Open Access
ARTICLE
Enhancing the Effectiveness of Trimethylchlorosilane Purification Process Monitoring with Variational Autoencoder
1
Key Laboratory of Advanced Process Control for Light Industry (Ministry of Education), Jiangnan University, Wuxi, 214122, China
2
Hangzhou JASU Environmental Monitoring Co., Ltd., Hangzhou, 311999, China
* Corresponding Author: Shunyi Zhao. Email:
(This article belongs to the Special Issue: Advances on Modeling and State Estimation for Industrial Processes)
Computer Modeling in Engineering & Sciences 2022, 132(2), 531-552. https://doi.org/10.32604/cmes.2022.019521
Received 27 September 2021; Accepted 30 December 2021; Issue published 15 June 2022
Abstract
In modern industry, process monitoring plays a significant role in improving the quality of process conduct. With the higher dimensional of the industrial data, the monitoring methods based on the latent variables have been widely applied in order to decrease the wasting of the industrial database. Nevertheless, these latent variables do not usually follow the Gaussian distribution and thus perform unsuitable when applying some statistics indices, especially the T2 on them. Variational AutoEncoders (VAE), an unsupervised deep learning algorithm using the hierarchy study method, has the ability to make the latent variables follow the Gaussian distribution. The partial least squares (PLS) are used to obtain the information between the dependent variables and independent variables. In this paper, we will integrate these two methods and make a comparison with other methods. The superiority of this proposed method will be verified by the simulation and the Trimethylchlorosilane purification process in terms of the multivariate control charts.Keywords
Cite This Article
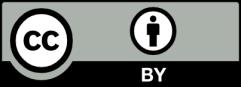
This work is licensed under a Creative Commons Attribution 4.0 International License , which permits unrestricted use, distribution, and reproduction in any medium, provided the original work is properly cited.