Open Access
ARTICLE
Strengthened Initialization of Adaptive Cross-Generation Differential Evolution
1 Department of Computer Science of Technology, Ocean University of China, Qingdao, 266100, China
2 Key Laboratory of Symbolic Computation and Knowledge Engineering of Ministry of Education, Jilin University, Changchun,
130012, China
3 Guangxi Key Laboratory of Hybrid Computation and IC Design Analysis, Guangxi University for Nationalities, Nanning,
530006, China
* Corresponding Author:Gaige Wang. Email:
(This article belongs to the Special Issue: Swarm Intelligence and Applications in Combinatorial Optimization)
Computer Modeling in Engineering & Sciences 2022, 130(3), 1495-1516. https://doi.org/10.32604/cmes.2021.017987
Received 21 June 2021; Accepted 26 September 2021; Issue published 30 December 2021
Abstract
Adaptive Cross-Generation Differential Evolution (ACGDE) is a recently-introduced algorithm for solving multiobjective problems with remarkable performance compared to other evolutionary algorithms (EAs). However, its convergence and diversity are not satisfactory compared with the latest algorithms. In order to adapt to the current environment, ACGDE requires improvements in many aspects, such as its initialization and mutant operator. In this paper, an enhanced version is proposed, namely SIACGDE. It incorporates a strengthened initialization strategy and optimized parameters in contrast to its predecessor. These improvements make the direction of crossgeneration mutation more clearly and the ability of searching more efficiently. The experiments show that the new algorithm has better diversity and improves convergence to a certain extent. At the same time, SIACGDE outperforms other state-of-the-art algorithms on four metrics of 24 test problems.Keywords
Cite This Article
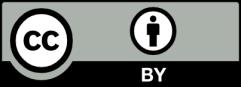
This work is licensed under a Creative Commons Attribution 4.0 International License , which permits unrestricted use, distribution, and reproduction in any medium, provided the original work is properly cited.