Open Access
ARTICLE
Remote Sensing Image Retrieval Based on 3D-Local Ternary Pattern (LTP) Features and Non-subsampled Shearlet Transform (NSST) Domain Statistical Features
Department of Electronics and Communication Engineering, Tezpur University, Assam, 784028, India
* Corresponding Author: Hilly Gohain Baruah. Email:
Computer Modeling in Engineering & Sciences 2022, 131(1), 137-164. https://doi.org/10.32604/cmes.2022.018339
Received 17 July 2021; Accepted 22 October 2021; Issue published 24 January 2022
Abstract
With the increasing popularity of high-resolution remote sensing images, the remote sensing image retrieval (RSIR) has always been a topic of major issue. A combined, global non-subsampled shearlet transform (NSST)-domain statistical features (NSSTds) and local three dimensional local ternary pattern (3D-LTP) features, is proposed for high-resolution remote sensing images. We model the NSST image coefficients of detail subbands using 2-state laplacian mixture (LM) distribution and its three parameters are estimated using Expectation-Maximization (EM) algorithm. We also calculate the statistical parameters such as subband kurtosis and skewness from detail subbands along with mean and standard deviation calculated from approximation subband, and concatenate all of them with the 2-state LM parameters to describe the global features of the image. The various properties of NSST such as multiscale, localization and flexible directional sensitivity make it a suitable choice to provide an effective approximation of an image. In order to extract the dense local features, a new 3D-LTP is proposed where dimension reduction is performed via selection of ‘uniform’ patterns. The 3D-LTP is calculated from spatial RGB planes of the input image. The proposed inter-channel 3D-LTP not only exploits the local texture information but the color information is captured too. Finally, a fused feature representation (NSSTds-3DLTP) is proposed using new global (NSSTds) and local (3D-LTP) features to enhance the discriminativeness of features. The retrieval performance of proposed NSSTds-3DLTP features are tested on three challenging remote sensing image datasets such as WHU-RS19, Aerial Image Dataset (AID) and PatternNet in terms of mean average precision (MAP), average normalized modified retrieval rank (ANMRR) and precision-recall (P-R) graph. The experimental results are encouraging and the NSSTds-3DLTP features leads to superior retrieval performance compared to many well known existing descriptors such as Gabor RGB, Granulometry, local binary pattern (LBP), Fisher vector (FV), vector of locally aggregated descriptors (VLAD) and median robust extended local binary pattern (MRELBP). For WHU-RS19 dataset, in terms of {MAP,ANMRR}, the NSSTds-3DLTP improves upon Gabor RGB, Granulometry, LBP, FV, VLAD and MRELBP descriptors by {41.93%,20.87%}, {92.30%,32.68%}, {86.14%,31.97%}, {18.18%,15.22%}, {8.96%,19.60%} and {15.60%,13.26%}, respectively. For AID, in terms of {MAP,ANMRR}, the NSSTds-3DLTP improves upon Gabor RGB, Granulometry, LBP, FV, VLAD and MRELBP descriptors by {152.60%,22.06%}, {226.65%,25.08%}, {185.03%,23.33%}, {80.06%,12.16%}, {50.58%,10.49%} and {62.34%,3.24%}, respectively. For PatternNet, the NSSTds-3DLTP respectively improves upon Gabor RGB, Granulometry, LBP, FV, VLAD and MRELBP descriptors by {32.79%, 10.34%}, {141.30%, 24.72%}, {17.47%,10.34%}, {83.20%,19.07%}, {21.56%,3.60%}, and {19.30%,0.48%} in terms of {MAP,ANMRR}. The moderate dimensionality of simple NSSTds-3DLTP allows the system to run in real-time.Keywords
Cite This Article
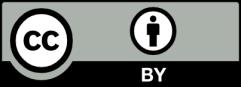
This work is licensed under a Creative Commons Attribution 4.0 International License , which permits unrestricted use, distribution, and reproduction in any medium, provided the original work is properly cited.