Open Access
ARTICLE
Evaluating the Clogging Behavior of Pervious Concrete (PC) Using the Machine Learning Techniques
1 School of Mines, China University of Mining and Technology, Xuzhou, 221116, China
2 School of Chemical Engineering and Technology, China University of Mining and Technology, Xuzhou, 221116, China
* Corresponding Author: Yuan Gao. Email:
(This article belongs to the Special Issue: Soft Computing Techniques in Materials Science and Engineering)
Computer Modeling in Engineering & Sciences 2022, 130(2), 805-821. https://doi.org/10.32604/cmes.2022.017792
Received 07 June 2021; Accepted 09 August 2021; Issue published 13 December 2021
Abstract
Pervious concrete (PC) is at risk of clogging due to the continuous blockage of sand into it during its service time. This study aims to evaluate and predict such clogging behavior of PC using hybrid machine learning techniques. Based on the 84 groups of the dataset developed in the earlier study, the clogging behavior of the PC was determined by the algorithm combing the SVM (support vector machines) and particle swarm optimization (PSO) methods. The PSO algorithm was employed to adjust the hyperparameters of the SVM and verify the performance using 10-fold cross-validation. The predicting results of the developed model were assessed by the coefficient of determination (R) and root mean square error (RMSE). The importance of the influential variables on the clogging behavior of PC was evaluated as well. The results showed that the PSO algorithm can effectively adjust the hyperparameters of the SVM model and can be used to construct the predictive model for the clogging behavior of the PC. The combined algorithm has the advantage of higher reliability and validity than the random hyperparameters selection. For the verification process, the developed model was able to obtain values of 0.9469 and 1.8148 for the R and RMSE, showing that the developed machine learning model can accurately be used to evaluate and predict the clogging behavior of the PC, guiding the mix-design of PC from the perspective of durability. The size of the clogging sand is the most important parameter and the thickness of the sample is the least significant factor affecting the clogging behavior. The proportions of the smallest aggregate size and largest aggregate size are the two most important design parameters of concrete with the consideration of the relatively higher importance scores, showing these two aggregates should be given special attention in future PC design for anti-clogging purposes.
Keywords
Cite This Article
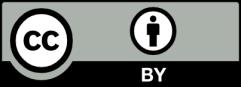
This work is licensed under a Creative Commons Attribution 4.0 International License , which permits unrestricted use, distribution, and reproduction in any medium, provided the original work is properly cited.