Open Access
ARTICLE
N-SVRG: Stochastic Variance Reduction Gradient with Noise Reduction Ability for Small Batch Samples
School of Information Science and Engineering, Fudan University, Shanghai, 200433, China
* Corresponding Author: Lirong Zheng. Email:
(This article belongs to the Special Issue: Enabled and Human-centric Computational Intelligence Solutions for Visual Understanding and Application)
Computer Modeling in Engineering & Sciences 2022, 131(1), 493-512. https://doi.org/10.32604/cmes.2022.019069
Received 01 September 2021; Accepted 11 October 2021; Issue published 24 January 2022
Abstract
The machine learning model converges slowly and has unstable training since large variance by random using a sample estimate gradient in SGD. To this end, we propose a noise reduction method for Stochastic Variance Reduction gradient (SVRG), called N-SVRG, which uses small batches samples instead of all samples for the average gradient calculation, while performing an incremental update of the average gradient. In each round of iteration, a small batch of samples is randomly selected for the average gradient calculation, while the average gradient is updated by rounding of the past model gradients during internal iterations. By suitably reducing the batch size B, the memory storage as well as the number of iterations can be reduced. The experiments are compared with the state-of-the-art Mini-Batch SGD, AdaGrad, RMSProp, SVRG and SCSG, and it is demonstrated that N-SVRG outperforms SVRG and SASG, and is on par with SCSG. Finally, by exploring the relationship between the small values of different parameters n. B and k and the effectiveness of the algorithm, we prove that our N-SVRG algorithm has some stability and can achieve sufficient accuracy even in the case of small batch size. The advantages and disadvantages of various methods are experimentally compared, and the stability of N-SVRG is explored by parameter settings.Keywords
Cite This Article
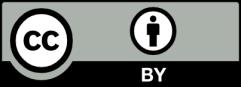
This work is licensed under a Creative Commons Attribution 4.0 International License , which permits unrestricted use, distribution, and reproduction in any medium, provided the original work is properly cited.