Open Access
ARTICLE
BEVGGC: Biogeography-Based Optimization Expert-VGG for Diagnosis COVID-19 via Chest X-ray Images
1 College of Computer Science and Technology, Henan Polytechnic University, Jiaozuo, 454000, China
2 Nursing Department, The Fourth People’s Hospital of Huai’an, Huai’an, 223001, China
3 Provincial Key Laboratory for Computer Information Processing Technology, Soochow University, Suzhou, 215000, China
* Corresponding Authors: Chaosheng Tang. Email: ; Shixin Chen. Email:
# Junding Sun & Xiang Li contributed equally to this paper, and should be considered as co-first authors
(This article belongs to the Special Issue: Computer-Assisted Imaging Processing and Machine Learning Applications on Diagnosis of Chest Radiograph)
Computer Modeling in Engineering & Sciences 2021, 129(2), 729-753. https://doi.org/10.32604/cmes.2021.016416
Received 04 March 2021; Accepted 29 June 2021; Issue published 08 October 2021
Abstract
Purpose: As to January 11, 2021, coronavirus disease (COVID-19) has caused more than 2 million deaths worldwide. Mainly diagnostic methods of COVID-19 are: (i) nucleic acid testing. This method requires high requirements on the sample testing environment. When collecting samples, staff are in a susceptible environment, which increases the risk of infection. (ii) chest computed tomography. The cost of it is high and some radiation in the scan process. (iii) chest X-ray images. It has the advantages of fast imaging, higher spatial recognition than chest computed tomography. Therefore, our team chose the chest X-ray images as the experimental dataset in this paper. Methods: We proposed a novel framework—BEVGG and three methods (BEVGGC-I, BEVGGC-II, and BEVGGC-III) to diagnose COVID-19 via chest X-ray images. Besides, we used biogeography-based optimization to optimize the values of hyperparameters of the convolutional neural network. Results: The experimental results show that the OA of our proposed three methods are 97.65% ± 0.65%, 94.49% ± 0.22% and 94.81% ± 0.52%. BEVGGC-I has the best performance of all methods. Conclusions: The OA of BEVGGC-I is 9.59% ± 1.04% higher than that of state-of-the-art methods.Keywords
Cite This Article
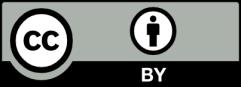
This work is licensed under a Creative Commons Attribution 4.0 International License , which permits unrestricted use, distribution, and reproduction in any medium, provided the original work is properly cited.