Open Access
ARTICLE
A Novel Named Entity Recognition Scheme for Steel E-Commerce Platforms Using a Lite BERT
1 School of Computer and Communication Engineering, University of Science and Technology Beijing, Beijing, 100083, China
2 Beijing Key Laboratory of Knowledge Engineering for Materials Science, Beijing, 100083, China
3 Shunde Graduate School, University of Science and Technology Beijing, Foshan, 528399, China
4 Ouyeel Co., Ltd., Shanghai, 201999, China
* Corresponding Author: Xiong Luo. Email:
(This article belongs to the Special Issue: Innovation and Application of Intelligent Processing of Data, Information and Knowledge in E-Commerce)
Computer Modeling in Engineering & Sciences 2021, 129(1), 47-63. https://doi.org/10.32604/cmes.2021.017491
Received 13 May 2021; Accepted 12 June 2021; Issue published 24 August 2021
Abstract
In the era of big data, E-commerce plays an increasingly important role, and steel E-commerce certainly occupies a positive position. However, it is very difficult to choose satisfactory steel raw materials from diverse steel commodities online on steel E-commerce platforms in the purchase of staffs. In order to improve the efficiency of purchasers searching for commodities on the steel E-commerce platforms, we propose a novel deep learning-based loss function for named entity recognition (NER). Considering the impacts of small sample and imbalanced data, in our NER scheme, the focal loss, the label smoothing, and the cross entropy are incorporated into a lite bidirectional encoder representations from transformers (BERT) model to avoid the over-fitting. Moreover, through the analysis of different classic annotation techniques used to tag data, an ideal one is chosen for the training model in our proposed scheme. Experiments are conducted on Chinese steel E-commerce datasets. The experimental results show that the training time of a lite BERT (ALBERT)-based method is much shorter than that of BERT-based models, while achieving the similar computational performance in terms of metrics precision, recall, and F1 with BERT-based models. Meanwhile, our proposed approach performs much better than that of combining Word2Vec, bidirectional long short-term memory (Bi-LSTM), and conditional random field (CRF) models, in consideration of training time and F1.Keywords
Cite This Article
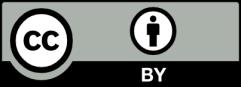
This work is licensed under a Creative Commons Attribution 4.0 International License , which permits unrestricted use, distribution, and reproduction in any medium, provided the original work is properly cited.