Open Access
ARTICLE
Lightweight Network Ensemble Architecture for Environmental Perception on the Autonomous System
1 School of Automation, Beijing Institute of Technology, Beijing, 100081, China
2 Institute of Tobacco Research of CAAS, Qingdao, 266000, China
* Corresponding Authors: Jing Li. Email: ; Songfeng Wang. Email:
Computer Modeling in Engineering & Sciences 2023, 134(1), 135-156. https://doi.org/10.32604/cmes.2022.021525
Received 19 January 2022; Accepted 07 March 2022; Issue published 24 August 2022
Abstract
It is important for the autonomous system to understand environmental information. For the autonomous system, it is desirable to have a strong generalization ability to deal with different complex environmental information, as well as have high accuracy and quick inference speed. Network ensemble architecture is a good choice to improve network performance. However, it is unsuitable for real-time applications on the autonomous system. To tackle this problem, a new neural network ensemble named partial-shared ensemble network (PSENet) is presented. PSENet changes network ensemble architecture from parallel architecture to scatter architecture and merges multiple component networks together to accelerate the inference speed. To make component networks independent of each other, a training method is designed to train the network ensemble architecture. Experiments on Camvid and CIFAR-10 reveal that PSENet achieves quick inference speed while maintaining the ability of ensemble learning. In the real world, PSENet is deployed on the unmanned system and deals with vision tasks such as semantic segmentation and environmental prediction in different fields.Keywords
Cite This Article
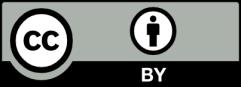
This work is licensed under a Creative Commons Attribution 4.0 International License , which permits unrestricted use, distribution, and reproduction in any medium, provided the original work is properly cited.