Open Access
REVIEW
Machine Learning Techniques for Intrusion Detection Systems in SDN-Recent Advances, Challenges and Future Directions
1
Shaheed Bhagat Singh State University, Firozpur, 152024, India
2
King Khalid University, Abha, 61421, Saudi Arabia
* Corresponding Author: Gulshan Kumar. Email:
Computer Modeling in Engineering & Sciences 2023, 134(1), 89-119. https://doi.org/10.32604/cmes.2022.020724
Received 08 December 2021; Accepted 16 March 2022; Issue published 24 August 2022
Abstract
Software-Defined Networking (SDN) enables flexibility in developing security tools that can effectively and efficiently analyze and detect malicious network traffic for detecting intrusions. Recently Machine Learning (ML) techniques have attracted lots of attention from researchers and industry for developing intrusion detection systems (IDSs) considering logically centralized control and global view of the network provided by SDN. Many IDSs have developed using advances in machine learning and deep learning. This study presents a comprehensive review of recent work of ML-based IDS in context to SDN. It presents a comprehensive study of the existing review papers in the field. It is followed by introducing intrusion detection, ML techniques and their types. Specifically, we present a systematic study of recent works, discuss ongoing research challenges for effective implementation of ML-based intrusion detection in SDN, and promising future works in this field.Keywords
Cite This Article
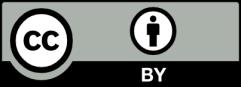
This work is licensed under a Creative Commons Attribution 4.0 International License , which permits unrestricted use, distribution, and reproduction in any medium, provided the original work is properly cited.