Open Access
ARTICLE
Efficient Data Augmentation Techniques for Improved Classification in Limited Data Set of Oral Squamous Cell Carcinoma
1 Department of Information Technology, College of Computers and Information Technology, Taif University, Taif, 21944, Saudi Arabia
2 Institute of Computing, Kohat University of Science and Technology, Kohat, 26000, Pakistan
* Corresponding Author: Wael Alosaimi. Email:
Computer Modeling in Engineering & Sciences 2022, 131(3), 1387-1401. https://doi.org/10.32604/cmes.2022.018433
Received 24 July 2021; Accepted 30 November 2021; Issue published 19 April 2022
Abstract
Deep Learning (DL) techniques as a subfield of data science are getting overwhelming attention mainly because of their ability to understand the underlying pattern of data in making classifications. These techniques require a considerable amount of data to efficiently train the DL models. Generally, when the data size is larger, the DL models perform better. However, it is not possible to have a considerable amount of data in different domains such as healthcare. In healthcare, it is impossible to have a substantial amount of data to solve medical problems using Artificial Intelligence, mainly due to ethical issues and the privacy of patients. To solve this problem of small dataset, different techniques of data augmentation are used that can increase the size of the training set. However, these techniques only change the shape of the image and hence the classification model does not increase accuracy. Generative Adversarial Networks (GANs) are very powerful techniques to augment training data as new samples are created. This technique helps the classification models to increase their accuracy. In this paper, we have investigated augmentation techniques in healthcare image classification. The objective of this research paper is to develop a novel augmentation technique that can increase the size of the training set, to enable deep learning techniques to achieve higher accuracy. We have compared the performance of the image classifiers using the standard augmentation technique and GANs. Our results demonstrate that GANs increase the training data, and eventually, the classifier achieves an accuracy of 90% compared to standard data augmentation techniques, which achieve an accuracy of up to 70%. Other advanced CNN models are also tested and have demonstrated that more deep architectures can achieve more than 98% accuracy for making classification on Oral Squamous Cell Carcinoma.Keywords
Cite This Article
Alosaimi, W., Uddin, M. I. (2022). Efficient Data Augmentation Techniques for Improved Classification in Limited Data Set of Oral Squamous Cell Carcinoma. CMES-Computer Modeling in Engineering & Sciences, 131(3), 1387–1401. https://doi.org/10.32604/cmes.2022.018433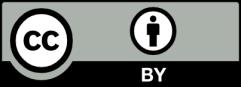