Open Access
ARTICLE
Structural Damage Identification Using Ensemble Deep Convolutional Neural Network Models
1
Department of Civil and Environmental Engineering, Amirkabir University of Technology, Tehran, Iran
2
Department of Urban Planning, Engineering Networks and Systems, Institute of Architecture and Construction, South Ural State
University, Chelyabinsk, Russian
3
Department of Computational Mechanics Laboratory, School of Pedagogical and Technological Education, Athens, Greece
* Corresponding Author: Danial Jahed Armaghani. Email:
(This article belongs to the Special Issue: Soft Computing Techniques in Materials Science and Engineering)
Computer Modeling in Engineering & Sciences 2023, 134(2), 835-855. https://doi.org/10.32604/cmes.2022.020840
Received 15 December 2021; Accepted 29 April 2022; Issue published 31 August 2022
Abstract
The existing strategy for evaluating the damage condition of structures mostly focuses on feedback supplied by traditional visual methods, which may result in an unreliable damage characterization due to inspector subjectivity or insufficient level of expertise. As a result, a robust, reliable, and repeatable method of damage identification is required. Ensemble learning algorithms for identifying structural damage are evaluated in this article, which use deep convolutional neural networks, including simple averaging, integrated stacking, separate stacking, and hybrid weighted averaging ensemble and differential evolution (WAE-DE) ensemble models. Damage identification is carried out on three types of damage. The proposed algorithms are used to analyze the damage of 4585 structural images. The effectiveness of the ensemble learning techniques is evaluated using the confusion matrix. For the testing dataset, the confusion matrix achieved an accuracy of 94 percent and a minimum recall of 92 percent for the best model (WAE-DE) in distinguishing damage types as flexural, shear, combined, or undamaged.Keywords
Cite This Article
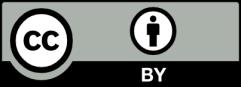
This work is licensed under a Creative Commons Attribution 4.0 International License , which permits unrestricted use, distribution, and reproduction in any medium, provided the original work is properly cited.