Open Access
ARTICLE
Pattern-Moving-Based Parameter Identification of Output Error Models with Multi-Threshold Quantized Observations
1 School of Automation and Electrical Engineering, University of Science and Technology Beijing; Key Laboratory of Knowledge Automation for Industrial Processes, Ministry of Education, Beijing, 100083, China
2 School of Information Engineering, Jingdezhen University, Jingdezhen, 333000, China
* Corresponding Author: Zhengguang Xu. Email:
(This article belongs to the Special Issue: Advances on Modeling and State Estimation for Industrial Processes)
Computer Modeling in Engineering & Sciences 2022, 130(3), 1807-1825. https://doi.org/10.32604/cmes.2022.017799
Received 08 June 2021; Accepted 03 September 2021; Issue published 30 December 2021
Abstract
This paper addresses a modified auxiliary model stochastic gradient recursive parameter identification algorithm (M-AM-SGRPIA) for a class of single input single output (SISO) linear output error models with multi-threshold quantized observations. It proves the convergence of the designed algorithm. A pattern-moving-based system dynamics description method with hybrid metrics is proposed for a kind of practical single input multiple output (SIMO) or SISO nonlinear systems, and a SISO linear output error model with multi-threshold quantized observations is adopted to approximate the unknown system. The system input design is accomplished using the measurement technology of random repeatability test, and the probabilistic characteristic of the explicit metric value is employed to estimate the implicit metric value of the pattern class variable. A modified auxiliary model stochastic gradient recursive algorithm (M-AM-SGRA) is designed to identify the model parameters, and the contraction mapping principle proves its convergence. Two numerical examples are given to demonstrate the feasibility and effectiveness of the achieved identification algorithm.Keywords
Cite This Article
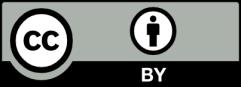