Open Access
ARTICLE
Image Representations of Numerical Simulations for Training Neural Networks
1
School of Civil and Transportation Engineering, Hebei University of Technology, Tianjin, 300401, China
2
School of Computer and Software, Nanjing University of Information Science & Technology, Nanjing, 210044, China
* Corresponding Author: Yiming Zhang. Email:
Computer Modeling in Engineering & Sciences 2023, 134(2), 821-833. https://doi.org/10.32604/cmes.2022.022088
Received 20 February 2022; Accepted 15 April 2022; Issue published 31 August 2022
Abstract
A large amount of data can partly assure good fitting quality for the trained neural networks. When the quantity of experimental or on-site monitoring data is commonly insufficient and the quality is difficult to control in engineering practice, numerical simulations can provide a large amount of controlled high quality data. Once the neural networks are trained by such data, they can be used for predicting the properties/responses of the engineering objects instantly, saving the further computing efforts of simulation tools. Correspondingly, a strategy for efficiently transferring the input and output data used and obtained in numerical simulations to neural networks is desirable for engineers and programmers. In this work, we proposed a simple image representation strategy of numerical simulations, where the input and output data are all represented by images. The temporal and spatial information is kept and the data are greatly compressed. In addition, the results are readable for not only computers but also human resources. Some examples are given, indicating the effectiveness of the proposed strategy.Keywords
Cite This Article
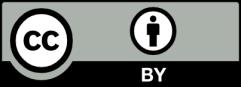
This work is licensed under a Creative Commons Attribution 4.0 International License , which permits unrestricted use, distribution, and reproduction in any medium, provided the original work is properly cited.