Open Access
ARTICLE
Modeling Drug Concentration in Blood through Caputo-Fabrizio and Caputo Fractional Derivatives
1
Department of Mathematics and Statistics, College of Science, King Faisal University, Hafuf, Al Ahsa, 31982, Saudi Arabia
2
Institute of Statistics, Biostatistics and Actuarial Science, Faculty of Science, Universite Catholique de Louvain,
Louvain-La-Neuve, 1348, Belgium
3
Department of Mathematics, Hodeidah University, Al-Hudaydah, 3114, Yemen
* Corresponding Author: Muath Awadalla. Email:
(This article belongs to the Special Issue: Applications of Fractional Operators in Modeling Real-world Problems: Theory, Computation, and Applications)
Computer Modeling in Engineering & Sciences 2023, 135(3), 2767-2785. https://doi.org/10.32604/cmes.2023.024036
Received 22 May 2022; Accepted 12 August 2022; Issue published 23 November 2022
Abstract
This study focuses on the dynamics of drug concentration in the blood. In general, the concentration level of a drug in the blood is evaluated by the mean of an ordinary and first-order differential equation. More precisely, it is solved through an initial value problem. We proposed a new modeling technique for studying drug concentration in blood dynamics. This technique is based on two fractional derivatives, namely, Caputo and Caputo-Fabrizio derivatives. We first provided comprehensive and detailed proof of the existence of at least one solution to the problem; we later proved the uniqueness of the existing solution. The proof was written using the Caputo-Fabrizio fractional derivative and some fixed-point techniques. Stability via the Ulam-Hyers (UH) technique was also investigated. The application of the proposed model on two real data sets revealed that the Caputo derivative was more suitable in this study. Indeed, for the first data set, the model based on the Caputo derivative yielded a Mean Squared Error (MSE) of 0.03095 with a corresponding best value of fractional order of derivative of 1.00360. Caputo-Fabrizio-basedderivative appeared to be the second-best method for the problem, with an MSE of 0.04324 for a corresponding best fractional derivative order of 0.43532. For the second experiment, Caputo derivative-based model still performed the best as it yielded an MSE of 0.04066, whereas the classical and the Caputo-Fabrizio methods were tied with the same MSE of 0.07299. Another interesting finding was that the MSE yielded by the Caputo-Fabrizio fractional derivative coincided with the MSE obtained from the classical approach.Keywords
Drugs are often inoculated in human blood during the treatment of a disease. One interesting fact is that the concentration of the drug decreases over time once it is inoculated. The initial concentration of a drug and many other parameters are used by medical practitioners to determine the frequency at which a specific drug should be administrated to reach the goal of curing a disease.
The clinical development of a new drug involves many phases. During phase 1 of drug development, the experimenter routinely evaluates time elapse until a new regimen reaches its steady state. The steady state here refers to the state at which the concentration of a drug in the blood does not change consistently anymore over time. In practice, patients have to take several shots of the drug in order to recover. However, each time a dose of the drug is administrated, part of it spreads all over the body and exists through excretions. The remaining part will accumulate to form sediment. The steady-state is reached when the concentration of the new dose coincides with the concentration of sediment. To identify the time from the first inoculation to the steady-state, the patient’s blood is sampled at regular time span intervals and the drug concentration is measured from the sample [1–5].
Many research works were produced in line with the evaluation of the steady-state of a drug in blood plasma. An overview of the selected method was proposed by Maganti et al. [6] and included approaches such as a Bayesian approach based on Markov Chain Monte Carlo (MCMC); No-statistical-significance-of-trend (NOSTASOT; spline regression among others. In the same line, a non-Newtonian-based important factors approach was introduced by Johnston et al. [7].
Besides the few but consistent methods of evaluating the steady-state during phase I study are many other approaches that we believe can be used successfully. We think of Fractional Calculus (FC) in this regard. Indeed, over the past decades, many researchers have successfully applied FC to solve real-life problems. One can name a few of these successes just to mention. For instance, Reference [8] was a review of recent trends in the successful application of FC in Biology and medical sciences. Margin has shown in [9] that FC could be successfully used to describe the dynamic of the events that occur in human blood tissues. Toledo-Hernandez et al. [10] have produced a piece on fermentation process modeling using FC. Fatma et al. [11] have studied the spray of omicron SARS-CoV-2 variant of COVID-19. Naik et al. [12] have investigated the effect of prostitution on HIV transmission dynamics. Qureshi et al. [13] have studied the concentration of ethanol in blood using a fractional derivative. They are many such examples in which FC has been successfully applied in modeling real-life phenomena [14,15]. The list is non-exhaustive however, we would recommend to a reader with an interest in the application of fractional calculus in Biology and Medicine to read the following selected papers (see [16–25]).
Almost eighty years ago, more precisely in the year 1940, the mathematician Ulam asked about the stability of functional equations [26], and a year later the mathematician Heyer responded to the question. By setting the necessary conditions for the stability of functional equations [27]. This result was generalized to differential equations, and it was believed that Obloza was the first to make this generalization [28], after which Alsina and Ger published a scientific paper containing what is known today as the Hyers-Ulam stability [29], Rus investigated the Hyers-Ulam stability of differential and integral equations using the Gronwall lemma [30]. Recently, the Hyers-Ulam stability problems of several differential equations were investigated by using the method of integral operators, see [31–34].
In this work, we kept a similar approach to earlier discussed examples. Indeed, we introduced modeling of the drug’s concentration blood using two fractional derivatives, namely the Caputo and the Caputo-Fabrizio fractional derivatives. Moreover, some qualitative analyses of the aforementioned models were obtained.
The remaining work is organized as follows. A review of FC definitions is provided in Section 2. The Section 3 focuses on a review of drug concentration modeling in blood. Section 4 establishes the existence and uniqueness of FC based approach proposed in this work. Section 5 establishes the Ulam-Hyers stability of the proposed fractional model. Section 6 is dedicated to applications on real-life data and the final section is the conclusion and discussion.
2 Some Useful Fractional Calculus Elements
FC has a strong foundation built upon definitions. Nowadays, they exit many fractional derivatives, each of them having its specific advantages and down points. This section focuses on some FC concepts required for the rest of the paper.
Definition 2.1 [35,36]. Given
where
This function was introduced by the author of the same name in 1903 (see [37]). With the advent and spread of research in the field of FC, the function quickly became an essential tool for FC and particularly for fractional differential equations (FDE). In practice, most solutions of FDE are expressed in terms of the Mittag-Leffer function.
Another important derivative that is among the basis of FC is the Caputo fractional derivative. It is an integral form-based derivative defined as follows.
Definition 2.2 [35]. The Reimann-Liouville (RL) fractional integrals is defined by
Definition 2.3 [35]. The Caputo fractional derivative of order
where
Definition 2.4 [35]. Given f, a function defined such that,
It is even possible to derive another useful fractional derivative that is used in this work. Indeed, if the function f introduced in Definition 2.3 is such that
The Caputo-Fabrizio fractional integral exists and is also useful in solving FDE. The said integral is defined as follows:
Definition 2.5 [35]. Given
where M is a function with the property
We end the shortlist of fractional derivatives and integrals of this section with Riemann-Liouville definitions.
Definition 2.6 [35]. Reimann-Liouville (RL) fractional integral is given by the following formula:
So far in this section, three fractional derivatives and two fractional integrals have been introduced. To end this section, we will state without proving some important Lemmas. These lemmas are used in sequel in the process of proving the existence of a solution to the problem and the uniqueness of the existing solution to the core problem which is also defined in the coming sections.
Lemma 2.3 [38] (Krasnoselskii’s Theorem).
Let us consider a Banach space B, and a convex set Q such that Q ⊂ B. Moreover, let q ∈ Q, and let us assume that H can be written as
i)
ii)
Then it follows that:
1)
2) ∃ q ∈ ∂Q and
Lemma 2.4 [38]. (Banach’s Contraction mapping principle).
Let X is a Banach space and let the operator Q: X → X be a contraction. Then, Q admits a unique fixed point x ∈ X with Qx = x.
3 A Review of Literature on Modeling of Drug Concentration in Blood
This section provides an overview of pharmacokinetics theories and the classical approach to building the dynamic model. A common approach to overcoming disease is to provide the sick person appropriate drug that will target harmful germs. It is therefore very substantial to inoculate the appropriate dose of the drug at suitable time intervals. Phase I of the clinical study of any drug focus on determining these parameters. Pharmacokinetics is defined as the branch of medicine that studies the dynamic (kinetics) of the drug when it is inoculated in a living body. In phase I of a clinical study, blood samples are taken at a regular time interval to evaluate the drug concentration. This is a purely experimental approach. There also exists a theoretical approach that consists of using a mathematical model and some historical data to develop a predictive model. A model that would predict drug concentration levels in the blood over time. In general, interest among researchers in computational biology has increased significantly over the past decades. Juinn et al. have dedicated research [39] to the study of Pharmacokinetics and Metabolism during a drug development process. Absorption, distribution, metabolism, and elimination (ADME), Reference [40] was the set of processes through which a drug is utilized by an individual leading to a concentration decrease over time.
It was mentioned in an earlier section that drug concentration decreases over time. Hence, a suitable mathematical model to describe such a process can be an exponential decay model. The following differential equation describes the decrease observed over time.
where
This study aims to investigate the performance of fractional differential equations in modeling while comparing them with classical differential equations used for solving the same problem. In the next sections, Caputo’s derivative and Caputo-Fabrizio’s derivative are used to build a fractional counterpart of the differential equation Eq. (1). The said models are applied to real-life data sets and the error rates they produce are used for a comparative study. The corresponding counterpart of Eq. (1) based on Caputo’s derivative and Caputo-Fabrizio’s derivative are respectively defined as follows:
In order the simplify notation and for conformity purpose, let a kernel function
The following Lemmas introduce more generalized formulae of the solutions to the fractional drug concentration models with the initial condition, as defined by Eqs. (5) and (6).
Lemma 3.1: (see [35]). Given
where
There exists a solution to Eq. (7) and, the said solution can be written as follows:
where
Remark: If the real number
Using Lemma 3.1 the solution to Eq. (3), when the derivative is defined in Caputo sense is expressed as follow
Lemma 3.2 (see [35]). Let
has a unique solution which is moreover expressed by the following expression:
with
4 Existence Result for the Drug Concentration
Considering the initial value problem given by Eq. (6), and denoting by
Let an operator E: H → H be defined as follows:
Eq. (15) is used to prove the existence and uniqueness of the solution. Besides, Lemmas 2.3 and 2.4 are needed in the process of proving the existence of a solution to the model proposed in Eq. (6). It is also used to prove the uniqueness of the solution found.
Theorem 4.1: Let us consider the function
i)
for all
ii)
where,
Moreover, assume that the following inequality holds for the defined constant:
Based on above setting, one is sure of the existence of at least one solution to the problem Eq. (6), subject to its initial conditions.
Proof. Let us consider the close set
Let us introduce two operators,
Given two elements
hence
In the forthcoming step of the proof, we will show that the operator
then
Eq. (19) is an evidence that
Moreover,
Beside all the above properties, one can prove compactness of
For
The quantity defined on the right-hand side of the inequality Eq. (20) goes to zero as
Theorem 4.2: Let
then the solution to the initial value problem Eq. (6) is unique.
Proof. Consider the close set
On the other hand, the following inequality is derived from the quantity or norm of
Using Eqs. (21)–(22) leads to the following relation:
Eq. (23) implies that
The second step of this proof is to show that the operator E is a contraction. To do so, observe that
The relation given by Eq. (24) leads to
On can see from Eq. (25) that E is a contraction. Therefore, recalling Lemma 2.4 is enough to conclude that Eq. (6) has a unique in the closed interval
Having proven the existence and the uniqueness of a solution to the problem defined by Eq. (6), we finally summarize the analytical solutions (see [34]) for these fractional models as follows: Based on Lemma 3.1, the analytical solution for the drug concentration model via Caputo sense Eq. (5) is given by
Based on Lemma 3.2, the analytical solution for the drug concentration model via Caputo-Fabrizio sense Eq. (6) is given by
All the necessary theoretical work on the proposed approach was done in previous sections. In the next section stability of the model is studied.
5 Ulam-Hyers Stability Results
In this section, we discuss the UH and generalized UH (GUH) stability of the considered problem.
Definition 5.1. Let
there exists a solution C ∈ H of Eq. (6) with
Definition 5.2. Let
Remark 5.3.
Lemma 5.4 (Gronwall’s Lemma, [42]). Let T > 0, and d > 0. Assume that
then
By Remark 5.3, the solution of Eq. (31) can be formulated by
where
Hence, we will make the following remark.
Remark 5.5. Let
Now we are ready to announce the results of the stabilization results for UH and GUH.
Theorem 5.6. Under the hypotheses of Theorem 4.1. If
Proof. Let
has the unique solution
Since
Thus, by our assumptions, Remark 5.5, along with Eqs. (32) and (35), we obtain
As
From Lemma 5.4, we have
which implies
Set
Hence, Eq. (36) is UH stable in H. Moreover, if we set
Let us consider the following CF-problem:
and the CF-inequality
where
For any t ∈ [0, 3],
Therefore, i) and ii) hold. Also,
Let
From Theorem 4.1, we known (s1) with
Applying
Moreover, we have
For
Hence, problem Eq. (36) is UH stable. If we set
6 Applications on Real-Life Data
In this section, Caputo and Caputo-Fabrizio fractional derivatives are used to solve FDE for drug concentration modeling in blood. The next figure represents the so-called ADME process. It shows that drugs can be administrated through an oral or intravenous route. In this section, our experiments are carried out on the intravenous administration. Bold and red arrows are meant to highlight the paths considered in the study. The figure also shows where blood can be sampled to measure drug concentration at any time. It is important to mention that the oral route administration is not studied here because it falls apart in a completely different category of differential equations.
The following two experiments were designed with respect to Fig. 1. In the experiments solutions based on the classical approach, caputo and Caputo-Fabrizio approaches are used to fit the data sets. Comparisons are made to select the best-performing method.
Figure 1: ADME process, with a highlight on intravenous route
Experiment N
David Bourne (see [43]) recorded experimental data on drug concentration behavior over time. In the said experiment a drug (antibiotic) was inoculated to a 50 kg woman through intravenous injection. For 10 h, blood samples were taken, and their drug concentrations were measured. The recorded data set was used to fit models defined by Eqs. (3) and (4). Their best parameters as well as their error level are given in the table below.
Table 1 contains values of the parameters for each model. Recalling classical model; Caputo derivative-based model; Caputo-Fabrizio based model described by Eqs. (1), (3) and (4), respectively. Looking at the MSE (see [36]) of each model, Caputo derivative-based model has the best performance, with MSE = 0.03095, and
Note: Best parameters were obtained through optimization routine, in particular error minimization. The non-linear optimization routine, nlm from R-programming was used for the purpose.
Fig. 2 shows the original data points and the fitted curves obtained from the classical method and the Caputo method. Curves overlap each other, this is also illustrated by the MSE range, which is very small. It is however possible to observe a little split of both curves from the 6th hour on the x-axis but zoom out the page containing the figure.
Figure 2: Caputo and classical methods
Fig. 3 represents original data points alongside the fitted lines obtained through the classical method and Caputo-Fabrizio method. Both curves overlap each other all over the way. It is not surprising as both approaches have the same MSE.
Figure 3: Caputo-Fabrizio and classical methods
Experiment N
In this study, a newly developed drug was injected (Intravenous route) into a 50 kg female patient at a proportion of 20 mg/kg. Blood samples were taken on a regular basis and, the plasma was analyzed to determine the current drug concentration in the blood. The entire process generated a data set that was fitted by the mean of FDE based on Caputo and Caputo-Fabrizio derivative, defined by Eqs. (3) and (4), respectively. The data set was retrieved from [44]. Performances of the models involved in the study are consigned below.
Table 2 contains values of the parameters for each model. Recalling classical model; Caputo derivative-based model; Caputo-Fabrizio based model described by Eqs. (1), (3) and (4), respectively. Looking at the MSE (see [36]) of each model, Caputo derivative-based model has the best performance, with MSE = 0.20992, and
Fig. 4 shows the original data points and the fitted curves obtained from the classical method and the Caputo method. Although there are only a few data points, it is observed that the Caputo fitting line is closer to the original data points than the classical fitting line.
Figure 4: Caputo and classical methods from second experiment
Fig. 5 represents original data points alongside the fitted lines obtained through the classical method and Caputo-Fabrizio method.
Figure 5: Caputo-Fabrizio and classical methods from second experiment
The goal of this work is achieved. We have proven that fractional differential equations could be efficiently used for drug concentration modeling. In this regard, we have proven the existence and uniqueness of the solution based on some fixed point techniques. We have also provided a stability analysis in the Ulam-Hyers sense of the CF-type FDE model. Moreover, based on the results from the two experiments, it is observed that the Caputo derivative performs the best. On the other hand, the Caputo-Fabrizio and classical methods have the same MSE. Based on the results of the experiments, one can observe that they are modeling problems for which the fractional calculus approach does not perform better than the classical method. Indeed, results from both experiments show that the Caputo derivative-based model has the best performance whereas Caputo-Fabrizio derivative-based model and the classical approach have the same performance (MSE). Moreover, they are the second best models.
In this study, every fractional order of derivative that leads to the lowest MSE fulfills the condition
In future work, we aim to build a comprehensive framework for choosing the right fractional derivative for a given problem.
In practice, the outcome of this study can increase the accuracy of a new model’s parameters calibration. Indeed, if a new drug is developed, and if its concentration level in the blood (during phase IV) decreases over time following an exponential decay trend; then an FDE based on the Caputo derivative would be recommended for estimating the best parameters. The statement best parameters refer to the exponential decay constant,
Authors’ Contributions: All authors contributed equally and significantly to this paper. All authors read and approved the final manuscript.
Acknowledgement: The authors express their thanks to the Deanship of Scientific Research, Vice Presidency for Graduate Studies and Scientific Research, King Faisal University, Saudi Arabia, for supporting this work through the Annual Funding Track by [Project No. AN000273].
Data Availability Statement: The data set used in the study is freely available through the https://www.boomer.org/c/p4/c02/c0208.php, it is found at [43]. https://www.intechopen.com/chapters/49459 found at [44].
Funding Statement: This work was supported through the Annual Funding Track by the Deanship of Scientific Research, Vice Presidency for Graduate Studies and Scientific Research, King Faisal University, Saudi Arabia [Project No. AN000273], granted after a successful application by M.A.
Conflicts of Interest: The authors declare that they have no conflicts of interest to report regarding the present study.
References
- Muindi, J. R., Peng, Y., Potter, D. M., Hershberger, P. A., & Tauch, J. S. (2002). Pharmacokinetics of high-dose oral calcitriol: Results from a phase 1 trial of calcitriol and paclitaxel. Clinical Pharmacology & Therapeutics, 72(6), 648-659. [Google Scholar] [CrossRef]
- Rowland, M., Tozer, T. N. (1995). Clinical pharmacokinetics: Concepts and applications. Baltimore: Williams and Wilkins.
- Jordan, P., Brunschwig, H., & Luedin, E. (2008). Modeling attainment of steady state of drug concentration in plasma by means of a Bayesian approach using MCMC methods. Pharmaceutical Statistics, 7(1), 36-41. [Google Scholar] [CrossRef]
- Varadharajan, S., Winiwarter, S., Carlsson, L., Engkvist, O., & Anantha, A. (2015). Exploring in silico prediction of the unbound brain-to-plasma drug concentration ratio: Model validation, renewal, and interpretation. Journal of Pharmaceutical Sciences, 104(3), 1197-1206. [Google Scholar] [CrossRef]
- Zhang, D., Hop, C. E., Patilea-Vrana, G., Gampa, G., & Seneviratne, H. K. (2019). Drug concentration asymmetry in tissues and plasma for small molecule–related therapeutic modalities. Drug Metabolism and Disposition, 47(10), 1122-1135. [Google Scholar] [CrossRef]
- Maganti, L., Panebianco, D. L., & Maes, A. L. (2008). Evaluation of methods for estimating time to steady state with examples from phase 1 studies. The AAPS Journal, 10(1), 141-147. [Google Scholar] [CrossRef]
- Johnston, B. M., Johnston, P. R., Corney, S., & Kilpatrick, D. (2004). Non-newtonian blood flow in human right coronary arteries: Steady state simulations. Journal of Biomechanics, 37(5), 709-720. [Google Scholar] [CrossRef]
- Ionescu, C., Lopes, A., Copot, D., Machado, J. T., & Bates, J. H. (2017). The role of fractional calculus in modeling biological phenomena: A review. Communications in Nonlinear Science and Numerical Simulation, 51, 141-159. [Google Scholar] [CrossRef]
- Magin, R. L. (2010). Fractional calculus models of complex dynamics in biological tissues. Computers & Mathematics with Applications, 59(5), 1586-1593. [Google Scholar] [CrossRef]
- Toledo-Hernandez, R., Rico-Ramirez, V., Iglesias-Silva, G. A., & Diwekar, U. M. (2014). A fractional calculus approach to the dynamic optimization of biological reactive systems. Part I: Fractional models for biological reactions. Chemical Engineering Science, 117, 217-228. [Google Scholar] [CrossRef]
- Fatma, K., Yavuz, M., Tamer, M., & Rafla, H. (2022). Fractional order modelling of omicron SARS-CoV-2 variant containing heart attack effect using real data from the United Kingdom. Chaos, Solitons & Fractals, 157, 111954. [Google Scholar]
- Naik, P., Yavuz, M., & Zu, J. (2020). The role of prostitution on HIV transmission with memory: A modeling approach. Alexandria Engineering Journal, 59(4), 2513-2531. [Google Scholar] [CrossRef]
- Qureshi, S., Yusuf, A., Shaikh, A. A., Inc, M., & Baleanu, D. (2019). Fractional modeling of blood ethanol concentration system with real data application. Chaos: An Interdisciplinary Journal of Nonlinear Science, 29(1), 013143. [Google Scholar] [CrossRef]
- Özköse, F., Senel, M. T., & Habbireeh, R. (2021). Fractional-order mathematical modelling of cancer cells-cancer stem cells-immune system interaction with chemotherapy. Mathematical Modelling and Numerical Simulation with Applications, 1(2), 67-83. [Google Scholar] [CrossRef]
- Hammouch, Z., Yavuz, M., & Özdemir, N. (2021). Numerical solutions and synchronization of a variable-order fractional chaotic system. Mathematical Modelling and Numerical Simulation with Applications, 1(1), 11-23. [Google Scholar] [CrossRef]
- Evirgen, F., Sümeyra, U., Özdemir, N., & Zakia, H. (2021). System response of an alcoholism model under the effect of immigration via non-singular kernel derivative. American Institute of Mathematical Sciences, 14(7), 2199-2212. [Google Scholar]
- Anwarud, D., & Muhammad, Z. A. (2022). Analysis of fractional-order vaccinated hepatitis-B epidemic model with mittag-leffer kernels. Mathematical Modelling and Numerical Simulation with Applications, 2(2), [Google Scholar]
- Naik, P. A., Ghoreishi, M., & Zu, J. (2022). Approximate solution of a nonlinear fractional-order HIV model using homotopy analysis method. International Journal of Numerical Analysis and Modeling, 19(1), 52-84. [Google Scholar]
- Naik, P. A., Zu, J., & Owolabi, K. M. (2020). Modeling the mechanics of viral kinetics under immune control during primary infection of HIV-1 with treatment in fractional order. Physica A: Statistical Mechanics and its Applications, 545, 123816. [Google Scholar] [CrossRef]
- Naik, P. A., Yavuz, M., Qureshi, S., Zu, J., & Townley, S. (2020). Modeling and analysis of COVID-19 epidemics with treatment in fractional derivatives using real data from Pakistan. The European Physical Journal Plus, 135(10), 795. [Google Scholar] [CrossRef]
- Özköse, F., & Yavuz, M. (2022). Investigation of interactions between COVID-19 and diabetes with hereditary traits using real data: A case study in Turkey. Computers in Biology and Medicine, 141(3), 105044. [Google Scholar]
- Yusuf, A., Qureshi, S., Mustapha, U. T., Musa, S. S., & Sulaiman, T. A. (2022). Fractional modeling for improving scholastic performance of students with optimal control. International Journal of Applied and Computational Mathematics, 8(1), 1-20. [Google Scholar] [CrossRef]
- Qureshi, S., & Jan, R. (2021). Modeling of measles epidemic with optimized fractional order under caputo differential operator. Chaos, Solitons & Fractals, 145, 110766. [Google Scholar] [CrossRef]
- Qureshi, S., Yusuf, A., & Aziz, S. (2021). Fractional numerical dynamics for the logistic population growth model under conformable caputo: A case study with real observations. Physica Scripta, 96(11), 114002. [Google Scholar] [CrossRef]
- Qureshi, S. (2021). Fox H-functions as exact solutions for caputo type mass spring damper system under sumudu transform. Journal of Applied Mathematics and Computational Mechanics, 20(1), 83-89. [Google Scholar] [CrossRef]
- Ulam, S. M. (1960). Problems in modern mathematics. Science Editions. New York, NY, USA: Wiley.
- Hyers, D. H. (1941). On the stability of the linear functional equation. PNAS, 27, 222-224. [Google Scholar] [CrossRef]
- Obloza, M. (1993). Hyers stability of the linear differential equation. https://rep.up.krakow.pl/xmlui/bitstream/handle/11716/7688/RND159--16--Hyers-stability--Obloza.pdf?sequence=1.
- Alsina, C., & Ger, R. (1998). On some inequalities and stability results related to the exponential function. Journal of Inequalities and Applications, 2(4), 373-380. [Google Scholar] [CrossRef]
- Rus, I. A. (2009). Remarks on ulam stability of the operatorial equations. Fixed Point Theory, 10(2), 305-320. [Google Scholar]
- Li, Y., & Shen, Y. (2010). Hyers-ulam stability of linear differential equations of second order. Applied Mathematics Letters, 23(3), 306-309. [Google Scholar]
- Abdo, M. S., Thabet, S., & Ahmad, B. (2020). The existence and Ulam–Hyers stability results for. Journal of Pseudo-Differential Operators and Applications, 11(4), 1757-1780. [Google Scholar] [CrossRef]
- Ali, S. A., Shatanawi, W., Kassim, M. D., Abdo, M. S., Saleh, S. (2022). Investigating a class of generalized caputo-type fractional integro-differential equations. Journal of Function Spaces, 2022, 8103046. DOI 10.1155/2022/8103046. [CrossRef]
- Ali, S. A., Abdo, M. S. (2022). Qualitative analysis for multiterm langevin systems with generalized caputo fractional operators of different orders. Mathematical Problems in Engineering, 2022, 1879152. DOI 10.1155/2022/1879152. [CrossRef]
- Diethelm, K. (2010). The analysis of fractional differential equations: An application-oriented exposition using differential operators of caputo type. In: Lecture notes in mathematics.
- Awadalla, M., Yannick, Y. Y. N., Asbeh, K. A. (2020). Modeling the dependence of barometric pressure with altitude using Caputo and Caputo–Fabrizio fractional derivatives. Journal of Mathematics, 2020, 2417681. DOI 10.1155/2020/2417681. [CrossRef]
- Mittag-Leffer, G. M. (1903). Sur la Nouvelle Fonction E(x). Comptes Rendus de l’Academie des Sciences Paris, 137(2), 554-558. [Google Scholar]
- Noupoue, Y. Y. Y., Tandoğdu, Y., & Awadalla, M. (2019). On numerical techniques for solving the fractional logistic differential equation. Advances in Difference Equations, 2019(1), 108. [Google Scholar]
- Lin, J. H., & Lu, A. Y. (1997). Role of pharmacokinetics and metabolism in drug discovery and development. Pharmacological Reviews, 49(4), 403-449. [Google Scholar]
- Majee, S. B., Avlani, D., Ghosh, P., & Biswas, G. R. (2018). Absorption, distribution, metabolism and elimination (ADME) and toxicity profile of marine sulfated polysaccharides used in bionanotechnology. African Journal of Pharmacy and Pharmacology, 12(1), 1-10. [Google Scholar]
- Khanday, M. A., Rafiq, A., & Nazir, K. (2017). Mathematical models for drug diffusion through the compartments of blood and tissue medium. Alexandria Journal of Medicine, 53(3), 245-249. [Google Scholar] [CrossRef]
- Gronwall, T. H. (1919). Note on the derivatives with respect to a parameter of the solutions of a system of differential equations. Annals of Mathematics,, 20, 292-296. [Google Scholar] [CrossRef]
- David, B. (2022). Basic pharmacokinetics. https://www.boomer.org/c/p4/c02/c0208.php.
- Tarek, A. A. (2022). Pharmacokinetics of drugs following IV bolus, IV infusion, and oral administration. https://www.intechopen.com/chapters/49459.
Cite This Article
Awadalla, M., Abuasbeh, K., Yannick, Y., Abdo, M. S. (2023). Modeling Drug Concentration in Blood through Caputo-Fabrizio and Caputo Fractional Derivatives. CMES-Computer Modeling in Engineering & Sciences, 135(3), 2767–2785. https://doi.org/10.32604/cmes.2023.024036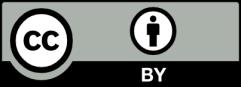