Open Access
ARTICLE
Skew t Distribution-Based Nonlinear Filter with Asymmetric Measurement Noise Using Variational Bayesian Inference
1 Key Laboratory of Advanced Process Control for Light Industry (Ministry of Education), Jiangnan University, Wuxi, 214122, China
2 School of Science, Jiangnan University, Wuxi, 214122, China
3 Department of Chemical and Materials Engineering, University of Alberta, Edmonton, AB T6G 2G6, Canada
* Corresponding Author: Hongtian Chen. Email:
(This article belongs to the Special Issue: Advances on Modeling and State Estimation for Industrial Processes)
Computer Modeling in Engineering & Sciences 2022, 131(1), 349-364. https://doi.org/10.32604/cmes.2021.019027
Received 30 August 2021; Accepted 20 October 2021; Issue published 24 January 2022
Abstract
This paper is focused on the state estimation problem for nonlinear systems with unknown statistics of measurement noise. Based on the cubature Kalman filter, we propose a new nonlinear filtering algorithm that employs a skew t distribution to characterize the asymmetry of the measurement noise. The system states and the statistics of skew t noise distribution, including the shape matrix, the scale matrix, and the degree of freedom (DOF) are estimated jointly by employing variational Bayesian (VB) inference. The proposed method is validated in a target tracking example. Results of the simulation indicate that the proposed nonlinear filter can perform satisfactorily in the presence of unknown statistics of measurement noise and outperform than the existing state-of-the-art nonlinear filters.Keywords
Cite This Article
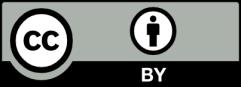
This work is licensed under a Creative Commons Attribution 4.0 International License , which permits unrestricted use, distribution, and reproduction in any medium, provided the original work is properly cited.