Open Access
ARTICLE
SFC Design and VNF Placement Based on Traffic Volume Scaling and VNF Dependency in 5G Networks
School of Information and Communication Engineering, University of Electronic Science and Technology of China, Chengdu, 611731, China
* Corresponding Author: Zhihao Zeng. Email:
(This article belongs to the Special Issue: Artificial Intelligence of Things (AIoT): Emerging Trends and Challenges)
Computer Modeling in Engineering & Sciences 2023, 134(3), 1791-1814. https://doi.org/10.32604/cmes.2022.021648
Received 26 January 2022; Accepted 22 April 2022; Issue published 20 September 2022
Abstract
The development of Fifth-Generation (5G) mobile communication technology has remarkably promoted the spread of the Internet of Things (IoT) applications. As a promising paradigm for IoT, edge computing can process the amount of data generated by mobile intelligent devices in less time response. Network Function Virtualization (NFV) that decouples network functions from dedicated hardware is an important architecture to implement edge computing, deploying heterogeneous Virtual Network Functions (VNF) (such as computer vision, natural language processing, intelligent control, etc.) on the edge service nodes. With the NFV MANO (Management and Orchestration) framework, a Service Function Chain (SFC) that contains a set of ordered VNFs can be constructed and placed in the network to offer a customized network service. However, the procedure of NFV orchestration faces a technical challenge in minimizing the network cost of VNF placement due to the complexity of the changing effect of traffic volume and the dependency on the VNF relationship. To this end, we jointly optimize SFC design and VNF placement to minimize resource cost while taking account of VNF dependency and traffic volume scaling. First, the problem is formulated as an Integer Linear Programming (ILP) model and proved NP-hard by reduction from Hamiltonian Cycle problem. Then we proposed an efficient heuristic algorithm called Traffic Aware and Interdependent VNF Placement (TAIVP) to solve the problem. Compared with the benchmark algorithms, emulation results show that our algorithm can reduce network cost by 10.2% and increase service request acceptance rate by 7.6% on average.Keywords
Cite This Article
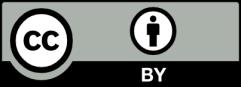