Open Access
ARTICLE
Detecting Icing on the Blades of a Wind Turbine Using a Deep Neural Network
1
School of Control and Computer Engineering, North China Electric Power University, Beijing, 102206, China
2
R&D, State Grid Information & Telecommunication Group Co., Beijing, 102211, China
* Corresponding Author: Jiaohui Xu. Email:
(This article belongs to the Special Issue: Hybrid Intelligent Methods for Forecasting in Resources and Energy Field)
Computer Modeling in Engineering & Sciences 2023, 134(2), 767-782. https://doi.org/10.32604/cmes.2022.020702
Received 07 December 2021; Accepted 14 March 2022; Issue published 31 August 2022
Abstract
The blades of wind turbines located at high latitudes are often covered with ice in late autumn and winter, where this affects their capacity for power generation as well as their safety. Accurately identifying the icing of the blades of wind turbines in remote areas is thus important, and a general model is needed to this end. This paper proposes a universal model based on a Deep Neural Network (DNN) that uses data from the Supervisory Control and Data Acquisition (SCADA) system. Two datasets from SCADA are first preprocessed through undersampling, that is, they are labeled, normalized, and balanced. The features of icing of the blades of a turbine identified in previous studies are then used to extract training data from the training dataset. A middle feature is proposed to show how a given feature is correlated with icing on the blade. Performance indicators for the model, including a reward function, are also designed to assess its predictive accuracy. Finally, the most suitable model is used to predict the testing data, and values of the reward function and the predictive accuracy of the model are calculated. The proposed method can be used to relate continuously transferred features with a binary status of icing of the blades of the turbine by using variables of the middle feature. The results here show that an integrated indicator system is superior to a single indicator of accuracy when evaluating the prediction model.Keywords
Cite This Article
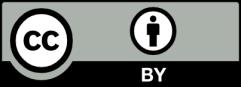
This work is licensed under a Creative Commons Attribution 4.0 International License , which permits unrestricted use, distribution, and reproduction in any medium, provided the original work is properly cited.