Open Access
ARTICLE
Prototypical Network Based on Manhattan Distance
1
College of Computer Science and Technology, Qingdao University, Qingdao, China
2
Psychiatric Department, Qingdao Municipal Hospital, Qingdao, China
3
Qingdao Institute of Bioenergy and Bioprocess Technology, Chinese Academy of Sciences, Qingdao, China
* Corresponding Author: Ke Wang. Email:
Computer Modeling in Engineering & Sciences 2022, 131(2), 655-675. https://doi.org/10.32604/cmes.2022.019612
Received 01 October 2021; Accepted 09 November 2021; Issue published 14 March 2022
Abstract
Few-shot Learning algorithms can be effectively applied to fields where certain categories have only a small amount of data or a small amount of labeled data, such as medical images, terrorist surveillance, and so on. The Metric Learning in the Few-shot Learning algorithm is classified by measuring the similarity between the classified samples and the unclassified samples. This paper improves the Prototypical Network in the Metric Learning, and changes its core metric function to Manhattan distance. The Convolutional Neural Network of the embedded module is changed, and mechanisms such as average pooling and Dropout are added. Through comparative experiments, it is found that this model can converge in a small number of iterations (below 15,000 episodes), and its performance exceeds algorithms such as MAML. Research shows that replacing Manhattan distance with Euclidean distance can effectively improve the classification effect of the Prototypical Network, and mechanisms such as average pooling and Dropout can also effectively improve the model.Keywords
Cite This Article
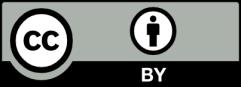