Open Access
ARTICLE
An Extended Fuzzy-DEMATEL System for Factor Analyses on Social Capital Selection in the Renovation of Old Residential Communities
1 School of Economics and Management, Liaoning University of Technology, Jinzhou, 121001, China
2 School of Information Management, Jiangxi University of Finance and Economics, Nanchang, 330032, China
3 Post-Doctoral Research Center, Zhongda Construction Co., Ltd., Guangzhou, 510280, China
4 School of Maritime Economics and Management, Dalian Maritime University, Dalian, 116026, China
* Corresponding Author: Shuping Wan. Email:
(This article belongs to the Special Issue: Extension, Modeling and Applications of Fuzzy Set Theory in Engineering and Science)
Computer Modeling in Engineering & Sciences 2023, 134(2), 1041-1067. https://doi.org/10.32604/cmes.2022.021981
Received 16 February 2022; Accepted 13 April 2022; Issue published 31 August 2022
Abstract
China has been promoting the renovation of old residential communities vigorously. Due to the financial pressure of the government and the sustainability of the renovation of old residential communities, public-private partnerships (PPP) have already gained attention. The selection of social capital is key to improving the efficiency of the PPP model in renovating old residential communities. In order to determine the influencing factors of social capital selection in the renovation of old residential communities, this paper aims to find an effective approach and analyze these factors. In this paper, a fuzzy decision-making and trial evaluation laboratory (fuzzy-DEMATEL) technique is extended and a more suitable system is developed for the selection of social capital using the existing group decision-making theory. In the first stage, grounded theory is used to extract the unabridged key influencing factors for social capital selection in the renovation of old residential communities. Secondly, by considering the impact of expert weights, the key influencing factors are identified. The interactions within these influencing factors are discussed and the credibility of the results is verified by sensitivity analysis. Finally, these key influencing factors are sorted by importance. Based on the results, the government should focus on a technical level, organizational management abilities, corporate reputation, credit status, etc. This study provides the government with a theoretical basis and a methodology for evaluating social capital selection.Keywords
China faces the challenge of sustainable urban development. Since the Chinese government implemented its reform and opening-up policies, people’s living standards have dramatically improved. Unfortunately, older residential communities built before 2000 have poor living conditions such as damaged roads, dilapidated pipelines, and a lack of public places. Thus, renovating old residential communities is considered to be a reasonable method of meeting people’s demand for a comfortable living environment [1]. As part of its efforts to promote the renovation and reconstruction of old residential communities, the Chinese government identified the importance of “building a spatial planning system” in 2015. In 2019, the Chinese government report stated the need to transform and upgrade the old urban residential areas, update the supporting facilities such as water, electricity, and gas, support elevator installation and improve living service facilities such as convenience squares, convenience stores, pedestrian streets, parking lots, and barrier-free passageways. China has since then entered a phase of comprehensively accelerating the renovation of old residential communities and listed a number of old residential community renovation projects. In China, based on data analysis from the National Bureau of Statistics and the Ministry of Housing and Urban-Rural Development, 51,200 old residential community renovation projects actually started in the first three quarters of 2021, accounting for 93% of all planned projects. In 2022, the institutional framework, policy system, and working mechanisms of the old urban residential community renovation will take shape. Depending on the actual situation in various regions, the renovation of old residential communities built before the end of 2000 will be completed by 2025 [2].
Social capital is destined to enter the field of old residential community renovation. In the process of renovating residential communities, high capital investment and a long life-cycle are associated with high risks [3]. Since the number of old residential community renovation projects has significantly increased, relying solely on government funds is no longer sufficient. Insufficient capital investment is a major obstacle to the renovation of old residential communities due to the high financial pressure on the local government. Adopting the PPP (public-private partnership) model would solve this problem as well as improve the efficiency of public product supply, share risks and make local governments more financially stable.
The PPP model is helpful to the strategy of sustainable development [4]. The selection of social capital is an integral part of any PPP project, which is included in the scope of government procurement management in China. Project procurement under the PPP model, however, differs from traditional government procurement in that the inquiry is canceled and the competition is increased [5]. As a result, the process of selecting social capital is more rigorous and more complex. This makes it necessary to build a reasonable evaluation index system based on the influencing factors of social capital selection in PPP projects.
Since social capital plays a different role in different projects, the influencing factors of social capital selection should also differ. As a new construction model emerging in China in recent years, the old residential community renovation project differs from previous reconstructions of old cities. Therefore, there are gaps in the existing research results on the social capital selection of old residential community renovation projects in both theory and practice. The novelty of this paper lies in the fact that the research system developed is a useful tool for analyzing the factors influencing social capital selection in the old residential community renovation project, which supports the successful application of the PPP model in the old residential community renovation project. The main contributions of this paper are as follows. Firstly, the evaluation system of factors influencing the selection of PPP projects in the renovation of old residential communities was extended. Secondly, the influencing factors of the old residential community renovation in China were successfully screened out, so the primary problem of social capital selection now resolved. Thirdly, the effectiveness of influencing factors was verified, and the selection process of social capital was optimized. Through the research, the government is provided with a theoretical basis and reference path to choose the social capital of the old residential community renovation project more scientifically and effectively.
The remainder of this paper is organized as follows. Section 2 discusses the process and influences of the old residential community renovation, identifies deficiencies in the existing studies, and explains the purpose of the study. In Section 3, the framework of social capital selection is presented for an old residential community renovation project. In Section 4, we present an extended fuzzy-DEMATEL system for determining the influencing factors of social capital selection in the renovation of old residential communities. In Section 5, examines the influencing factors of social capital selection in the renovation of old residential communities using the integrated system proposed. In Section 6, we compare the results of our research with the traditional fuzzy-DEMATEL approach and other approaches. Our conclusions and limitations are discussed in Section 7.
Urban renewal is the subject of many international studies. As a part of urban renewal, renovating old residential communities can also learn from research on urban renewal. As a result of the renovation of old communities, residents of the neighborhoods can improve their living environment, social connectedness, sense of community, and economic conditions, and improve their health and well-being [6]. Cai et al. [7] earlier elaborated the main strategies of “micro-transformation” of old residential communities from the perspectives of ideas, methods and mechanisms, which provided a reference for many researchers. Zheng et al. [8] analyzed urban renewal and sustainable development, improved the evaluation of urban renewal to a more comprehensive perspective, and analyzed the process of sustainable urban renewal from the functions of different planning elements and the roles and relationships of different stakeholders. The direction of the renovation of old residential communities has been of more importance than the cost and progress of the project [9]. Walford et al. [10] believed that urban renewal should consider architectural environment design effects on the elderly, and it should be suitable for the elderly and focus on their mental health. Charlotte et al. [11] studied the impact of public space reconstruction in poverty-stricken areas on people’s positive and optimistic attitudes towards life and provided useful references and valuable insights for future sustainable urban renewal. Marta et al. [12] analyzed from the perspective of the use vision of the land-use sectors, aiming to realize the sustainable use of land in the process of urban renewal from the three purposes of Best Land, Regional Connected and Local Multifunctional. Arslan et al. [13] studied the relationship between built environment design and social sustainable renewal in the process of urban renewal, evaluates built environment design from five aspects: accessibility and quality of social life, conservation of resources, quality of the built environment, protection of disadvantaged groups, and commercial and economic opportunities. By studying the public engagement process, financial well-being, and community cohesion and stability, Trop [14] evaluated the social impact of property demolitions and construction projects, as well as methods to improve the results of all parties affected, emphasizing that local government plays an important role in this process. Li et al. [15] constructed a benefit-evaluation model to assess the benefits of transforming and expanding old green villages, which demonstrated that the green transformation offers more benefits than the traditional transformation. Yang et al. [16] explored the factors affecting residential satisfaction of poor spaces in new cities from the perspectives of individual characteristics of residents, residential and neighborhood environment, etc., and made recommendations for innovative management technologies, improvements in living conditions, and the construction of related support facilities. Based on the living experience and social cognitive, Guo et al. [17] and Huang et al. [18] also put forward a sustainable renovation strategy for old residential communities in practice. Despite the fact that the above-mentioned literature analysis on the old residential community renovation did not identify any specific influencing factors, it can offer a direction for experts to understand the old residential community renovation and further analyze the influencing factors of social capital selection.
Researchers have studied the factors that influence the implementation of the old residential community renovation project, which are also important when choosing social capital. Cai et al. [19] examined the influencing factors of the external environment, the project’s own scheme, contracts, construction, capital, and other factors, as well as the influencing factors of project stakeholders. Moreover, the influencing mechanisms between various influence factors are revealed, and major policy recommendations are offered. Zhong et al. [20] examined the cost management difficulties of old residential community renovation projects in light of a series of phenomena such as unreasonable construction organization designs and low quality of construction personnel, thus leading to non-standard construction processes, etc., and provides solutions for each of them. Palumbo et al. [21] looked at the economic, social, and environmental aspects of social and environmental regeneration programs in poor communities with low housing quality in Europe for achieving low-carbon transformation through strategies like renewable energy production, smart mobility, water management, waste management, civic participation, and civic engagement. Randall et al. [22] complemented the indicators to evaluate the progress of sustainable urban development, adding vacancies rate, business composition, and turnover, along with existing measures to measure sustainable urban renewal of land-use diversity.
It is an important direction for social capital to participate in the renovation of old residential communities. Shen et al. [23] developed a model of urban regeneration engine that promoted the participation of governments, enterprises, residents, social organizations, and financial institutions. Zhang et al. [24] and Ouenniche et al. [25] noted that it is crucial to choose the right partner and determine the valuation criteria. From the perspective of construction enterprises, Du et al. [26] examined the requirements for standing out from the crowd in the selection of social capital. Li et al. [27] investigated different ways of choosing the best social capital partner under three different payment modes: government payment, user payment, and feasibility gap subsidy, and created an evaluation model to evaluate the impact of social capital partner selection on the effectiveness of government-enterprise cooperation from the perspectives of social welfare maximization and operational efficiency. Zhou et al. [28] applied factor analysis to extract the key variables for making decisions on urban renewal and analyzed the divergent opinions of various stakeholders so as to obtain the consensus opinions on achieving sustainability in urban development. Wang et al. [29] applied the entropy method and fuzzy theory to the indicator system established by factor analysis theory, and calculated the comprehensive benefits of the three core stakeholders, which include government, developers, and residents. Taking the United States, Britain, and Japan as examples, Ren et al. [30] suggested the formation of a formal cooperation model between the government and social capital in the investment and financing of urban renewal, and the active enlistment of social capital. Geng et al. [31] provided a social capital selection framework for electrochemical storage power stations, which had implications for other types of projects involving social capital selection. As a method of systematic factor analysis, fuzzy DEMATEL has been successfully applied to supply chain risk management [32,33], solid waste disposal [34], disaster assessment [35], weapon systems selection [36], etc.
Most existing studies on the renovation of old residential communities focus on the content and direction of the renovation, while very few are concerned with the remodeling model. Renovating old residential communities is in its early stages, and the PPP model is an important development path. Having a suitable social capital can prevent the slow renovation process in the future, from affecting the operational efficiency of the project. An appropriate partner has a greater impact on the efficiency of PPP projects. Therefore, choosing the right partner is a particularly important issue. In contrast, the studies concerning social capital selection are mostly qualitative in nature with few quantitative studies, and the mechanics of the interaction between the factors are not thoroughly explored. Multiple stakeholders are involved in the renovation of old residential communities, and a unilateral evaluation from the perspective of the government or owners will lead to a distorted evaluation. An empirical study identifying the influence factors of social capital selection in the renovation of old residential communities is lacking. Theoretically, if we are to make significant progress on sustainable urban renewal in China, we must first identify the critical influencing factors and investigate their influencing mechanisms. It is the purpose of this paper to describe how the combination of qualitative research and Fuzzy-DEMATEL can be used as a valuable managerial tool to suggest decisions regarding the government’s partner selection process and to suggest how the social capital can improve its competitiveness.
3 Framework of Social Capital Selection System
The research of decision-making analysis mainly focuses on two directions: First, the behavior mechanism of the decision-making process is discussed theoretically. The other is to simulate the actual decision-making process. The selection of social capital for PPP projects can be viewed as a classic multi-attribute group decision-making problem [37]. Sun et al. [38] have proposed the framework of the social capital selection system, which is used for the renovation of old residential communities as Fig. 1. In Fig. 1, the grey block shows influencing factors of social capital selection and the importance can be found. An extended Fuzzy-DEMATEL system for analyzing influencing factors of social capital selection in the renovation of old residential communities is proposed and applied in this paper.
Figure 1: Framework of social capital selection system
Establishing an effective system with the key influencing factors is crucial to the selection of social capital. In some research articles, the fuzzy-DEMATEL method was successfully used to analyze the influencing factors [39–41]. The fuzzy-DEMATEL approach helps determine the importance of influencing factors more accurately and ensures the stability of the data obtained from qualitative and quantitative analysis. A fuzzy-DEMATEL approach can not only build the relationship and structure of influencing factors but also solve the evaluation problem in complex systems under uncertain circumstances. The fuzzy-DEMATEL approach is used in this study. Nevertheless, the fuzzy-DEMATEL approach is not completely applicable to the PPP projects of the old residential community renovation. In recent years, the old residential community renovation project has just arisen in China, and many researchers and engineers do not have enough background knowledge about it, which may lead to incomplete screenings and inaccurate evaluations of social capital selection factors. From this perspective, this study has made innovations.
In this paper, the fuzzy-DEMATEL method is extended to an integrated system for determining the influencing factors of social capital selection in the renovation of old residential communities.
4.1 Limitations Analysis of the Traditional Fuzzy-DEMATEL Approach
In the case of PPP projects for the renovation of old residential communities, the traditional fuzzy-DEMATEL approach has two limitations:
(1) The old model of community renovation is constantly being improved, which is more conducive to the happiness of the residents and economic development. There are many factors affecting the old residential community renovation project, but the traditional approach does not consider the scientific validity and integrity of the index source, which leads to an incomplete evaluation.
(2) In China, the old residential community renovation model is in the exploratory stage, and experts in different fields have perspectives on it. Using the traditional method is applied to select social capital for an old residential community renovation project, ignores expert weight and a causal relationship among influencing factors, which weakens the rigor of the selection of some extent.
4.2 Establishment and Extension of Influencing Factors Evaluation System
The purpose of this study is to discover the factors that influence the social capital selection and their relationship. In order to analyze the influencing factors, there are three steps, as shown in Fig. 2. A conceptual framework is developed for determining the influencing factors of social capital selection. First, based on programming grounded theory, the main influencing factors are determined, and the initial list is modified and adjusted through expert interviews to determine the final influencing factors, as well as taking into consideration the practice of old residential community renovation at the same time in this step. In addition, expert scoring is used to determine the interactions among the influencing factors. In this step, the fuzzy language scale is used to establish the qualitative relationship, and DEMATEL is used to analyze the causal relationship among influencing factors. As a third step, the causality analysis and sensitivity analysis is used to identify the influencing mechanism between various influencing factors, and the main policy suggestions are provided. The specific steps are listed in Fig. 2.
Figure 2: Establishment of influencing factors evaluation system
Developed by Glaser et al. in 1967, grounded theory is a qualitative research method of bottom-up analysis [42]. Research materials are obtained through literature reviews or interviews, which are then analyzed and summarized to form the final research framework. However, the grounded theory continues to be popular today [43]. Prior to starting a study, researchers do not set any preconditions or assumptions. The only things they pay attention to are realistic problems. That means that they observe the actual situation of the real world, and explore the research field with an open mind. Existing grounded theory can be categorized into three groups according to its epistemology, namely classical grounded theory, programmed grounded theory, and constructivist grounded theory, with slightly different coding sequences and procedures [44]. In qualitative research, programmed grounded theory which can explore the causal relationship between variables is used in this paper, and the grounded theory operating program is shown in Fig. 3. A data processing and theory construction program consists of three steps: open coding, axial coding, and selective coding.
Figure 3: Grounded theory operating program
(1) Open coding
Open coding is a process of conceptualizing and abstracting information data by analyzing and summarizing interview materials and information from the literature, sentence by sentence, paragraph by paragraph, and gradually arriving at the concepts and categories of interest [45].
NVivo 11 Plus is used to query word frequency and encode sorted papers further to view the most frequently occurring words. after reading, analyzing, and summarizing dozens of papers about factors affecting social capital selection. In the next step of coding, the words with the highest frequency will be used.
Using NVivo software for open coding, we separated the parts related to influencing factors in the paper into multiple texts, coded them word-by-word, sentence-by-sentence, then abstracted and defined each text as a node. Once all texts have been defined, the defined part will gradually be structured, and similar definitions will be merged together to form new nodes. Open coding results in the three-level indicator of the indicator evaluation system.
(2) Axial coding
Following the open coding, we performed a comparative analysis of the nodes summarized in the previous step to determine whether there is a correlation between the relevant nodes, refine and adjust the nodes, and classify all the nodes to complete the axial coding process. It is the process of studying the category and dimension of indicators, and the process of analyzing the category and dimension is also the process of theoretical construction [46]. The nodes are combined into secondary indicators such as corporate reputation, credit status, profitability, and degree of satisfaction with similar projects. As the evaluation indicators are improved, a greater theoretical sensitivity is developed, and an increased understanding of factors affecting the selection of social capital is gained.
(3) Selective coding
In order to analyze and classify the indicators obtained from axial coding, their internal relationships are analyzed. This is the process of selecting core categories, systematically associating them with other categories, validating those associations, and populating categories that need further refinement and development [44]. The induction summary indicators are based on the social benefits, technological level, corporate reputation and credit status, corporate financial capability, ability to organize and manage, corporate performance status and risk management capabilities of seven first-level indicators. This article, which includes information on the factors that influence the selection of social capital based on the perspective of corporate production and management, social responsibility and a range of qualitative and quantitative metrics, summarizes the factors that influence the selection of social capital.
(4) Theoretical saturation test
Three experts conducted semi-structured interviews and the interview content was analyzed through open coding, axial coding, and selective coding to verify the accuracy and authenticity of the indicator system [47]. New nodes do not appear in the results. Thus, we consider the above influencing factor model of social capital selection to be theoretically saturated.
(1) Determination of expert weight
To make the results of this study more realistic, the influence of expert weight is included in the extended Fuzzy-DEMATEL system. Many studies have been conducted on judging the weight of experts. CesaBianchi et al. [48] weighted experts based on the number of mistakes they have made in the past. Aly et al. [49] examined a method for determining the weights of a group of expert decision makers by evaluating their knowledge, relevance, and experience. Liu et al. [50] proposed a two-layer weight determination model for obtaining expert weights in complex multi-attribute large-group decision-making problems. Wan et al. [51] built a fractional programming model that can be transformed into a linear program and used to find the expert weights. Li et al. [52] developed two models for calculating experts’ weights using the cross-entropy index, etc. As a result of the above-mentioned particularity of social capital selection in PPP projects in the bidding process, and due to the complexity of the old residential community renovation projects, experts are vital in analyzing the influence factors of social capital selection. Therefore, this study recommends using subjective and objective comprehensive weighting methods to determine expert weight. Chi et al. [53] examined optimal combinations of weights, but the methods of objective weights are unsuitable for selecting social capital in the renovation of old residential communities. Therefore, a simple and feasible method for combining the subjective and objective weight is proposed.
Firstly, experts’ objective weight should be determined. The entropy weight method is an objective method of assigning weights based on the concept of information entropy, which measures the variation degree of each index value [54]. The higher the information entropy value, the less weight the expert’s opinion is given to the final result, and the more inaccurate the information is provided. It is possible to avoid the interference of subjective factors by calculating information entropy, intuitively reflecting the fluctuation of expert evaluation data, and decreasing the impact of expert opinions with large differences on the evaluation objective. The objective weight of each expert is
Secondly, experts’ subjective weight should be determined. By using categorically based evaluation technique (MACBETH), it is possible to translate decision-makers’ subjective preferences into mathematical weights, so that each expert’s weight can be determined [55]. For m experts
where, the optimal solution of the integer linear programming is the MACBETH weight
Finally, the comprehensive weight is determined by combination weight. The subjective weighting method can represent the decision-maker’s intention to each expert, which can be significant, but it is often unable to fully comprehend all the characteristics of each expert. In some instances, the entropy method based on data analysis can eliminate interference from expert experience and risk-facing ability to human subjective factors. If the preference of decision-makers regarding the indicators of experts are not taken into account, experts with little influence on the evaluation results will be given a higher weight. Therefore, objective and subjective weights should be considered comprehensively to determine the expert weight W of a comprehensive evaluation, which can be calculated by Eq. (5).
(2) Analysis of influencing factors
Triangular fuzzy numbers are a branch of fuzzy mathematics which plays a vital role in the process of making uncertain decisions and can very effectively deal with the phenomenon that experts’ cognition of objective things is affected by uncertainty and fuzzy decisions.
Firstly, the interaction between influencing factors is determined with fuzzy numbers. Experts from different industries were asked to score the relationship between the influencing factors of social capital selection in the renovation of old residential communities under the PPP model. As a result of vagueness of the human perceptions, the fuzzy language operator method is used to evaluate the relationship between influencing factors, with five levels of language expression: very high (VH), high (H), moderate (M), low (L), and no effect (N) [56], language operator and the corresponding triangular fuzzy Numbers are shown in Table 1.
The triangular fuzzy number is
Then, in order to standardize experts’ opinions, triangular fuzzy numbers are standardized through Eqs. (6)−(9) [57].
Then, by using Eqs. (10)−(13), the fuzzy data are converted into crisp score, so as to obtain the interaction between the influencing factors [58].
Then, Eq. (14) is used to combine the opinions of different experts in order to obtain the direct interaction between the influencing factors.
where
So as to get the initial direct interaction matrix Z.
Then, Eq. (15) is used to normalize direct interaction matrix
Then, the total relation matrix T is calculated by Eq. (16) to obtain the comprehensive interaction among the influencing factors.
Then, causality analysis, by calculating the sum of each row and column in the total relationship matrix by Eqs. (17) and (18), obtains the degree of direct influence
Then, the cause-effect relationship diagram is established by calculating and, with (
In the end, a scatter chart is drawn using importance index and reason index to analyze the importance of each influencing factor [59].
Interview materials and published core literature comprise most of the research materials. China National Knowledge Infrastructure (CNKI) database and Web of Science (WOS) database both use “PPP social capital selection” as the key word or the title of the literature contains “social capital selection” or “PPP mode”. The literature is encoded using NVivo 11 software.
As shown in Table 2, the factors affecting the selection of social capital can be obtained by the grounded theory analysis process of open coding, axial coding, selective coding and theoretical saturation.
5.2 Analysis of Influencing Factors
In this study, three experts who have participated in the research and practice of the renovation of old residential communities, respectively from universities, governments and enterprises, were invited. The weight of experts is determined by combining subjective and objective information. Experts used the triangular fuzzy language terms to evaluate and obtain the fuzzy evaluation matrix based on the relative importance of each indicator. In Table 3, the fuzzy matrix is standardized by Eqs. (6)−(9); the fuzzification process is standardized by Eqs. (10)−(13); the initial direct interaction matrix Z is calculated by Eq. (14). Using Eqs. (15) and (16), the total relation matrix T in Table 4 is calculated. The importance index and the cause index are then obtained in Table 5.
According to the data obtained in Table 5, the importance index (
Figure 4: Scatter chart for importance and cause index
By combining Fig. 4 and Table 5, we can analyze the influencing factors and discuss each factor’s impact on the entire system, thus indicating the critical factors in selecting social capital. The abundance of professional and technical personnel (T5) is the highest in the calculated importance index (
Generally, the mean value of importance indicators for technological level (5.5124) is the highest, followed by the ability to organize and manage, corporate reputation, and credit status of 4.8910 and 4.8744, respectively. Therefore, when evaluating social capital, we should pay closer attention to the technological level of social capital and set a higher weight level. The main purpose of social capital is to increase investment in technology innovation, improve organizational, management, and resource integration capabilities, maintain corporate reputation and commercial credit, and provide reference for enterprise future development.
Among the 16 factors with positive cause index (
There is a negative cause index for 19 influencing factors, and these factors are greatly affected by other influencing factors, which can be considered efficient influencing factors. Relief of financial pressures has the lowest causality influencing factor (S1 = −1.4458). Second, owner satisfaction (P3 = −1.3406), financing ability (F3 = −0.9029), willingness to tolerate risks (R4 = −0.6917), degree of willingness to cooperate (P2 = −0.6886), evaluation of project performance (P4 = −0.6522), etc. The influence of these factors on other factors is less than that of other factors on itself. Since influencing factors are influenced by causal factors, causal factors should be the focus of social capital evaluation or improvement. In light of the interdependence of several factors, attention should be paid to the influential factor indicators with large causative index values, to ensure that the evaluation results of social capital selection are more reasonable and perfect.
6.2 Sensitivity Analysis of Results
The purpose of sensitivity analysis is to identify the degree to which the weight of the decision made by the evaluation expert affects the final result, to judge the reliability of the data analysis, and to understand the impact of different weighting combinations of decision evaluators (keeping the weights equal then adjusting more weight to each evaluator).
First, equal weights are assigned to each evaluator, and then the weight of each evaluator is changed according to the working years and job responsibilities of each expert to analyze how much the cause-effect relations vary. In view of the fact that the second and third experts have a longer working life, are faced with greater risks, and have more experience in the selection process of social capital, their weight values are changed equally in the sensitivity analysis. Scenarios based on evaluators’ different weight combinations are given in Table 6.
Through the analysis of Fig. 5 and Table 7, we can see that the causal factors in each of the six scenarios remain roughly the same, proving their reliability and validity. Although, on the whole, our research results are in line with the sensitivity analysis process, as shown in Fig. 5 that the importance index does not completely coincide with the cause index under different weights, and we believe that different expert weights have an impact on it. According to the importance index, the relationship between this influencing factor and other influencing factors is close. Under different expert weight combinations, the range of fluctuations varies, but the magnitude of fluctuations remains unchanged. Consequently, we believe that each expert has a different view regarding the tightness of the connection between indicators, so the importance index values obtained under different expert weights are different, but the relative tightness between each indicator remains the same, so we believe that this study meets the requirements.
Figure 5: Causal diagram of sensitivity analysis
6.3 Further Discussions and Comparisons
This paper compares an extended fuzzy-DEMATEL system with the traditional fuzzy-DEMATEL approach [40,41,59], and has the following advantages:
(1) For influencing factors of social capital selection in the renovation of old residential communities, the extended fuzzy-DEMATEL system provides a more comprehensive range of influencing factors, and it is a more scientific way to obtain it.
(2) This paper examines the influence of expert weight. Using the more appropriate method of determining weight, the determination of influencing factors is more accurate, and the causal relationship among influencing factors is more accurate.
(3) The research findings complement the deficiency of influencing factors of social capital selection in the renovation of old residential communities in existing research.
Furthermore, it is also compared with the technique for order of preference by similarity to the ideal solution (TOPSIS) and the system dynamics method (SD). Compared with the subjective definition of positive and negative ideal points in the TOPSIS method [60], the extended fuzzy-DEMATEL system can better reflect the effect of influencing factors and provide more complete, accurate, and reliable results. Compared with the SD method [61], which uses causality diagram and inventory flow table to simulate and analyze the importance of each index qualitatively and quantitatively, the extended fuzzy-DEMATEL system can determine the priority and importance of influencing factors by cause index and importance index, which is simple and efficient. In this study, the extended fuzzy-DEMATEL system adopted the subjective and objective weighting method to improve the use of qualitative research methods, rather than defined weights directly in other studies [60,61]. The influencing factors were directly screened [60,61], which is not enough to prove the rationality and completeness of the established index system. The application of grounded theory to the extended fuzzy-DEMATEL system fills this research void.
In this study, we construct an extended fuzzy-DEMATEL system and analyze the influencing factors of social capital selection, which can provide guidance for the selection of social capital in the old residential community renovation project. The trend of urban renewal and sustainable development is for social capital to participate in the renovation of old residential communities. For the scientific choice of social capital, the main task is to study the influencing factors. An extended fuzzy-DEMATEL system is used in this paper to analyze influencing factors in social capital selection in renovation of old residential communities. The system combines the grounded theory with the triangular fuzzy DEMATEL method to form an integrated system. Using the extended fuzzy-DEMATEL system, those important indicators influencing the selection of social capital in the old residential community renovation project can be identified, which can assist government decision-makers to identify the appropriate social capital using a scientific and reasonable method and improve government procurement efficiency. The grounded theory ensures the integrity of influencing factors without omission, the triangular fuzzy theory can establish the qualification of the relationship, and the DEMATEL method is used to analyze the causal relationship among the influencing factors. Compared to the other methods compared in this paper, the extended fuzzy-DEMATEL system has a more complete index system, a clearer causal relationship between various influencing factors, and a better ability to handle vague and inaccurate information. In addition, sensitivity analysis is introduced to verify the research results and demonstrate their effectiveness.
In the renovation of old residential communities, the causality analysis of influencing factors of social capital selection shows that the evaluation process of social capital selection should concentrate on the analysis of technological level, ability to organize and manage, corporate reputation and credit status, among other factors. A number of influencing factors with causal relationships exist, including historical project honors (C3), technological innovation level (T2), core technology strength and methods (T4), R&D investment intensity (T1), completeness of mechanical equipment (T3), the abundance of professional and technical personnel (T5), Financing ability (F3).
The results of this study can be used by government decision-makers to evaluate the factors influencing the social capital selection and improve work efficiency using scientific evaluation methods. For social capital managers, the causal relationship of influential factors can also help them focus on causal factors and improve their own advantages. As part of the future research process, we can invite more experts to participate in the evaluation process and improve its reliability.
7.2 Limitations and Reflections
There are some limitations to this study. From the research methods and content, the following will be discussed. This paper proposes an extended fuzzy-DEMATEL system, which improves the traditional fuzzy-DEMATEL method. However, the old residential community renovation is an emerging construction model in China, and society has a weak systematic understanding of PPP model for old residential community renovation projects, which has inevitably led to subjective bias. This results in the weighting of experts and the completeness of indicators at the expense of in-depth understanding. Despite the fact that this study has made some contributions to this perspective, it is preferable to conduct more in-depth theoretical research. In future studies, more methods for determining expert weight can be applied to different types of projects. In this way, a more accurate expert weight relationship can be obtained and a more efficient means of engineering construction can be provided. In addition, experts are faced with complex systems, and the incomplete knowledge structure may influence the research results. This is also a disadvantage of fuzzy-DEMATEL method. To determine the influencing factors of expert weight in old residential community renovation projects, more empirical research is required. Another limitation is that the old residential community renovation is a new construction model in China in recent years, which may have affected the research contents. Because of this, when applying grounded theory to qualitative analysis, we have comprehensively considered the existing literature and the analysis results of experts in various fields. Nevertheless, with the advancement of urban renewal in China, new models and factors will emerge one after another, and the influencing factors and their degrees of influence presented in this paper may change.
In spite of these limitations, we believe that we have developed an interesting subject in the literature of old residential community renovation, which has never been fully explored. Research in the future will be interesting if the influencing factors of social capital selection in the old residential community renovation project can be studied qualitatively and quantitatively. Additionally, future research can also verify and develop the results of this study from the perspectives of research methods and research content. The Pythagorean fuzzy number, for example, does not set the value of the variables [62], which expands the membership space and can make up for the deficiency of complex indicators. It can be used to verify whether similar conclusions can be derived from those studies. The existing research results can also be made more practical based on the evolution of the public-private partnership model of old residential community renovations as well as advancements in experts’ understanding of such projects.
Acknowledgement: Thanks to the editors of this journal and the anonymous reviewer.
Funding Statement: This paper is supported by the National Natural Science Foundation of China (No. 62141302), the Humanities Social Science Programming Project of the Ministry of Education of China (No. 20YJA630059), the Natural Science Foundation of Jiangxi Province of China (No. 20212BAB201011), the China Postdoctoral Science Foundation (Grant No. 2019M662265) and the Research Project of Economic and Social Development in Liaoning Province (Grant No. 2022lslybkt-053).
Conflicts of Interest: The authors declare that they have no conflicts of interest to report regarding the present study.
References
1. Guan, H. Y., Zhu, X. C., Liu, Y. J. (2015). A study of individual rule-identity and strategy-expectation in the transition of governing the commons-based on the survey of residential community elevator replacement in nanjing. Management Review, 27(8), 13–22 (in Chinese). [Google Scholar]
2. General Office of The State Council of the People’s Republic of China (2020). Guiding opinions on comprehensively promoting the renovation of old residential areas in cities and towns (in Chinese). [Google Scholar]
3. Akintoye, A., Taylor, C., Fitzgerald, E. (1998). Risk analysis and management of private finance initiative projects. Engineering, Construction & Architectural Management, 5(1), 9–21. DOI 10.1108/eb021056. [Google Scholar] [CrossRef]
4. Feng, J., Wang, N., Sun, G. (2022). Measurement of innovation-driven development performance of large-scale environmental protection enterprises investing in public-private partnership projects based on the hybrid method. Sustainability, 14, 5096. DOI 10.3390/su14095096. [Google Scholar] [CrossRef]
5. Ministry of Finance of the People’s Republic of China (2014). Measures for the administration of government procurement for public-private partnership ([2014] No. 215) (in Chinese). [Google Scholar]
6. Jalaludin, B., Maxwell, M., Saddik, B., Lobb, E., Byun, R. et al. (2012). A Pre-and-post study of an urban renewal program in a socially disadvantaged neighbourhood in Sydney, Australia. BMC Public Health, 12(1), 521. DOI 10.1186/1471-2458-12-521. [Google Scholar] [CrossRef]
7. Cai, Y. N., Yang, X. J., Li, D. L. (2017). Micro-transformation: The renewal method of old urban community. Urban Development Studies, 24(4), 29–34 (in Chinese). [Google Scholar]
8. Zheng, H. W., Shen, G. Q., Hao, W. (2014). A review of recent studies on sustainable urban renewal. Habitat International, 41(1), 272–279. DOI 10.1016/j.habitatint.2013.08.006. [Google Scholar] [CrossRef]
9. Song, F. X., Kang, S. Y. (2020). The predicament and path of old residential district reconstruction under the background of aging population. Hebei Academic Journal, 40(5), 191–197 (in Chinese). [Google Scholar]
10. Walford, N., Phillips, J., Hockey, A., Pratt, S. (2017). Assessing the needs of older people in urban settings: Integration of emotive, physiological and built environment data. Geo: Geography and Environment, 4(1), e0037. DOI 10.1002/geo2.37. [Google Scholar] [CrossRef]
11. Pawlowski, C. S., Winge, L., Carroll, S., Schmidt, T., Wagner, A. M. et al. (2017). Move the neighbourhood: Study design of a community-based participatory public open space intervention in a danish deprived neighbourhood to promote active living. BMC Public Health, 17, 481. DOI 10.1186/s12889-017-4423-4. [Google Scholar] [CrossRef]
12. Pérez-Soba, M., Paterson, J., Metzger, M. J., Gramberger, M., Houtkamp, J. et al. (2018). Sketching sustainable land use in Europe by 2040: A multi-stakeholder participatory approach to elicit cross-sectoral visions. Regional Environmental Change, 18(3), 775–787. DOI 10.1007/s10113-018-1297-7. [Google Scholar] [CrossRef]
13. Yildiz, S., Kivrak, S., Gultekin, A. B., Arslan, G. (2020). Built environment design-social sustainability relation in urban renewal. Sustainable Cities and Society, 60, 102173. DOI 10.1016/j.scs.2020.102173. [Google Scholar] [CrossRef]
14. Trop, T. (2017). Social impact assessment of rebuilding an urban neighborhood: A case study of a demolition and reconstruction project in Petah Tikva, Israel. Sustainability, 9(6), 1076. DOI 10.3390/su9061076. [Google Scholar] [CrossRef]
15. Li, L. Y., Gu, H. (2021). Study on comprehensive benefit evaluation of green transformation in old residential areas based on AHM-extension evaluation model. Ecological Economy, 37(3), 95–100+160 (in Chinese). [Google Scholar]
16. Yang, B. H., Wu, W. X., Xu, Y. T., Huang, K. (2021). Residential satisfaction of poor space in new city and its influencing factors: A case study of Xi'an enterprise community. Progress in Geography, 40(5), 798–811 (in Chinese). DOI 10.18306/dlkxjz.2021.05.007. [Google Scholar] [CrossRef]
17. Guo, B., Li, Y., Wang, J. X. (2018). The improvement strategy on the management status of the old residence community in Chinese cities: An empirical research based on social cognitive perspective. Cognitive Systems Research, 52, 556–570. DOI 10.1016/j.cogsys.2018.08.002. [Google Scholar] [CrossRef]
18. Huang, W. Z., Hu, L. H., Xing, Y. L. (2022). Sustainable renewal strategies for older communities from the perspective of living experience. Sustainability, 14(5), 2813. DOI 10.3390/su14052813. [Google Scholar] [CrossRef]
19. Cai, X. Q., Kong, L. (2021). Study on influencing factors of project progress of old residential district reconstruction project. Construction Economy, 42(7), 63–66 (in Chinese). [Google Scholar]
20. Zhong, X. F. (2021). Study on cost management difficulties and measures of old residential area reconstruction project. Construction Economy, 42(3), 60–63 (in Chinese). [Google Scholar]
21. Palumbo, M. L., Fimmanò, D., Mangiola, G., Rispoli, V., Annunziato, M. (2017). Strategies for an urban renewal in Rome: Massimina Co_Goal. 7th International Conference on Future Buildings and Districts-Energy Efficiency from Nano to Urban Scale, pp. 559–564. Lausanne, Switzerland. [Google Scholar]
22. Randall, T., Kavalchuk, T., Nelson, R. (2017). Metrics of urban sustainability: A case study of changing downtowns in thunder Bay, Canada. Sustainability, 9(7), 1272. DOI 10.3390/su9071272. [Google Scholar] [CrossRef]
23. Shen, T. Y., Yao, X. Y., Wen, F. H. (2021). The urban regeneration engine model: An analytical framework and case study of the renewal of old communities. Land Use Policy, 108, 105571. DOI 10.1016/j.landusepol.2021.105571. [Google Scholar] [CrossRef]
24. Zhang, L. H., Zhao, Z. L., Kan, Z. N. (2019). Private-sector partner selection for public-private partnership projects of electric vehicle charging infrastructure. Energy Science & Engineering, 7(5), 1469–1484. DOI 10.1002/ese3.367. [Google Scholar] [CrossRef]
25. Ouenniche, J., Boukouras, A., Rajabi, M. (2016). An ordinal game theory approach to the analysis and selection of partners in public-private partnership projects. Journal of Optimization Theory and Applications, 169(1), 314–343. DOI 10.1007/s10957-015-0844-3. [Google Scholar] [CrossRef]
26. Du, J., Wu, H. Y., Zhu, L. (2018). Research on the participation mode selection of construction enterprises in PPP projects. Construction Research Congress 2018: Infrastructure and Facility Management, 2018, 332–342. DOI 10.1061/9780784481295. [Google Scholar] [CrossRef]
27. Li, D. D., Liu, X. W., Qiao, W., Li, P., Yang, J. Y. (2021). Government optimal partner selection for PPP projects: An analysis based on different payment modes. Systems Engineering, 39(1), 50–57 (in Chinese). [Google Scholar]
28. Zhou, T., Zhou, Y. L., Liu, G. W. (2017). Key variables for decision-making on urban renewal in China: A case study of chongqing. Sustainability, 9(3), 370. DOI 10.3390/su9030370. [Google Scholar] [CrossRef]
29. Wang, Y. S., Li, J. F., Zhang, G. L., Li, Y. K., Asare, M. H. (2017). Fuzzy evaluation of comprehensive benefit in urban renewal based on the perspective of core stakeholders. Habitat International, 66, 163–170. DOI 10.1016/j.habitatint.2017.06.003. [Google Scholar] [CrossRef]
30. Ren, R. R., Gao, H. W. (2021). Characteristics and experience of urban renewal investment and financing modes in the United States, Britain and Japan. Macroeconomic Study, 43(8), 168–175 (in Chinese). [Google Scholar]
31. Geng, S., Xu, C. Q., Lin, L. J. (2018). Social capital selection framework of public-private partnership project of electrochemical storage power station under linguistic environment. Journal of Cleaner Production, 199, 751–762. DOI 10.1016/j.jclepro.2018.07.122. [Google Scholar] [CrossRef]
32. He, L. N., Wang, G. T., Liu, J. (2021). Supply chain risk management based on hesitant fuzzy DEMATEL and house of risk. Computer Integrated Manufacturing Systems, 2021, 27(5), 1459–1468 (in Chinese). [Google Scholar]
33. Li, J. S., Xu, K. L. (2021). A combined fuzzy DEMATEL and cloud model approach for risk assessment in process industries to improve system reliability. Quality and Reliability Engineering International, 37(5), 2110–2133. DOI 10.1002/qre.2848. [Google Scholar] [CrossRef]
34. Abdullah, L., Zulkifli, N., Liao, H. C., Herrera-Viedma, E., Al-Barakati, A. (2019). An interval-valued intuitionistic fuzzy DEMATEL method combined with choquet integral for sustainable solid waste management. Engineering Applications of Artificial Intelligence, 82, 207–215. DOI 10.1016/j.engappai.2019.04.005. [Google Scholar] [CrossRef]
35. Tan, R. P., Zhang, W. D. (2020). Multiple attribute decision making method based on DEMATEL and fuzzy distance of trapezoidal fuzzy neutrosophic numbers and its application in typhoon disaster evaluation. Journal of Intelligent & Fuzzy Systems, 39(3), 3413–3439. DOI 10.3233/JIFS-191758. [Google Scholar] [CrossRef]
36. Rostamkhani, R., Abbasi, M. (2021). An integrated fuzzy ANP-fuzzy DEMATEL-fuzzy multi-objective linear programming approach for weapon systems acquisition. International Journal of Industrial Engineering-Theory Applications and Practice, 28(2), 209–227. [Google Scholar]
37. Liu, L., Wu, J., Wei, G. W., Wei, C., Wang, J. et al. (2020). Entropy-based GLDS method for social capital selection of a PPP project with q-rung orthopair fuzzy information. Entropy, 22(4), 414. DOI 10.3390/e22040414. [Google Scholar] [CrossRef]
38. Sun, G. S., Zhang, X. L., Feng, J., Tang, X. R. (2021). Social capital selection of PPP mode for offshore engineering construction based on group decision theory. Mathematics in Practice and Theory, 2021, 51(17), 77–85 (in Chinese). [Google Scholar]
39. Lin, K. P., Tseng, M. L., Pai, P. F. (2018). Sustainable supply chain management using approximate fuzzy DEMATEL method. Resources Conservation and Recycling, 128, 134–142. DOI 10.1016/j.resconrec.2016.11.017. [Google Scholar] [CrossRef]
40. Zhu, S. Y., Li, D. Z., Jiang, Y. (2020). The impacts of relationships between critical barriers on sustainable old residential neighborhood renewal in China. Habitat International, 103, 1–12. DOI 10.1016/j.habitatint.2020.102232. [Google Scholar] [CrossRef]
41. Seker, S., Zavadskas, E. K. (2017). Application of fuzzy DEMATEL method for analyzing occupational risks on construction sites. Sustainability, 9(11), 2083. DOI 10.3390/su9112083. [Google Scholar] [CrossRef]
42. Glaser, B., Strauss, A. L. (1968). The discovery of grounded theory: Strategy for qualitative research. Nursing Research, 17(4), 377–380. DOI 10.1097/00006199-196807000-00014. [Google Scholar] [CrossRef]
43. Thomas, G., James, D. (2005). Reinventing grounded theory: Some questions about theory, ground and discovery. British Educational Research Journal, 32(6), 767–795. DOI 10.1080/01411920600989412. [Google Scholar] [CrossRef]
44. Jia, X. D., Heng, L. (2016). A preliminary study on the construction paradigm of Chinese native management theory based on “Grounded spirit”. Chinese Journal of Management, 13(3), 336–346. (in Chinese). [Google Scholar]
45. Hoare, K. J., Mills, J., Francis, K. (2012). Dancing with data: An example of acquiring theoretical sensitivity in a grounded theory study. International Journal of Nursing Practice, 18(3), 240–245. DOI 10.1111/j.1440-172X.2012.02038.x. [Google Scholar] [CrossRef]
46. Strauss, A. L., Corbin, J. M. (2008). Basics of qualitative research: Techniques and procedures for developing grounded theory. Los Angeles: Sage Publications. [Google Scholar]
47. Zhang, X., Mao, J. Y. (2012). Review of the progress and improvement steps of Chinese enterprise management case studies--Review of Chinese enterprise management case and theory construction research forum (2010). Management World, 28(2), 105–111 (in Chinese). [Google Scholar]
48. CesaBianchi, N., Freund, Y., Helmbold, D. P., Warmuth, M. K. (1996). On-line prediction and conversion strategies. Machine Learning, 25(1), 71–110. DOI 10.1007/BF00115301. [Google Scholar] [CrossRef]
49. Aly, S., Vrana, I. (2008). Evaluating the knowledge, relevance and experience of expert decision makers utilizing the Fuzzy-AHP. Agricultural Economics, 54(11), 529–535. [Google Scholar]
50. Liu, B. S., Shen, Y. H., Chen, Y., Chen, X. H., Wang, Y. M. (2015). A two-layer weight determination method for complex multi-attribute large-group decision-making experts in a linguistic environment. Information Fusion, 23, 156–165. DOI 10.1016/j.inffus.2014.05.001. [Google Scholar] [CrossRef]
51. Wan, S. P., Wang, F., Dong, J. Y. (2016). A novel risk attitudinal ranking method for intuitionistic fuzzy values and application to MADM. Applied Soft Computing, 40, 98–112. DOI 10.1016/j.asoc.2015.11.022. [Google Scholar] [CrossRef]
52. Li, Y. H., Cheng, Y. L., Mou, Q., Xian, S. (2020). Novel cross-entropy based on multi-attribute group decision-making with unknown experts’ weights under interval-valued intuitionistic fuzzy environment. International Journal of Computational Intelligence Systems, 13(1), 1295–1304. DOI 10.2991/ijcis.d.200817.001. [Google Scholar] [CrossRef]
53. Chi, G. T., Qi, F., Zhang, N. (2012). The city’s ecosystem evaluation model based on optimal combination weights and its application. Operations Research and Management Science, 21(2), 183–191 (in Chinese). [Google Scholar]
54. He, Y. H., Guo, H. W., Jin, M. Z., Ren, P. Y. (2016). A linguistic entropy weight method and its application in linguistic multi-attribute group decision making. Nonlinear Dynamics, 84(1), 399–404. DOI 10.1007/s11071-015-2595-y. [Google Scholar] [CrossRef]
55. Yang, H. Q., Wang, L. Q., An, X. X. (2021). Multi-attribute health model of equipment based on subjective and objective integrated weighting. Machine Design and Research, 37(4), 154–158 (in Chinese). [Google Scholar]
56. Addae, B. A., Zhang, L., Zhou, P., Wang, F. D. (2019). Analyzing barriers of smart energy city in Accra with two-step fuzzy DEMATEL. Cities, 89(7), 218–227. DOI 10.1016/j.cities.2019.01.043. [Google Scholar] [CrossRef]
57. Lin, R. J. (2013). Using fuzzy DEMATEL to evaluate the green supply chain management practices. Journal of Cleaner Production, 40, 32–39. DOI 10.1016/j.jclepro.2011.06.010. [Google Scholar] [CrossRef]
58. Opricovic, S., Tzeng, G. H. (2003). Defuzzification within a multicriteria decision model. International Journal of Uncertainty, Fuzziness and Knowledge-Based Systems, 11(5), 635–652. DOI 10.1142/S0218488503002387. [Google Scholar] [CrossRef]
59. Zhou, Q., Huang, W. L., Zhang, Y. (2011). Identifying critical success factors in emergency management using a fuzzy DEMATEL method. Safety Science, 49(2), 243–252. DOI 10.1016/j.ssci.2010.08.005. [Google Scholar] [CrossRef]
60. Wu, B., Zong, L. K., Yan, X., Soares, C. G. (2018). Incorporating evidential reasoning and TOPSIS into group decision-making under uncertainty for handling ship without command. Ocean Engineering, 164, 590–603. DOI 10.1016/j.oceaneng.2018.06.054. [Google Scholar] [CrossRef]
61. Guo, D. M., Li, G. Q., Hu, N. L., Hou, J. (2021). System dynamics analysis of man-machine efficacy in plateau mines. IEEE Access, 9, 18072–18084. DOI 10.1109/Access.6287639. [Google Scholar] [CrossRef]
62. Wang, L., Garg, H. (2021). Algorithm for multiple attribute decision-making with interactive archimedean norm operations under pythagorean fuzzy uncertainty. International Journal of Computational Intelligence Systems, 14(1), 503–527. DOI 10.2991/ijcis.d.201215.002. [Google Scholar] [CrossRef]
Cite This Article
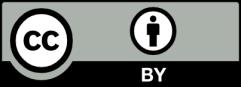
This work is licensed under a Creative Commons Attribution 4.0 International License , which permits unrestricted use, distribution, and reproduction in any medium, provided the original work is properly cited.