Open Access
ARTICLE
A Method Based on Knowledge Distillation for Fish School Stress State Recognition in Intensive Aquaculture
1 College of Information and Electrical Engineering, China Agricultural University, Beijing, 100083, China
2 National Innovation Center for Digital Fishery, China Agricultural University, Beijing, 100083, China
3 School of Information Science and Technology, Beijing Forestry University, Beijing, 100083, China
* Corresponding Author: Yingyi Chen. Email:
Computer Modeling in Engineering & Sciences 2022, 131(3), 1315-1335. https://doi.org/10.32604/cmes.2022.019378
Received 23 September 2021; Accepted 30 November 2021; Issue published 19 April 2022
Abstract
Fish behavior analysis for recognizing stress is very important for fish welfare and production management in aquaculture. Recent advances have been made in fish behavior analysis based on deep learning. However, most existing methods with top performance rely on considerable memory and computational resources, which is impractical in the real-world scenario. In order to overcome the limitations of these methods, a new method based on knowledge distillation is proposed to identify the stress states of fish schools. The knowledge distillation architecture transfers additional inter-class information via a mixed relative loss function, and it forces a lightweight network (GhostNet) to mimic the soft probabilities output of a well-trained fish stress state recognition network (ResNeXt101). The fish school stress state recognition model's accuracy is improved from 94.17% to 98.12% benefiting from the method. The proposed model has about 5.18 M parameters and requires 0.15 G FLOPs (floating-point operations) to process an image of size 224 × 224. Furthermore, fish behavior images are collected in a land-based factory, and a dataset is constructed and extended through flip, rotation, and color jitter augmentation techniques. The proposed method is also compared with other state-of-the-art methods. The experimental results show that the proposed model is more suitable for deployment on resource-constrained devices or real-time applications, and it is conducive for real-time monitoring of fish behavior.Keywords
Cite This Article
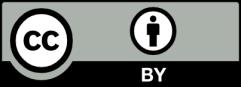
This work is licensed under a Creative Commons Attribution 4.0 International License , which permits unrestricted use, distribution, and reproduction in any medium, provided the original work is properly cited.