Open Access
ARTICLE
A Fast Small-Sample Modeling Method for Precision Inertial Systems Fault Prediction and Quantitative Anomaly Measurement
1 Xi'an Research Institute of High-Tech, Xi'an, 710025, China
2 Northwest University of Politics and Law, Xi'an, 710122, China
* Corresponding Author: Hongqiao Wang. Email:
(This article belongs to the Special Issue: Machine Learning-Guided Intelligent Modeling with Its Industrial Applications)
Computer Modeling in Engineering & Sciences 2022, 130(1), 187-203. https://doi.org/10.32604/cmes.2022.018000
Received 21 June 2021; Accepted 29 July 2021; Issue published 29 November 2021
Abstract
Inertial system platforms are a kind of important precision devices, which have the characteristics of difficult acquisition for state data and small sample scale. Focusing on the model optimization for data-driven fault state prediction and quantitative degree measurement, a fast small-sample supersphere one-class SVM modeling method using support vectors pre-selection is systematically studied in this paper. By theorem-proving the irrelevance between the model's learning result and the non-support vectors (NSVs), the distribution characters of the support vectors are analyzed. On this basis, a modeling method with selected samples having specific geometry character from the training sets is also proposed. The method can remarkably eliminate the NSVs and improve the algorithm's efficiency. The experimental results testify that the scale of training samples and the modeling time consumption both give a sharply decrease using the support vectors pre-selection method. The experimental results on inertial devices also show good fault prediction capability and effectiveness of quantitative anomaly measurement.Keywords
Cite This Article
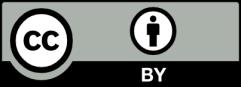
This work is licensed under a Creative Commons Attribution 4.0 International License , which permits unrestricted use, distribution, and reproduction in any medium, provided the original work is properly cited.