Open Access
ARTICLE
Thermogram Adaptive Efficient Model for Breast Cancer Detection Using Fractional Derivative Mask and Hybrid Feature Set in the IoT Environment
1 Rajasthan Technical University, Kota, Rajasthan, 324010, India
2 Anand International College of Engineering, Jaipur, 303012, India
3 Nonlinear Dynamics Research Center (NDRC), Ajman University, Ajman, 20550, United Arab Emirates
4 International Center for Basic and Applied Sciences, Jaipur, 302029, India
5 Institute of Mathematical Modeling, Almaty, 050000, Kazakhstan
* Corresponding Author: Praveen Agarwal. Email:
(This article belongs to the Special Issue: Advanced Mathematical Tool-Based Internet of Things (IoT))
Computer Modeling in Engineering & Sciences 2022, 130(2), 923-947. https://doi.org/10.32604/cmes.2022.016065
Received 03 February 2021; Accepted 23 August 2021; Issue published 13 December 2021
Abstract
In this paper, a novel hybrid texture feature set and fractional derivative filter-based breast cancer detection model is introduced. This paper also introduces the application of a histogram of linear bipolar pattern features (HLBP) for breast thermogram classification. Initially, breast tissues are separated by masking operation and filtered by Grmwald–Letnikov fractional derivative-based Sobel mask to enhance the texture and rectify the noise. A novel hybrid feature set using HLBP and other statistical feature sets is derived and reduced by principal component analysis. Radial basis function kernel-based support vector machine is employed for detecting the abnormality in the thermogram. The performance parameters are calculated using five-fold cross-validation scheme using MATLAB 2015a simulation software. The proposed model achieves the classification accuracy, sensitivity, specificity, and area under the curve of 94.44%, 95.55%, 92.22%, 96.11%, respectively. A comparative investigation of different texture features with respect to fractional order to classify the breast malignancy is also presented. The proposed model is also compared with a few existing state-of-art schemes which verifies the efficacy of the model. Fractional order offers extra adaptability in overcoming the limitations of thermal imaging techniques and assists radiologists in prior breast cancer detection. The proposed model is more generalized which can be used with different thermal image acquisition protocols and IoT based applications.Keywords
Cite This Article
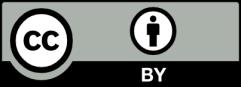