Open Access
ARTICLE
Quantifying Solid Solution Strengthening in Nickel-Based Superalloys via High-Throughput Experiment and Machine Learning
1 State Key Laboratory of Powder Metallurgy, Central South University, Changsha, 410083, China
2 AECC Commercial Aircraft Engine Co., Ltd., Shanghai, 200241, China
3 State Key Laboratory for Strength and Vibration of Mechanical Structures, School of Aerospace Engineering, Xi’an, 710049, China
* Corresponding Authors: Liming Tan. Email: ; Xiaochao Jin. Email:
# These authors contributed equally to this work
Computer Modeling in Engineering & Sciences 2023, 135(2), 1521-1538. https://doi.org/10.32604/cmes.2022.021639
Received 25 January 2022; Accepted 15 June 2022; Issue published 27 October 2022
Abstract
Solid solution strengthening (SSS) is one of the main contributions to the desired tensile properties of nickel-based superalloys for turbine blades and disks. The value of SSS can be calculated by using Fleischer’s and Labusch’s theories, while the model parameters are incorporated without fitting to experimental data of complex alloys. In this work, four diffusion multiples consisting of multicomponent alloys and pure Ni are prepared and characterized. The composition and microhardness of single γ phase regions in samples are used to quantify the SSS. Then, Fleischer’s and Labusch’s theories are examined based on high-throughput experiments, respectively. The fitted solid solution coefficients are obtained based on Labusch’s theory and experimental data, indicating higher accuracy. Furthermore, six machine learning algorithms are established, providing a more accurate prediction compared with traditional physical models and fitted physical models. The results show that the coupling of high-throughput experiments and machine learning has great potential in the field of performance prediction and alloy design.Keywords
Cite This Article
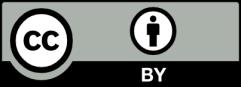
This work is licensed under a Creative Commons Attribution 4.0 International License , which permits unrestricted use, distribution, and reproduction in any medium, provided the original work is properly cited.