Open Access
ARTICLE
Machine Learning Enhanced Boundary Element Method: Prediction of Gaussian Quadrature Points
1 College of Architecture and Civil Engineering, Xinyang Normal University, Xinyang, 464000, China
2 College of Intelligent Construction, Wuchang University of Technology, Wuhan, 430223, China
3 School of Architectural Engineering, Huanghuai University, Zhumadian, 463000, China
* Corresponding Author: Leilei Chen. Email:
(This article belongs to the Special Issue: Machine Learning-Guided Intelligent Modeling with Its Industrial Applications)
Computer Modeling in Engineering & Sciences 2022, 131(1), 445-464. https://doi.org/10.32604/cmes.2022.018519
Received 30 July 2021; Accepted 20 October 2021; Issue published 24 January 2022
Abstract
This paper applies a machine learning technique to find a general and efficient numerical integration scheme for boundary element methods. A model based on the neural network multi-classification algorithm is constructed to find the minimum number of Gaussian quadrature points satisfying the given accuracy. The constructed model is trained by using a large amount of data calculated in the traditional boundary element method and the optimal network architecture is selected. The two-dimensional potential problem of a circular structure is tested and analyzed based on the determined model, and the accuracy of the model is about 90%. Finally, by incorporating the predicted Gaussian quadrature points into the boundary element analysis, we find that the numerical solution and the analytical solution are in good agreement, which verifies the robustness of the proposed method.Keywords
Cite This Article
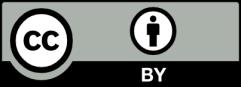
This work is licensed under a Creative Commons Attribution 4.0 International License , which permits unrestricted use, distribution, and reproduction in any medium, provided the original work is properly cited.