Open Access
ARTICLE
State Estimation of Regional Power Systems with Source-Load Two-Terminal Uncertainties
1 School of New Energy and Power Engineering, Lanzhou Jiaotong University, Lanzhou, 730070, China
2 School of Electrical Engineering, Xi’an University of Technology, Xi’an, 710048, China
3 School of Automation and Electrical Engineering, Lanzhou Jiaotong University, Lanzhou, 730070, China
* Corresponding Author: Shuaibing Li. Email:
(This article belongs to the Special Issue: Artificial Intelligence in Renewable Energy and Storage Systems)
Computer Modeling in Engineering & Sciences 2022, 132(1), 295-317. https://doi.org/10.32604/cmes.2022.019996
Received 28 October 2021; Accepted 03 December 2021; Issue published 02 June 2022
Abstract
The development and utilization of large-scale distributed power generation and the increase of impact loads represented by electric locomotives and new energy electric vehicles have brought great challenges to the stable operation of the regional power grid. To improve the prediction accuracy of power systems with source-load two-terminal uncertainties, an adaptive cubature Kalman filter algorithm based on improved initial noise covariance matrix Q0 is proposed in this paper. In the algorithm, the Q0 is used to offset the modeling error, and solves the problem of large voltage amplitude and phase fluctuation of the source-load two-terminal uncertain systems. Verification of the proposed method is implemented on the IEEE 30 node system through simulation. The results show that, compared with the traditional methods, the improved adaptive cubature Kalman filter has higher prediction accuracy, which verifies the effectiveness and accuracy of the proposed method in state estimation of the new energy power system with source-load two-terminal uncertainties.
Keywords
Cite This Article
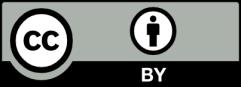