Open Access
ARTICLE
Transferable Features from 1D-Convolutional Network for Industrial Malware Classification
1 School of Computer and Communication Engineering, University of Science and Technology Beijing, Beijing, 100083, China
2 Beijing Key Laboratory of Knowledge Engineering for Materials Science, Beijing, 100083, China
3 Shunde Graduate School, University of Science and Technology Beijing, Foshan, 528399, China
4 Beijing Intelligent Logistics System Collaborative Innovation Center, Beijing, 101149, China
* Corresponding Author: Xiong Luo. Email:
(This article belongs to the Special Issue: Machine Learning-Guided Intelligent Modeling with Its Industrial Applications)
Computer Modeling in Engineering & Sciences 2022, 130(2), 1003-1016. https://doi.org/10.32604/cmes.2022.018492
Received 28 July 2021; Accepted 28 September 2021; Issue published 13 December 2021
Abstract
With the development of information technology, malware threats to the industrial system have become an emergent issue, since various industrial infrastructures have been deeply integrated into our modern works and lives. To identify and classify new malware variants, different types of deep learning models have been widely explored recently. Generally, sufficient data is usually required to achieve a well-trained deep learning classifier with satisfactory generalization ability. However, in current practical applications, an ample supply of data is absent in most specific industrial malware detection scenarios. Transfer learning as an effective approach can be used to alleviate the influence of the small sample size problem. In addition, it can also reuse the knowledge from pre-trained models, which is beneficial to the real-time requirement in industrial malware detection. In this paper, we investigate the transferable features learned by a 1D-convolutional network and evaluate our proposed methods on 6 transfer learning tasks. The experiment results show that 1D-convolutional architecture is effective to learn transferable features for malware classification, and indicate that transferring the first 2 layers of our proposed 1D-convolutional network is the most efficient way to reuse the learned features.Keywords
Cite This Article
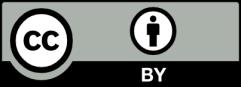