Open Access
ARTICLE
Machine Learning-Based Channel State Estimators for 5G Wireless Communication Systems
1
Department of Electrical Engineering, Faculty of Engineering, Al-Azhar University, Qena, 83513, Egypt
2
Department of Computer Engineering, College of Computers and Information Technology, Taif University, Taif, 21944,
Saudi Arabia
3
Department of Management Information Systems, Taif University, Taif, 21944, Saudi Arabia
* Corresponding Author: Mohamed Hassan Essai Ali. Email:
(This article belongs to the Special Issue: AI-Driven Engineering Applications)
Computer Modeling in Engineering & Sciences 2023, 135(1), 755-778. https://doi.org/10.32604/cmes.2022.022246
Received 28 February 2022; Accepted 27 May 2022; Issue published 29 September 2022
Abstract
For a 5G wireless communication system, a convolutional deep neural network (CNN) is employed to synthesize a robust channel state estimator (CSE). The proposed CSE extracts channel information from transmit-and-receive pairs through offline training to estimate the channel state information. Also, it utilizes pilots to offer more helpful information about the communication channel. The proposed CNN-CSE performance is compared with previously published results for Bidirectional/long short-term memory (BiLSTM/LSTM) NNs-based CSEs. The CNN-CSE achieves outstanding performance using sufficient pilots only and loses its functionality at limited pilots compared with BiLSTM and LSTM-based estimators. Using three different loss function-based classification layers and the Adam optimization algorithm, a comparative study was conducted to assess the performance of the presented DNNs-based CSEs. The BiLSTM-CSE outperforms LSTM, CNN, conventional least squares (LS), and minimum mean square error (MMSE) CSEs. In addition, the computational and learning time complexities for DNN-CSEs are provided. These estimators are promising for 5G and future communication systems because they can analyze large amounts of data, discover statistical dependencies, learn correlations between features, and generalize the gotten knowledge.Keywords
Cite This Article
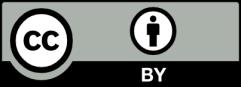