Open Access
ARTICLE
Reduced Order Machine Learning Finite Element Methods: Concept, Implementation, and Future Applications
1 Department of Mechanical Engineering, Northwestern University, Evanston, 60208, USA
2 Theoretical and Applied Mechanics, Northwestern University, Evanston, 60208, USA
3 Department of Mechanical Engineering, The University of Texas at Dallas, Richardson, 75080, USA
* Corresponding Author:Wing Kam Liu. Email:
(This article belongs to the Special Issue: Advances in Computational Mechanics and Optimization
To celebrate the 95th birthday of Professor Karl Stark Pister)
Computer Modeling in Engineering & Sciences 2021, 129(3), 1351-1371. https://doi.org/10.32604/cmes.2021.017719
Received 01 June 2021; Accepted 08 July 2021; Issue published 25 November 2021
Abstract
This paper presents the concept of reduced order machine learning finite element (FE) method. In particular, we propose an example of such method, the proper generalized decomposition (PGD) reduced hierarchical deeplearning neural networks (HiDeNN), called HiDeNN-PGD. We described first the HiDeNN interface seamlessly with the current commercial and open source FE codes. The proposed reduced order method can reduce significantly the degrees of freedom for machine learning and physics based modeling and is able to deal with high dimensional problems. This method is found more accurate than conventional finite element methods with a small portion of degrees of freedom. Different potential applications of the method, including topology optimization, multi-scale and multi-physics material modeling, and additive manufacturing, will be discussed in the paper.Keywords
Cite This Article
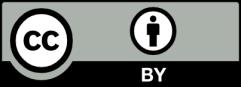
This work is licensed under a Creative Commons Attribution 4.0 International License , which permits unrestricted use, distribution, and reproduction in any medium, provided the original work is properly cited.