Open Access
ARTICLE
A New Childhood Pneumonia Diagnosis Method Based on Fine-Grained Convolutional Neural Network
1 School of Mechanical Engineering and Electronic Information, China University of Geosciences, Wuhan, 430074, China
2 Department of Pediatrics, Tongji Hospital, Tongji Medical College, Huazhong University of Science and Technology, Wuhan, 430074, China
* Corresponding Authors: Long Wen. Email: ; Xiaoping Luo. Email:
(This article belongs to the Special Issue: Computing Methods for Industrial Artificial Intelligence)
Computer Modeling in Engineering & Sciences 2022, 133(3), 873-894. https://doi.org/10.32604/cmes.2022.022322
Received 04 March 2022; Accepted 12 May 2022; Issue published 03 August 2022
Abstract
Pneumonia is part of the main diseases causing the death of children. It is generally diagnosed through chest X-ray images. With the development of Deep Learning (DL), the diagnosis of pneumonia based on DL has received extensive attention. However, due to the small difference between pneumonia and normal images, the performance of DL methods could be improved. This research proposes a new fine-grained Convolutional Neural Network (CNN) for children’s pneumonia diagnosis (FG-CPD). Firstly, the fine-grained CNN classification which can handle the slight difference in images is investigated. To obtain the raw images from the real-world chest X-ray data, the YOLOv4 algorithm is trained to detect and position the chest part in the raw images. Secondly, a novel attention network is proposed, named SGNet, which integrates the spatial information and channel information of the images to locate the discriminative parts in the chest image for expanding the difference between pneumonia and normal images. Thirdly, the automatic data augmentation method is adopted to increase the diversity of the images and avoid the overfitting of FG-CPD. The FG-CPD has been tested on the public Chest X-ray 2017 dataset, and the results show that it has achieved great effect. Then, the FG-CPD is tested on the real chest X-ray images from children aged 3–12 years ago from Tongji Hospital. The results show that FG-CPD has achieved up to 96.91% accuracy, which can validate the potential of the FG-CPD.Keywords
Cite This Article
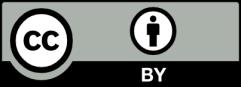