Open Access
REVIEW
Overview of 3D Human Pose Estimation
1
Huaiyin Institute of Technology, Huai’an, 223000, China
2
Jiangsu Outlook Shenzhou Big Data Technology Co., Ltd., Nanjing, 210002, China
3
Institute of Semiconductors, Chinese Academy of Sciences, Beijing, 100083, China
4
Center of Materials Science and Optoelectronics Engineering & School of Integrated Circuits, University of Chinese Academy of
Sciences, Beijing, 100049, China
5
Inspur Software Group Company, Ltd., Jinan, 250104, China
6
China Great Wall Industry Corporation, Beijing, 100054, China
7
China Great Wall Industry Corporation Navigation Co., Ltd., Beijing, 100144, China
* Corresponding Authors: Haifang Jian. Email: ; Gongming Wang. Email:
(This article belongs to the Special Issue: Enabled and Human-centric Computational Intelligence Solutions for Visual Understanding and Application)
Computer Modeling in Engineering & Sciences 2023, 134(3), 1621-1651. https://doi.org/10.32604/cmes.2022.020857
Received 16 December 2021; Accepted 26 April 2022; Issue published 20 September 2022
Abstract
3D human pose estimation is a major focus area in the field of computer vision, which plays an important role in practical applications. This article summarizes the framework and research progress related to the estimation of monocular RGB images and videos. An overall perspective of methods integrated with deep learning is introduced. Novel image-based and video-based inputs are proposed as the analysis framework. From this viewpoint, common problems are discussed. The diversity of human postures usually leads to problems such as occlusion and ambiguity, and the lack of training datasets often results in poor generalization ability of the model. Regression methods are crucial for solving such problems. Considering image-based input, the multi-view method is commonly used to solve occlusion problems. Here, the multi-view method is analyzed comprehensively. By referring to video-based input, the human prior knowledge of restricted motion is used to predict human postures. In addition, structural constraints are widely used as prior knowledge. Furthermore, weakly supervised learning methods are studied and discussed for these two types of inputs to improve the model generalization ability. The problem of insufficient training datasets must also be considered, especially because 3D datasets are usually biased and limited. Finally, emerging and popular datasets and evaluation indicators are discussed. The characteristics of the datasets and the relationships of the indicators are explained and highlighted. Thus, this article can be useful and instructive for researchers who are lacking in experience and find this field confusing. In addition, by providing an overview of 3D human pose estimation, this article sorts and refines recent studies on 3D human pose estimation. It describes kernel problems and common useful methods, and discusses the scope for further research.Keywords
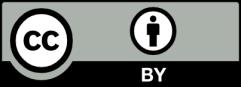