Open Access
ARTICLE
Novel Soft Computing Model for Predicting Blast-Induced Ground Vibration in Open-Pit Mines Based on the Bagging and Sibling of Extra Trees Models
1
Department of Surface Mining, Mining Faculty, Hanoi University of Mining and Geology, Hanoi, 100000, Vietnam
2
Innovations for Sustainable and Responsible Mining (ISRM) Research Group, Hanoi University of Mining and Geology,
Hanoi, 100000, Vietnam
* Corresponding Author: Quang-Hieu Tran. Email:
(This article belongs to the Special Issue: Computational Intelligent Systems for Solving Complex Engineering Problems: Principles and Applications)
Computer Modeling in Engineering & Sciences 2023, 134(3), 2227-2246. https://doi.org/10.32604/cmes.2022.021893
Received 11 February 2022; Accepted 22 April 2022; Issue published 20 September 2022
Abstract
This study considered and predicted blast-induced ground vibration (PPV) in open-pit mines using bagging and sibling techniques under the rigorous combination of machine learning algorithms. Accordingly, four machine learning algorithms, including support vector regression (SVR), extra trees (ExTree), K-nearest neighbors (KNN), and decision tree regression (DTR), were used as the base models for the purposes of combination and PPV initial prediction. The bagging regressor (BA) was then applied to combine these base models with the efforts of variance reduction, overfitting elimination, and generating more robust predictive models, abbreviated as BA-ExTree, BAKNN, BA-SVR, and BA-DTR. It is emphasized that the ExTree model has not been considered for predicting blastinduced ground vibration before, and the bagging of ExTree is an innovation aiming to improve the accuracy of the inherently ExTree model, as well. In addition, two empirical models (i.e., USBM and Ambraseys) were also treated and compared with the bagging models to gain a comprehensive assessment. With this aim, we collected 300 blasting events with different parameters at the Sin Quyen copper mine (Vietnam), and the produced PPV values were also measured. They were then compiled as the dataset to develop the PPV predictive models. The results revealed that the bagging models provided better performance than the empirical models, except for the BA-DTR model. Of those, the BA-ExTree is the best model with the highest accuracy (i.e., 88.8%). Whereas, the empirical models only provided the accuracy from 73.6%–76%. The details of comparisons and assessments were also presented in this study.Keywords
Cite This Article
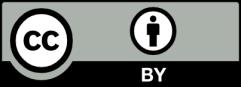
This work is licensed under a Creative Commons Attribution 4.0 International License , which permits unrestricted use, distribution, and reproduction in any medium, provided the original work is properly cited.