Open Access
ARTICLE
Action Recognition Based on CSI Signal Using Improved Deep Residual Network Model
1 School of Information Science and Technology, Northwest University, Xi’an, 710127, China
2 School of Mathematics, Northwest University, Xi’an, 710127, China
* Corresponding Author:Jian Jia. Email:
(This article belongs to the Special Issue: Modeling and Analysis of Autonomous Intelligence)
Computer Modeling in Engineering & Sciences 2022, 130(3), 1827-1851. https://doi.org/10.32604/cmes.2022.017654
Received 28 May 2021; Accepted 09 July 2021; Issue published 30 December 2021
Abstract
In this paper, we propose an improved deep residual network model to recognize human actions. Action data is composed of channel state information signals, which are continuous fine-grained signals. We replaced the traditional identity connection with the shrinking threshold module. The module automatically adjusts the threshold of the action data signal, and filters out signals that are not related to the principal components. We use the attention mechanism to improve the memory of the network model to the action signal, so as to better recognize the action. To verify the validity of the experiment more accurately, we collected action data in two different environments. The experimental results show that the improved network model is much better than the traditional network in recognition. The accuracy of recognition in complex places can reach 92.85%, among which the recognition rate of raising hands is up to 96%. We combine the improved residual deep network model with channel state information action data, and prove the effectiveness of our model for classification through experimental data.Keywords
Cite This Article
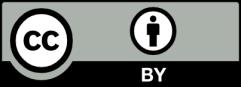
This work is licensed under a Creative Commons Attribution 4.0 International License , which permits unrestricted use, distribution, and reproduction in any medium, provided the original work is properly cited.