Open Access
ARTICLE
Ensemble Classifier Design Based on Perturbation Binary Salp Swarm Algorithm for Classification
1 School of Management, Hefei University of Technology, Hefei, 230009, China
2 School of Statistics, Zhejiang Gongshang University, Hangzhou, 310018, China
3 Key Laboratory of Process Optimization and Intelligent Decision-Making, Ministry of Education, Hefei, 230009, China
4 Collaborative Innovation Center for Statistical Data Engineering Technology and Application, Zhejiang Gongshang University, Hangzhou, 310018, China
* Corresponding Author: Qizhi He. Email:
(This article belongs to the Special Issue: Data-Driven Robust Group Decision-Making Optimization and Application)
Computer Modeling in Engineering & Sciences 2023, 135(1), 653-671. https://doi.org/10.32604/cmes.2022.022985
Received 03 April 2022; Accepted 17 May 2022; Issue published 29 September 2022
Abstract
Multiple classifier system exhibits strong classification capacity compared with single classifiers, but they require significant computational resources. Selective ensemble system aims to attain equivalent or better classification accuracy with fewer classifiers. However, current methods fail to identify precise solutions for constructing an ensemble classifier. In this study, we propose an ensemble classifier design technique based on the perturbation binary salp swarm algorithm (ECDPB). Considering that extreme learning machines (ELMs) have rapid learning rates and good generalization ability, they can serve as the basic classifier for creating multiple candidates while using fewer computational resources. Meanwhile, we introduce a combined diversity measure by taking the complementarity and accuracy of ELMs into account; it is used to identify the ELMs that have good diversity and low error. In addition, we propose an ECDPB with powerful optimizing ability; it is employed to find the optimal subset of ELMs. The selected ELMs can then be used to form an ensemble classifier. Experiments on 10 benchmark datasets have been conducted, and the results demonstrate that the proposed ECDPB delivers superior classification capacity when compared with alternative methods.Keywords
Cite This Article
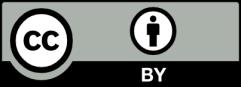
This work is licensed under a Creative Commons Attribution 4.0 International License , which permits unrestricted use, distribution, and reproduction in any medium, provided the original work is properly cited.