Open Access
ARTICLE
A Study of BERT-Based Classification Performance of Text-Based Health Counseling Data
Namseoul University, Cheonan, Chungcheongnam-do, Korea
* Corresponding Author: Cheong Ghil Kim. Email:
(This article belongs to the Special Issue: Computer Modeling of Artificial Intelligence and Medical Imaging)
Computer Modeling in Engineering & Sciences 2023, 135(1), 795-808. https://doi.org/10.32604/cmes.2022.022465
Received 10 March 2022; Accepted 24 May 2022; Issue published 29 September 2022
Abstract
The entry into a hyper-connected society increases the generalization of communication using SNS. Therefore, research to analyze big data accumulated in SNS and extract meaningful information is being conducted in various fields. In particular, with the recent development of Deep Learning, the performance is rapidly improving by applying it to the field of Natural Language Processing, which is a language understanding technology to obtain accurate contextual information. In this paper, when a chatbot system is applied to the healthcare domain for counseling about diseases, the performance of NLP integrated with machine learning for the accurate classification of medical subjects from text-based health counseling data becomes important. Among the various algorithms, the performance of Bidirectional Encoder Representations from Transformers was compared with other algorithms of CNN, RNN, LSTM, and GRU. For this purpose, the health counseling data of Naver Q&A service were crawled as a dataset. KoBERT was used to classify medical subjects according to symptoms and the accuracy of classification results was measured. The simulation results show that KoBERT model performed high performance by more than 5% and close to 18% as large as the smallest.Keywords
Cite This Article
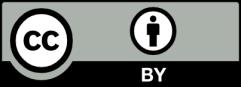
This work is licensed under a Creative Commons Attribution 4.0 International License , which permits unrestricted use, distribution, and reproduction in any medium, provided the original work is properly cited.