Open Access
ARTICLE
A Hybrid BPNN-GARF-SVR Prediction Model Based on EEMD for Ship Motion
School of Instrumentation and Optoelectronic Engineering, Beihang University, Beijing, 100191, China
* Corresponding Author: Wei Wang. Email:
(This article belongs to the Special Issue: Models of Computation: Specification, Implementation and Challenges)
Computer Modeling in Engineering & Sciences 2023, 134(2), 1353-1370. https://doi.org/10.32604/cmes.2022.021494
Received 18 January 2022; Accepted 16 March 2022; Issue published 31 August 2022
Abstract
Accurate prediction of ship motion is very important for ensuring marine safety, weapon control, and aircraft carrier landing, etc. Ship motion is a complex time-varying nonlinear process which is affected by many factors. Time series analysis method and many machine learning methods such as neural networks, support vector machines regression (SVR) have been widely used in ship motion predictions. However, these single models have certain limitations, so this paper adopts a multi-model prediction method. First, ensemble empirical mode decomposition (EEMD) is used to remove noise in ship motion data. Then the random forest (RF) prediction model optimized by genetic algorithm (GA), back propagation neural network (BPNN) prediction model and SVR prediction model are respectively established, and the final prediction results are obtained by results of three models. And the weights coefficients are determined by the correlation coefficients, reducing the risk of prediction and improving the reliability. The experimental results show that the proposed combined model EEMD-GARF-BPNN-SVR is superior to the single predictive model and more reliable. The mean absolute percentage error (MAPE) of the proposed model is 0.84%, but the results of the single models are greater than 1%.Keywords
Cite This Article
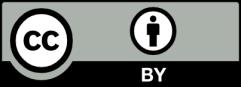