of a simple random sample of size n from a particular population, followed by the ranking of the findings by experts. The RSS is mostly defined in terms of perfect and imperfect ranking. The perfect and imperfect ranking structures are similar in general. A correlation of one between the two variables is required to achieve perfect ranking in any bivariate normal model where the variable of interest (
Then, using RSS data from the hth cycle, the unbiased estimator of the population mean, as defined by Takahasi et al. [38], is described as:
Assume that the variable of interest X is difficult to measure and order, but that there is a linked variable Y that is correlated with X (Dell et al. [34]). A variable Y can be utilised to obtain the rank of X in the following way:
where Y and
For the
Then variance of
where
Different authors, like, Kim et al. [40], Abid et al. [8], and Abbas et al. [36] used the RSS-based Wilcoxon signed-rank statistic to monitor shifts in the process location, defined as follows:
where
One may consult [22] for further detail on the RSS approach.
The plotting statistic of the NPREWMA-SR control chart is defined as:
The initial value of the plotting statistic
The process is considered to be IC when
Abbas [29] designed the HWMA control chart to monitor the process location, which is defined by the plotting statistic, given as follows:
where
The control limits of the HWMA control chart are defined as
The process is said to IC when
2.6 Proposed
This subsection explains the methodology and formulation procedure of the proposed RSS-based NP HWMA control chart for process location shifts. The proposed control chart is labeled as the
The starting value of the statistic
As a result, the control limits of the
To detect the shifts in the process location, the proposed
The IC and OOC performances of the proposed
(i)
(ii)
(iii)
Subsection 3.1 offers the IC performance of the proposed
This subsection addresses the design and implementation of the proposed
3.1.1 Design and Implementation
The design of the proposed
(i) Generate a random sample from the underlying distribution.
(ii) Specify the design parameters, i.e.,
(iii) Draw a sample from any distribution used in this study.
(iv) Compute the plotting statistic
(v) Find
(vi) Plot
(vii) If
(viii) Repeat Steps (ii) to (vii) 50,000 times and calculate the
(ix) To compute
This Subsection highlights the proposed
The design parameters of the proposed control chart used to determine OOC efficiency are displayed in Table 2. In order to investigate the OOC performance of the proposed NPHWMA_RSS control chart, numerous values of δ ∈ (0.025, 0.05, 0.075, 0.10, 0.25, 0.50, 0.75, 1.00, 1.50, 2.00, 2.50, 3.00, 5.00) are practiced. The
Table 2, and Figs. 1–3 provide the following outcomes:
(i) The proposed chart’s IC
(ii) As the smoothing parameter values increase, the performance of the proposed
(iii) As the sample size increases, the shift detection ability of the proposed
(iv) The proposed
(v) The proposed chart’s
(vi) The RL distribution of the proposed
(vii) The efficiency of the proposed control chart with perfect RSS outperforms the efficiency of the proposed control chart with imperfect RSS. For example, with perfect RSS under normal distribution at
(viii) The
Figure 1: ARL characteristics of the proposed
Figure 2: ARL characteristics of the proposed
Figure 3: ARL characteristics of the proposed
Figure 4: ARL meaures of the proposed
This section compares the proposed chart against the competing charts, including EWMA, NPEWMA-SR, NPEWMA-SN, NPREWMA-SR, and HWMA control charts. The comparisons are given in the following subsection.
4.1 Proposed vs. EWMA Control Chart
The
For instance, with the same control chart properties, the proposed
4.2 Proposed vs. NPEWMA-SR Control Chart
In comparison with the NPEWMA-SR control chart, the proposed
Similarly, when we examine
4.3 Proposed vs. NPEWMA-SN Control Chart
The
4.4 Proposed vs. NPREWMA-SR Control Chart
The proposed
4.5 Proposed vs. HWMA Control Chart
The proposed
5 Illustrative Example Using Real Data
To demonstrate the applicability of the proposed
Figure 5: Real-life application of the proposed
6 Summary, Conclusions, and Future Recommendations
The normality assumption for the process is essential for parametric control charts to monitor process parameters (location and/or dispersion) shifts. If the process under consideration does not follow a normal distribution, the nonparametric (NP) control charts are used. In addition, the homogeneously weighted moving average (HWMA) control chart is introduced for improved process location monitoring. Moreover, the ranked set sampling (RSS) technique outperforms simple random sampling (SRS). This study proposed the NP HWMA control chart with the RSS scheme, which results in an NP HWMA Wilcoxon signed-rank chart under the RSS technique (
Acknowledgement: The authors extended their appreciation to the Deanship of Scientific Research at King Khalid University for funding this work through Large Groups Project under the Grant No. RGP.2/132/43. The authors express their gratitude to the editor and referees for their valuable time and efforts on our manuscript.
Funding Statement: Funds are available under the Grant No. RGP.2/132/43 at King Khalid University, Kingdom of Saudi Arabia.
Conflicts of Interest: The authors declare that they have no conflicts of interest to report regarding the present study.
References
1. Shewhart, W. A. (1930). Economic control of quality manufactured product. Bell Labs Technical Journal, 9(2), 364–389. [Google Scholar]
2. Page, E. S. (1954). Continuous inspection schemes. Biometrika, 41, 100–115. DOI 10.1093/biomet/41.1-2.100. [Google Scholar] [CrossRef]
3. Roberts, S. (1959). Control chart tests based on geometric moving averages. Technometrics, 1(3), 239–250. DOI 10.1080/00401706.1959.10489860. [Google Scholar] [CrossRef]
4. Yang, S. F., Lin, J. S., Cheng, S. W. (2011). A new nonparametric EWMA sign control chart. Expert Systems with Applications, 38(5), 6239–6243. DOI 10.1016/j.eswa.2010.11.044. [Google Scholar] [CrossRef]
5. Graham, M., Chakraborti, S., Human, S. (2011). A nonparametric EWMA sign chart for location based on individual measurements. Quality Engineering, 23, 227–241. DOI 10.1080/08982112.2011.575745. [Google Scholar] [CrossRef]
6. Graham, M., Chakraborti, S., Human, S. (2011). A nonparametric exponentially weighted moving average signed-rank chart for monitoring location. Computational Statistics & Data Analysis, 55, 2490–2503. DOI 10.1016/j.csda.2011.02.013. [Google Scholar] [CrossRef]
7. Chakraborty, N., Chakraborti, S., Human, S. W., Balakrishnan, N. (2016). A generally weighted moving average signed-rank control chart. Quality and Reliability Engineering International, 32(8), 2835–2845. DOI 10.1002/qre.1968. [Google Scholar] [CrossRef]
8. Abid, M., Nazir, H. Z., Riaz, M., Lin, Z. (2017). An efficient nonparametric EWMA Wilcoxon signed-rank chart for monitoring location. Quality and Reliability Engineering International, 33(3), 669–685. DOI 10.1002/qre.2048. [Google Scholar] [CrossRef]
9. Ali, S., Abbas, Z., Nazir, H. Z., Riaz, M., Zhang, X. et al. (2020). On designing non-parametric EWMA sign chart under ranked set sampling scheme with application to industrial process. Mathematics, 8(9), 1497. DOI 10.3390/math8091497. [Google Scholar] [CrossRef]
10. Lucas, J. M., Saccucci, M. S. (1990). Exponentially weighted moving average control schemes: Properties and enhancements. Technometrics, 32(1), 1–12. DOI 10.1080/00401706.1990.10484583. [Google Scholar] [CrossRef]
11. Shamma, S. E., Shamma, A. K. (1992). Development and evaluation of control charts using double exponentially weighted moving averages. International Journal of Quality and Reliability Management, 9(6), 18–25. DOI 10.1108/02656719210018570. [Google Scholar] [CrossRef]
12. Zhang, L., Chen, G. (2005). An extended EWMA mean chart. Quality Technology and Quantitative Management, 2, 39–52. DOI 10.1080/16843703.2005.11673088. [Google Scholar] [CrossRef]
13. Abbas, N., Riaz, M., Does, R. J. M. M. (2012). Mixed exponentially weighted moving average–cumulative sum charts for process monitoring. Quality and Reliability Engineering International, 29(3), 345–356. DOI 10.1002/qre.1385. [Google Scholar] [CrossRef]
14. Zaman, B., Riaz, M., Abbas, N., Does, R. J. M. M. (2015). Mixed cumulative sum–exponentially weighted moving average control charts: An efficient way of monitoring process location. Quality and Reliability Engineering International, 31, 1407–1421. DOI 10.1002/qre.1678. [Google Scholar] [CrossRef]
15. Anwar, S. M., Aslam, M., Riaz, M., Zaman, B. (2020). On mixed memory control charts based on auxiliary information for efficient process monitoring. Quality and Reliability Engineering International, 36(6), 1949–1968. DOI 10.1002/qre.2667. [Google Scholar] [CrossRef]
16. Adegoke, N. A., Riaz, M., Sanusi, R. A., Smith, A. N., Pawley, M. D. (2017). EWMA-type scheme for monitoring location parameter using auxiliary information. Computers & Industrial Engineering, 114, 114–119. DOI 10.1016/j.cie.2017.10.013. [Google Scholar] [CrossRef]
17. Sanusi, R. A., Riaz, M., Abbas, N. (2017). Combined shewhart CUSUM charts using auxiliary variable. Computers & Industrial Engineering, 105, 329–337. DOI 10.1016/j.cie.2017.01.018. [Google Scholar] [CrossRef]
18. Haq, A. (2018). A new adaptive EWMA control chart using auxiliary information for monitoring the process mean. Communications in Statistics-Theory and Methods, 47(19), 4840–4858. DOI 10.1080/03610926.2018.1448417. [Google Scholar] [CrossRef]
19. Zaman, B., Lee, M. H., Riaz, M., Abujiya, M. R. (2019). An adaptive approach to EWMA dispersion chart using Huber and Tukey functions. Quality and Reliability Engineering International, 35(6), 1542–1581. DOI 10.1002/qre.2460. [Google Scholar] [CrossRef]
20. Aslam, M., Anwar, S. M. (2020). An improved Bayesian modified-EWMA location chart and Its applications in mechanical and sport industry. PLoS One, 15(2), e0229422. DOI 10.1371/journal.pone.0229422. [Google Scholar] [CrossRef]
21. Adeoti, O. A., Malela-Majika, J. C. (2020). Double exponentially weighted moving average control chart with supplementary runs-rules. Quality Technology & Quantitative Management, 17(2), 149–172. DOI 10.1080/16843703.2018.1560603. [Google Scholar] [CrossRef]
22. Abbasi, S., Haq, A. (2019). Optimal CUSUM and adaptive CUSUM charts with auxiliary information for process mean. Journal of Statistical Computation and Simulation, 89(2), 337–361. DOI 10.1080/00949655.2018.1548619. [Google Scholar] [CrossRef]
23. Rasheed, Z., Zhang, H., Anwar, S. M., Zaman, B. (2021). Homogeneously mixed memory charts with application in the substrate production process. Mathematical Problems in Engineering, 2021, 2582210. DOI 10.1155/2021/2582210. [Google Scholar] [CrossRef]
24. Rasheed, Z., Zhang, H., Arslan, M., Zaman, B., Anwar, S. M. et al. (2021b). An efficient robust nonparametric triple EWMA Wilcoxon signed-rank control chart for process location. Mathematical Problems in Engineering, 2570198. DOI 10.1155/2021/2570198. [Google Scholar] [CrossRef]
25. Anwar, S. M., Aslam, M., Zaman, B., Riaz, M. (2021). Mixed memory control chart based on auxiliary information for simultaneously monitoring of process parameters: An application in glass field. Computers & Industrial Engineering, 156, 107284. DOI 10.1016/j.cie.2021.107284. [Google Scholar] [CrossRef]
26. Liu, X., Khan, M., Rasheed, Z., Anwar, S. M., Arslan, M. (2022). New hybrid EWMA charts for efficient process dispersion monitoring with application in automobile industry. Computer Modeling in Engineering & Sciences, 131(2), 1171–1195. DOI 10.32604/cmes.2022.019199. [Google Scholar] [CrossRef]
27. Rasheed, Z., Khan, M., Abiodun, N. L., Anwar, S. M., Khalaf, G. et al. (2022). Improved nonparametric control chart based on ranked set sampling with application of chemical data modelling. Mathematical Problems in Engineering, 2022, 7350204. DOI 10.1155/2022/7350204. [Google Scholar] [CrossRef]
28. Hunter, J. S. (1986). The exponentially weighted moving average. Journal of Quality Technology, 18(1), 203–210. DOI 10.1080/00224065.1986.11979014. [Google Scholar] [CrossRef]
29. Abbas, N. (2018). Homogeneously weighted moving average control chart with an application in substrate manufacturing process. Computers & Industrial Engineering, 120, 460–470. DOI 10.1016/j.cie.2018.05.009. [Google Scholar] [CrossRef]
30. Abid, M., Mei, S., Nazir, H. Z., Riaz, M., Hussain, S. (2020). A mixed HWMA-CUSUM mean chart with an application to manufacturing process. Quality and Reliability Engineering International, 37(2), 618–631. [Google Scholar]
31. Adeoti, O. A., Koleoso, S. O. (2020). A hybrid homogeneously weighted moving average control chart for process monitoring. Quality and Reliability Engineering International, 36(6), 2170–2186. DOI 10.1002/qre.2690. [Google Scholar] [CrossRef]
32. Abid, M., Shabbir, A., Nazir, H. Z., Sherwani, R. A. K., Riaz, M. (2020). A double homogeneously weighted moving average control chart for monitoring of the process mean. Quality and Reliability Engineering International, 36(5), 1513–1527. DOI 10.1002/qre.2641. [Google Scholar] [CrossRef]
33. Anwar, S. M., Aslam, M., Zaman, B., Riaz, M. (2021). An enhanced double homogeneously weighted moving average control chart to monitor process location with application in automobile field. Quality and Reliability Engineering International, 38(1), 174–194. DOI 10.1002/qre.2966. [Google Scholar] [CrossRef]
34. Dell, T. R., Clutter, J. L. (1972). Ranked set sampling theory with order statistics background. Biometrics, 28(2), 545–555. DOI 10.2307/2556166. [Google Scholar] [CrossRef]
35. Bakir, S. T. (2004). A distribution-free Shewhart quality control chart based on signed-ranks. Quality Engineering, 16(4), 613–623. DOI 10.1081/QEN-120038022. [Google Scholar] [CrossRef]
36. Abbas, Z., Nazir, H. Z., Abid, M., Akhtar, N., Riaz, M. (2020). Enhanced nonparametric control charts under simple and ranked set sampling schemes. Transactions of the Institute of Measurement and Control, 42(14), 2744–2759. DOI 10.1177/0142331220931977. [Google Scholar] [CrossRef]
37. Mclntyre, G. A. (1952). A method for unbiased selective sampling using ranked sets. Australian Journal of Agricultural Research, 3(4), 385–390. DOI 10.1071/AR9520385. [Google Scholar] [CrossRef]
38. Takahasi, K., Wakimoto, K. (1968). On unbiased estimates of the population mean based on the sample stratified by means of ordering. Annals of the Institute of Statistical Mathematics, 20(1), 1–31. DOI 10.1007/BF02911622. [Google Scholar] [CrossRef]
39. Stokes, S. L. (1977). Ranked set sampling with concomitant variables. Communications in Statistics-Theory and Methods, 6(12), 1207–1211. DOI 10.1080/03610927708827563. [Google Scholar] [CrossRef]
40. Kim, D. A., Kim, Y. C. (1996). Wilcoxon signed rank test using ranked-set sampling. Korean Journal Computational and Applied Mathematics, 3(2), 235–243. DOI 10.1007/BF03008904. [Google Scholar] [CrossRef]
41. Montgomery, D. C. (2009). Introduction to statistical quality control. New York: John Willey & Sons. [Google Scholar]
42. Almanjahie, I. M., Dar, J. G., Al-Omari, A. I., Mir, A. (2021). Quantile version of Mathai-Haubold entropy of order statistics. Computer Modeling in Engineering & Sciences, 128(3), 907–925. DOI 10.32604/cmes.2021.014896. [Google Scholar] [CrossRef]
43. Lucas, J. M., Crosier, R. B. (1982). Fast initial response for CUSUM quality-control schemes: Give your CUSUM a head start. Technometrics, 24(3), 199–205. DOI 10.1080/00401706.1982.10487759. [Google Scholar] [CrossRef]
44. Yoon, S., MacGregor, J. (2001). Fault dignosis with multivariate statistical models part I: Using steady state fault signatures. Journal of Process Control, 11, 387–400. DOI 10.1016/S0959-1524(00)00008-1. [Google Scholar] [CrossRef]
45. Shi, X., Lv, Y., Fei, Z., Liang, J. (2013). A multivariable statistical process monitoring method based on multiscale analysis and principal curves. International Journal of Innovative Computing, Information and Control, 9, 1781–1800. [Google Scholar]
46. Ridwan, A. S., Muhammad, R., Nurudeen, A. A., Min, X. (2017). An EWMA monitoring scheme with a single auxiliary variable for industrial processes. Computers & Industrial Engineering, 114, 1–10. DOI 10.1016/j.cie.2017.10.001. [Google Scholar] [CrossRef]
47. Adegoke, N. A., Smith, A. N., Anderson, M. J., Sanusi, R. A., Pawley, M. D. (2019). Efficient homogeneously weighted moving average chart for monitoring process mean using an auxiliary variable. IEEE Access, 7, 94021–94032. DOI 10.1109/Access.6287639. [Google Scholar] [CrossRef]
Appendix A
According to Kim et al. [40], for an in control process
A.1.
A.2.
A.3.
For an in control process
Hence
Appendix B
B.1.
The mean of
B.2.
The variance of
If
As
If
Hence
Cite This Article
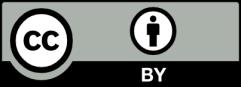
This work is licensed under a Creative Commons Attribution 4.0 International License , which permits unrestricted use, distribution, and reproduction in any medium, provided the original work is properly cited.