Open Access
ARTICLE
An Interpretable CNN for the Segmentation of the Left Ventricle in Cardiac MRI by Real-Time Visualization
1 Robotics Institute, School of Computer Science, Carnegie Mellon University, Pittsburgh, PA, 15217, USA
2 Department of Electrical & Computer Engineering, College of Engineering, Northeastern University, Boston, MA, 02115, USA
3 Security and Optimization for Networked Globe Laboratory (SONG Lab), Embry-Riddle Aeronautical University, Daytona Beach, FL, 32114, USA
4 School of Computer Science and Engineering, University of Electronic Science and Technology of China, Chengdu, 610054, China
* Corresponding Author: Liang Luo. Email:
(This article belongs to the Special Issue: Models of Computation: Specification, Implementation and Challenges)
Computer Modeling in Engineering & Sciences 2023, 135(2), 1571-1587. https://doi.org/10.32604/cmes.2022.023195
Received 14 April 2022; Accepted 06 July 2022; Issue published 27 October 2022
Abstract
The interpretability of deep learning models has emerged as a compelling area in artificial intelligence research. The safety criteria for medical imaging are highly stringent, and models are required for an explanation. However, existing convolutional neural network solutions for left ventricular segmentation are viewed in terms of inputs and outputs. Thus, the interpretability of CNNs has come into the spotlight. Since medical imaging data are limited, many methods to fine-tune medical imaging models that are popular in transfer models have been built using massive public ImageNet datasets by the transfer learning method. Unfortunately, this generates many unreliable parameters and makes it difficult to generate plausible explanations from these models. In this study, we trained from scratch rather than relying on transfer learning, creating a novel interpretable approach for autonomously segmenting the left ventricle with a cardiac MRI. Our enhanced GPU training system implemented interpretable global average pooling for graphics using deep learning. The deep learning tasks were simplified. Simplification included data management, neural network architecture, and training. Our system monitored and analyzed the gradient changes of different layers with dynamic visualizations in real-time and selected the optimal deployment model. Our results demonstrated that the proposed method was feasible and efficient: the Dice coefficient reached 94.48%, and the accuracy reached 99.7%. It was found that no current transfer learning models could perform comparably to the ImageNet transfer learning architectures. This model is lightweight and more convenient to deploy on mobile devices than transfer learning models.Keywords
Cite This Article
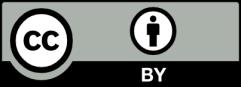