Open Access
ARTICLE
Change Point Detection for Process Data Analytics Applied to a Multiphase Flow Facility
1 Department of Mathematical Sciences, Chalmers University of Technology, SE-42196, Gothenburg, Sweden and ABB Corporate Research Centre, Ladenburg, Germany
2 Department of Mathematical Sciences, Chalmers University of Technology and University of Gothenburg, Gothenburg, Sweden
3 ABB Corporate Research Center, Ladenburg, Germany
* Corresponding Author: Rebecca Gedda. Email:
(This article belongs to the Special Issue: Advances on Modeling and State Estimation for Industrial Processes)
Computer Modeling in Engineering & Sciences 2023, 134(3), 1737-1759. https://doi.org/10.32604/cmes.2022.019764
Received 13 October 2021; Accepted 06 April 2022; Issue published 20 September 2022
Abstract
Change point detection becomes increasingly important because it can support data analysis by providing labels to the data in an unsupervised manner. In the context of process data analytics, change points in the time series of process variables may have an important indication about the process operation. For example, in a batch process, the change points can correspond to the operations and phases defined by the batch recipe. Hence identifying change points can assist labelling the time series data. Various unsupervised algorithms have been developed for change point detection, including the optimisation approach which minimises a cost function with certain penalties to search for the change points. The Bayesian approach is another, which uses Bayesian statistics to calculate the posterior probability of a specific sample being a change point. The paper investigates how the two approaches for change point detection can be applied to process data analytics. In addition, a new type of cost function using Tikhonov regularisation is proposed for the optimisation approach to reduce irrelevant change points caused by randomness in the data. The novelty lies in using regularisation-based cost functions to handle ill-posed problems of noisy data. The results demonstrate that change point detection is useful for process data analytics because change points can produce data segments corresponding to different operating modes or varying conditions, which will be useful for other machine learning tasks.Graphic Abstract
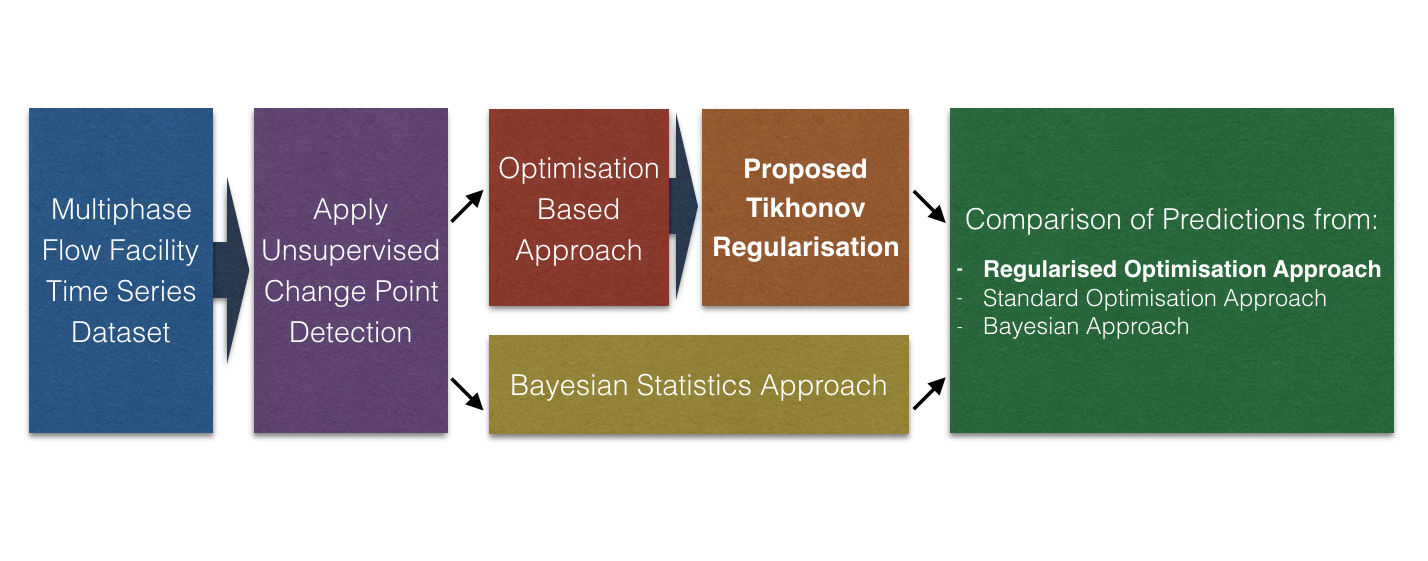
Keywords
Cite This Article
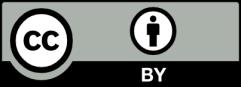
This work is licensed under a Creative Commons Attribution 4.0 International License , which permits unrestricted use, distribution, and reproduction in any medium, provided the original work is properly cited.