Open Access
ARTICLE
A High-Efficiency Inversion Method for the Material Parameters of an Alberich-Type Sound Absorption Coating Based on a Deep Learning Model
1 School of Mechanical Engineering, Guizhou University, Guiyang, 550025, China
2 Aviation Academy, Guizhou Open University, Guiyang, 550023, China
* Corresponding Author: Meng Tao. Email:
Computer Modeling in Engineering & Sciences 2022, 131(3), 1693-1716. https://doi.org/10.32604/cmes.2022.019336
Received 17 September 2021; Accepted 22 November 2021; Issue published 19 April 2022
Abstract
Research on the acoustic performance of an anechoic coating composed of cavities in a viscoelastic material has recently become an area of great interest. Traditional forward research methods are unable to manipulate sound waves accurately and effectively, are difficult to analyse, have time-consuming solution processes, and have large optimization search spaces. To address these issues, this paper proposes a deep learning-based inverse research method to efficiently invert the material parameters of Alberich-type sound absorption coatings and rapidly predict their acoustic performance. First, an autoencoder (AE) model is pretrained to reconstruct the viscoelastic material parameters of an Alberich-type sound absorption coating, the material parameters are extracted into the latent feature space by the encoder, and the decoder model is saved. The internal relationship between the reflection coefficient and latent feature space is trained to establish a multilayer perceptron (MLP). Then, the reflection coefficients in the test set are input to the trained MLP and decoder models to automatically invert the material parameters. The accuracy of the inversion result is 95.08%. Finally, a predictive model is trained to rapidly predict the acoustic performance of the inverted material parameters. The speed of a single test target is 80 times faster than that of the finite element method (FEM). Furthermore, sound absorber material parameters with the best sound absorption performance and a three-band sound absorber are inverted, and their actual sound absorption performance is predicted by the proposed method. The proposed deep learning-based inversion research method provides a solution for low-frequency, wide-band, strong attenuation, and precisely controlled sound waves. It achieves an efficient inversion of material parameters and the rapid forecasting of acoustic performance. The training model can be used for a sound absorbing coating composed of irregular cavities in a viscoelastic material and predict its acoustic performance by only modifying the dataset.Keywords
Cite This Article
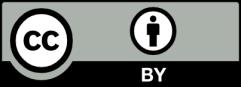
This work is licensed under a Creative Commons Attribution 4.0 International License , which permits unrestricted use, distribution, and reproduction in any medium, provided the original work is properly cited.