Open Access
ARTICLE
Mu-Net: Multi-Path Upsampling Convolution Network for Medical Image Segmentation
1 School of Information and Software Engineering, University of Electronic Science and Technology of China, Chengdu,
610054, China
2 Department of Economics and Finance/Sustainable Real Estate Research Center, Hong Kong Shue Yan University, Hong Kong, China
3 Rattanakosin International College of Creative Entrepreneurship, Rajamangala University of Technology Rattanakosin, Nakhon
Pathom, 73170, Thailand
4 Department of Computer Science and Engineering, School of Sciences, European University Cyprus, Nicosia, 1516, Cyprus
5 CIICESI, ESTG, Polit´ecnico do Porto, 4610-156, Felgueiras, Portugal
* Corresponding Author: Bei Hui. Email:
Computer Modeling in Engineering & Sciences 2022, 131(1), 73-95. https://doi.org/10.32604/cmes.2022.018565
Received 02 August 2021; Accepted 21 October 2021; Issue published 24 January 2022
Abstract
Medical image segmentation plays an important role in clinical diagnosis, quantitative analysis, and treatment process. Since 2015, U-Net-based approaches have been widely used for medical image segmentation. The purpose of the U-Net expansive path is to map low-resolution encoder feature maps to full input resolution feature maps. However, the consecutive deconvolution and convolutional operations in the expansive path lead to the loss of some high-level information. More high-level information can make the segmentation more accurate. In this paper, we propose MU-Net, a novel, multi-path upsampling convolution network to retain more high-level information. The MU-Net mainly consists of three parts: contracting path, skip connection, and multi-expansive paths. The proposed MU-Net architecture is evaluated based on three different medical imaging datasets. Our experiments show that MU-Net improves the segmentation performance of U-Net-based methods on different datasets. At the same time, the computational efficiency is significantly improved by reducing the number of parameters by more than half.Keywords
Cite This Article
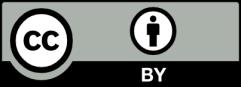
This work is licensed under a Creative Commons Attribution 4.0 International License , which permits unrestricted use, distribution, and reproduction in any medium, provided the original work is properly cited.