Open Access
EDITORIAL
Introduction to the Special Issue on Soft Computing Techniques in Materials Science and Engineering
1 Department of Civil Engineering, Computational Mechanics Laboratory, School of Pedagogical and Technological Education, Athens, 15122, Greece
2 Department of Urban Planning, Engineering Networks and Systems, Institute of Architecture and Construction, South Ural State University, Chelyabinsk, 454080, Russia
3 Department of Engineering, University of Palermo, Palermo, 90128, Italy
4 Department of Surface Mining, Mining Faculty, Hanoi University of Mining and Geology (HUMG), Hanoi, 100000, Vietnam
* Corresponding Author: Panagiotis G. Asteris. Email:
(This article belongs to the Special Issue: Soft Computing Techniques in Materials Science and Engineering)
Computer Modeling in Engineering & Sciences 2023, 135(2), 839-841. https://doi.org/10.32604/cmes.2023.025694
Received 26 July 2022; Accepted 05 August 2022; Issue published 27 October 2022
Abstract
This article has no abstract.Soft computing (SC) refers to the ability of a digital computer or robot to perform functions that are normally associated with intelligent individuals, such as reasoning and problem-solving. An example of this would be a project aimed at creating systems capable of reasoning, discovering meaning, generalising, or learning from past experience. Science and engineering problems that are both non-linear and complex can be solved using these methodologies. It has been proven that these algorithms can be used to solve numerous real-world problems. The techniques outlined can be used to increase the accuracy of existing models/equations, or they can be used to propose a new model that can address the problem.
Due to the increasing importance of these models, this thematic issue is aimed at shedding some light on the applications of SC algorithms in solving problems in Materials Science and Engineering. The thematic issue comprises nine papers, which are briefly summarised in the following paragraphs. The implemented methods in the following papers can be applied with caution to other science and engineering problems by researchers.
The first paper, entitled “A Novel Heuristic Algorithm for the Modelling and Risk Assessment of the COVID-19 Pandemic Phenomenon” [1], presented a novel SC technique for analysing the risk assessment of COVID-19 daily death cases in various countries (different parts of the world). Their model was able to categorise and predict the path of the pandemic phenomena while at the same time providing a useful tool for public health authorities in decision-making and operational planning. This paper, because of its importance, has been cited many times so far.
The second paper, entitled “The Influence of Various Structure Surface Boundary Conditions on Pressure Characteristics of Underwater Explosion” [2] was an excellent application of the hybrid finite element method (FEM) in the underwater explosion interaction. The authors proposed a Runge–Kutta discontinuous Galerkin method combined with FEM to solve the problem of the complex waves of fluids and structural dynamic response. Based on the results, the authors came up with some ideas for how the risk could be reduced in different situations.
The third paper, entitled “Maximum Probabilistic and Dynamic Traffic Load Effects on Short-to-Medium Span Bridges” [3] was another interesting paper presenting a probabilistic method in traffic and transportation engineering. The idea was to conduct an efficient and accurate extrapolation, developing a framework on short-to-medium span bridges using practical heavy traffic data in China. The designed vehicle load model is conservative compared to some available design codes.
The fourth paper, entitled “Evaluating the Clogging Behavior of Pervious Concrete (PC) Using the Machine Learning Techniques” [4] presented a novel hybrid model which was a combination of support vector machine and particle swarm optimization in predicting an important issue in concrete technology. The hybrid model was used to determine the clogging behaviour of previous concrete based on a database with 84 data points. The particle swarm optimization algorithm was able to effectively adjust the hyperparameters of the support vector machine model, resulting in obtaining a high level of accuracy. The authors mentioned that their proposed model can be used in other relevant problems.
The fifth paper, entitled “Prerequisite Relations among Knowledge Units: A Case Study of Computer Science Domain” [5] proposed a statistical model for measuring dependencies in learning resources between knowledge units. Instructors are expected to present knowledge units in a semantically well-organized manner to facilitate students’ understanding of the material. The proposed model reveals how inner concepts of a knowledge unit are dependent on each other and on concepts not in the knowledge unit. The evaluation, employing three textbooks, shows that the proposed model succeeds in discovering hidden relationships knowledge units in learning resources and in exposing the knowledge gaps in some knowledge among units.
The sixth paper, entitled “Novel Time Series Bagging Based Hybrid Models for Predicting Historical Water Levels in the Mekong Delta Region, Vietnam” [6] estimated the historical water levels of the Mekong River delta using data collected from 2000 to 2018. This estimation was in the category of time series problems and the authors used different bagging-based models, namely: Bagging (RF), Bagging (SOM) and Bagging (M5P). A high level of accuracy and a low level of system error were obtained by these models. The authors concluded that these Bagging-based hybrid models are promising tools, and they can be used for accurate prediction of water levels in other projects.
The seventh paper, entitled “Seismic finite Performance of Assembled Shear Wall with Defective Sleeve Connection” [7], three kinds of shear walls with full sleeve grouting, fully defective sleeve and partially defective were designed for element analysis to analyze the influence of defects on the seismic performance of shear walls. The research shows that at the beginning of loading (5 s), the three models begin to appear compressive damage at the bottom of the wall in all three models. FEM methods are used. The stiffness degradation curves of the three models basically overlap with one another. Before the limit displacement, the stiffness results of the non-defect model and the partially defective model are greater than that of the fully defective model. When the displacement is loaded to 20 mm, the stiffness degradation of the three models is equivalent.
The eighth paper, entitled “Structural Damage Identification Using Ensemble Deep Convolutional Neural Network Models” [8] conducted a series of analyses, evaluations, and examinations to develop a practical way of identifying structural damage. The name of their developed model is a hybrid weighted averaging ensemble and differential evolution (WAE-DE) ensemble model, which was trained using a total of 4585 images from different damage types. Their ensemble model received a high accuracy level from the evaluation indices for the testing stage. The authors have shown the WAE-DE, as a successful general model. They mentioned that the highest possible accuracy can be obtained with some specific SC models.
The last paper published in this special issue, entitled “Rock Strength Estimation Using Several Tree-Based ML Techniques” [9], was presented in the area of civil-geotechnics, and the authors introduced a series of tree-based models to predict rock strength. The idea was to use simple rock index tests as inputs to estimate rock strength as these tests are easy to conduct and cheap. The extreme gradient boosting machine was selected as the best tree-based model in this study. The authors also performed a series of important analyses on the input parameters and their effects on the system output. The way of examining these effects is interesting and can be applied in other similar studies.
Funding Statement: The authors received no specific funding for this study
Conflicts of Interest: The authors declare that they have no conflicts of interest to report regarding the present study
References
1. Asteris, P. G., Douvika, M. G., Karamani, C. A., Skentou, A. D., Chlichlia, K. et al. (2020). A novel heuristic algorithm for the modeling and risk assessment of the COVID-19 pandemic phenomenon. Computer Modeling in Engineering & Sciences, 125(2), 815–828. DOI 10.32604/cmes.2020.013280. [Google Scholar] [CrossRef]
2. Qin, Y., Wang, Y., Wang, Z., Yao, X. (2021). The influence of various structure surface boundary conditions on pressure characteristics of underwater explosion. Computer Modeling in Engineering & Sciences, 126(3), 1093–1123. DOI 10.32604/cmes.2021.012969. [Google Scholar] [CrossRef]
3. Lu, N., Wang, H., Wang, K., Liu, Y. (2021). Maximum probabilistic and dynamic traffic load effects on short-to-medium span bridges. Computer Modeling in Engineering & Sciences, 127(1), 345–360. DOI 10.32604/cmes.2021.013792. [Google Scholar] [CrossRef]
4. Huang, J., Zhang, J., Gao, Y. (2022). Evaluating the clogging behavior of pervious concrete (PC) using the machine learning techniques. Computer Modeling in Engineering & Sciences, 130(2), 805–821. DOI 10.32604/cmes.2022.017792. [Google Scholar] [CrossRef]
5. Nafa, F., Babour, A., Melton, A. (2022). Prerequisite relations among knowledge units: A case study of computer science domain. Computer Modeling in Engineering & Sciences, 133(3), 639–652. DOI 10.32604/cmes.2022.020084. [Google Scholar] [CrossRef]
6. Hoan, N. T., Dung, N. V., Thu, H. L., Quynh, H. T., Al-Ansari, N. et al. (2022). Novel time series bagging based hybrid models for predicting historical water levels in the Mekong Delta region, Vietnam. Computer Modeling in Engineering & Sciences, 131(3), 1431–1449. DOI 10.32604/cmes.2022.018699. [Google Scholar] [CrossRef]
7. Yan, H., Song, B., Xu, D., Zhang, G. (2022). Seismic performance of assembled shear wall with defective sleeve connection. Computer Modeling in Engineering & Sciences, 131(1), 199–217. DOI 10.32604/cmes.2022.016312. [Google Scholar] [CrossRef]
8. Barkhordari, M. S., Armaghani, D. J., Asteris, P. G. (2023). Structural damage identification using ensemble deep convolutional neural network models. Computer Modeling in Engineering & Sciences, 134(2), 835–855. DOI 10.32604/cmes.2022.020840. [Google Scholar] [CrossRef]
9. Liu, Z., Armaghani, D. J., Fakharian, P., Li, D., Ulrikh, D. V. et al. (2022). Rock strength estimation using several tree-based ML techniques. Computer Modeling in Engineering & Sciences, 133(3), 799–824. DOI 10.32604/cmes.2022.021165. [Google Scholar] [CrossRef]
Cite This Article
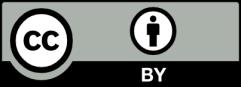
This work is licensed under a Creative Commons Attribution 4.0 International License , which permits unrestricted use, distribution, and reproduction in any medium, provided the original work is properly cited.