Open Access
ARTICLE
Image Reconstruction for ECT under Compressed Sensing Framework Based on an Overcomplete Dictionary
1 School of Electrical and Control Engineering, Xi’an University of Science and Technology, Xi’an, 710054, China
2 Shaanxi Coal and Shaanbei Mining Co., Ltd., Xi’an, 719000, China
* Corresponding Author: Xuebin Qin. Email:
(This article belongs to the Special Issue: Mechanical Reliability of Advanced Materials and Structures for Harsh Applications)
Computer Modeling in Engineering & Sciences 2022, 130(3), 1699-1717. https://doi.org/10.32604/cmes.2022.018234
Received 09 July 2021; Accepted 02 September 2021; Issue published 30 December 2021
Abstract
Electrical capacitance tomography (ECT) has great application potential in multiphase process monitoring, and its visualization results are of great significance for studying the changes in two-phase flow in closed environments. In this paper, compressed sensing (CS) theory based on dictionary learning is introduced to the inverse problem of ECT, and the K-SVD algorithm is used to learn the overcomplete dictionary to establish a nonlinear mapping between observed capacitance and sparse space. Because the trained overcomplete dictionary has the property to match few features of interest in the reconstructed image of ECT, it is not necessary to rely on the sparsity of coefficient vector to solve the nonlinear mapping as most algorithms based on CS theory. Two-phase flow distribution in a cylindrical pipe was modeled and simulated, and three variations without sparse constraint based on Landweber, Tikhonov, and Newton-Raphson algorithms were used to rapidly reconstruct a 2-D image.Keywords
Cite This Article
Qin, X., Shen, Y., Hu, J., Li, M., Yang, P. et al. (2022). Image Reconstruction for ECT under Compressed Sensing Framework Based on an Overcomplete Dictionary. CMES-Computer Modeling in Engineering & Sciences, 130(3), 1699–1717. https://doi.org/10.32604/cmes.2022.018234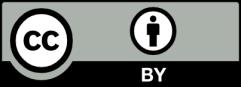